Oct 31, 2023 | Volume 13 - Issue 3
Dalena Dillman Taylor, Saundra M. Tabet, Megan A. Whitbeck, Ryan G. Carlson, Sejal Barden, Nicole Silverio
Individuals living in poverty have higher rates of mental health disorders compared to those not living in poverty. Measures are available to assess adults’ levels of psychological distress; however, there is limited support for instruments to be used with a diverse population. The purpose of our study was to examine the factor structure of Outcome Questionnaire-45.2 scores with an economically vulnerable sample of adults (N = 615), contributing to the evidence of validity of the measure’s scores in diverse mental health settings. Implications for professional counselors are considered, including clinical usage of the brief Outcome Questionnaire-16 and key critical items.
Keywords: poverty, psychological distress, factor structure, Outcome Questionnaire-45.2, validity
In the United States, it is estimated that 34 million adults live in poverty (i.e., income less than $12,880 per year), and poverty is a significant factor contributing to poor mental and physical health outcomes (Hodgkinson et al., 2017). Poverty, or economic vulnerability, refers to the extent to which individuals have difficulty living with their current level of income, increasing the risk for adverse social and economic consequences (Semega et al., 2021). Economically vulnerable adults often experience greater social inequality, lower educational attainment, less economic mobility (Stanford Center on Poverty and Inequality, 2015), and difficulty securing full-time employment (Dakin & Wampler, 2008), which leads to increased distress (Lam et al., 2019). Lower income levels are also associated with several mental health conditions (e.g., anxiety, depression, suicide attempts; Santiago et al., 2011). Further, Lam and colleagues (2019) found strong negative associations between income, socioeconomic status, and psychological distress.
To effectively support their clients, counselors must understand the unique context and financial stressors related to living in poverty. Incorporating poverty-sensitive measures into assessment and evaluation practices is essential to providing culturally responsive care that considers the systemic and environmental barriers of poverty (Clark et al., 2020). Implementing culturally responsive assessments ensures that counselors use outcome measures that are attuned to poverty-related experiences (Clark et al., 2020). Such measures can help counselors identify and prioritize treatment planning approaches and acknowledge the reality that economic disadvantages create for clients (Foss-Kelly et al., 2017). However, the availability of poverty-sensitive assessments is limited.
Measuring Psychological Distress in Adults Living in Poverty
Because of the risk of mental health issues related to economic vulnerability, assessments with evidence of validity and reliability that measure psychological distress relative to income are warranted. Professional counselors can individualize their therapeutic approach to meet the needs of this population with the assistance of accurate assessments of related mental health conditions. Naher and colleagues (2020) noted the need for individual-level data as well as interventions specifically targeted to adults living in poverty. Although outcome assessments exist to measure psychological distress or severity of mental illness symptoms (e.g., Beck Depression Inventory [BDI], Beck et al., 1961; Generalized Anxiety Disorder Screener [GAD-7], Löwe et al., 2008; Patient Health Questionnaire-9 [PHQ-9], Kroenke et al., 2001), there is a lack of measures with evidence of validity and reliability with economically vulnerable adult populations. Therefore, our investigation examined the factor structure of the Outcome Questionnaire-45.2 (OQ-45.2; Lambert et al., 2004) with an economically vulnerable adult population, increasing the applicability of the measure in mental health settings.
Outcome Questionnaire-45.2
The OQ-45.2 (Lambert et al., 2004) is one of the most widely used outcome measures of psychological distress in applied mental health settings (Hatfield & Ogles, 2004). The OQ-45.2 assists professional counselors with monitoring client progress and can be administered multiple times throughout treatment, as it is sensitive to changes over time (Lambert et al., 1996). The OQ-45.2 has been implemented in outcome-based research with diverse populations such as university counseling center clients (Tabet et al., 2019), low-income couples (Carlson et al., 2017), and ethnic minority groups (Lambert et al., 2006). Lambert et al. (1996) reported strong test-retest reliability (r = .84) and internal consistency (α = .93) for the OQ-45.2, based on a sample of undergraduate students (n = 157) and a sample of individuals receiving Employee Assistance Program services (n = 289). However, researchers have yet to investigate the psychometric properties of the OQ-45.2 with an economically disadvantaged, diverse population.
Given the utility of the OQ-45.2 as a client-reported feedback measure, clinicians can use the OQ-45.2 in a variety of ways to evaluate client progress, including measuring changes in individual distress across the course of counseling and before and after specific treatment interventions, as well as to glean a baseline level of distress at the start of counseling (Lambert, 2017). For example, one study used the OQ-45.2 as a primary outcome measure for anxiety symptoms in clients engaging in cognitive behavioral therapy (Levy et al., 2020). The OQ-45.2 was administered at the beginning of each weekly counseling session and change scores were calculated between each session, which helped clinicians understand that about half of their sample reported clinically significant reductions in symptoms in just nine sessions (Levy et al., 2020). This example demonstrates how the OQ-45.2 can be implemented to monitor treatment outcomes and improve the duration and efficiency of counseling. A clinician can also use salient items as part of the intake clinical interview to encourage clients to elaborate on the specific symptoms they are experiencing, and how they may be impacting their functioning, across a variety of clinical settings (Espiridion et al., 2021; Lambert, 2017; Levy et al., 2020).
Factor Structure of OQ-45.2
Researchers contested the factor structure proposed by Lambert et al. (2004), suggesting the need for further validation of the three-factor oblique measurement model and exploration of other possible factor structures (e.g., Kim et al., 2010; Mueller et al., 1998; Rice et al., 2014; Tabet et al., 2019). Mueller and colleagues (1998) examined three models: (a) a one-factor model, (b) a two-factor oblique model, and (c) a three-factor oblique model, none of which fit the data well. In addition, the factors in the three-factor model were highly correlated, ranging from .83 to .91, asserting that the subscales may not be statistically indistinguishable and the OQ-45.2 might be a unidimensional measure of global distress.
Kim and colleagues (2010) also explored three models to assess adequate fit of the data: (a) a one-factor model, (b) a three-factor model, and (c) a revised 22-item four-factor model. Indicating weak support for the OQ-45.2’s factorial validity across all models, researchers cautioned against widespread utilization in mental health and research settings, encouraging further psychometric exploration and validation of the OQ-45.2 (Kim et al., 2010).
Rice and colleagues (2014) found evidence to support a two-factor OQ-45.2 model that included (a) overall maladjustment and (b) substance use. Results indicated relatively good fit (comparative fit index [CFI] = .990, root-mean-square error of approximation [RMSEA] = .068) for a two-factor measure with 11 items, which demonstrated better model fit than the original three-factor model
(CFI = .840, RMSEA = .086 [90% confidence interval {CI} = .085, .087]). Overall, multiple researchers have demonstrated poor fit for the original factor structure of the OQ-45.2 (Kim et al., 2010; Mueller et al., 1998; Rice et al., 2014; Tabet et al., 2019), supporting the need for further validation for using the OQ-45.2 with samples of adults living in poverty.
This study’s primary aim is to examine the factor structure of the OQ-45.2 with an economically vulnerable sample to enhance the generalizability of the OQ-45.2 in mental health settings. Therefore, the following research questions guided our study:
RQ1. What is the factor structure of OQ-45.2 scores with a sample of adults living in poverty?
RQ2. What is the internal consistency reliability of the abbreviated 16-item OQ-45.2 scores with a sample of adults living in poverty?
RQ3. What is the test-retest reliability of the abbreviated 16-item OQ-45.2 scores with a sample of adults living in poverty?
Method
Participants and Procedures
Participants comprised a sub-sample from a grant-funded, community-based, relationship education program for individuals and couples at a university in the Southeastern United States. The project was funded through the U.S. Department of Health and Human Services, Administration for Children and Families, Office of Family Assistance (Grant #90FM0078). Study recruitment strategically involved passive and active recruitment strategies (Carlson et al., 2014) from various community locations that primarily serve low-income individuals and families (e.g., libraries, employment offices). Participants met inclusion criteria if they were at least 18 years old and interested in learning about healthy relationships. The relationship education intervention utilized was an evidence-based curriculum that taught individuals tools to improve their relationships in a small group setting (Prevention and Relationship Education Program [PREP]; Pearson et al., 2015).
We obtained ethical approval from the university’s IRB prior to data collection. Each person participated in a group intake session that consisted of a review of the informed consent; a battery of assessments, including the OQ-45.2; and a brief activity. Study participants (N = 615) included in this current analysis consented between July 2015 and June 2019.
Demographic Information
We collected demographic data as part of this study, which included gender, age, ethnicity, income, educational level, working status, and marital status (see Table 1). The majority of participants fell below the poverty line when factoring in number of children and/or under- or unemployment. Therefore, our sample consisted of a diverse population, including variations in income, age, ethnicity, and race.
Table 1
Participant Demographic Characteristics
Descriptive Characteristic Total Sample (n, %)
Age
18–20 years
21–24 years
25–34 years
35–44 years
45–54 years
55–64 years
65 years or older |
34 (5.5)
52 (8.5)
130 (21.1)
139 (22.6)
137 (22.3)
91 (14.8)
32 (5.2) |
Gender (female) |
498 (81.0) |
Race |
|
American Indian or Alaska Native |
18 (2.9) |
Asian |
19 (3.1) |
Black or African American |
176 (28.6) |
Native American or Pacific Islander |
2 (0.3) |
White |
248 (40.3) |
Other |
144 (23.4) |
Ethnicity
Hispanic or Latino
Not Hispanic or Latino
Income |
258 (42.0)
356 (57.9) |
Less than $500 |
216 (35.1) |
$501–$1,000 |
108 (17.6) |
$1,001–$2,000
$2,001–3,000
$3,001–$4,000
$4,001–$5,000
More than $5,000 |
124 (20.2)
81 (13.2)
28 (4.6)
18 (2.9)
18 (2.9) |
Educational Level
No degree or diploma earned
High school diploma
Some college but no degree completion
Associate degree
Bachelor’s degree
Master’s / advanced degree |
24 (3.9)
18 (2.9)
75 (12.2)
66 (10.7)
134 (21.8)
77 (12.5) |
Marital Status
Married
Engaged
Divorced
Widowed
Never Married |
93 (15.1)
11 (1.8)
164 (26.7)
24 (3.9)
270 (43.9) |
Employment Status
Full-time employment
Part-time employment
Temporary, occasional, or seasonal, or odd jobs for pay
Not currently employed
Employed, but number of hours change from week to week
Selected multiple responses
Number of Children
0
1
2
3
4
5
6 |
227 (36.9)
83 (13.5)
41 (6.7)
207 (33.7)
29 (13.5)
6 (1.0)
148 (24.1)
60 (9.8)
44 (7.2)
17 (2.8)
6 (1.0)
4 (0.7)
1 (0.4) |
Instrument
The Outcome Questionnaire-45.2
The OQ-45.2 is a self-report questionnaire that captures individuals’ subjective functionality in various aspects of life that can lead to common mental health concerns (e.g., anxiety, depression, substance use). The current three-factor structure of the OQ-45.2 has 45 items rated on a 5-point Likert scale, with rankings of 0 (never), 1 (rarely), 2 (sometimes), 3 (frequently), and 4 (almost always; Lambert et al., 2004). Nine OQ-45.2 items are reverse scored, with total OQ-45.2 scores calculated by summing all 45 items with a range from 0 to 180. Clinically significant changes are represented in a change score of at least 14, whether positive or negative (i.e., increased or reduced distress).
The Symptom Distress subscale (25 items) evaluates anxiety, depression, and substance abuse symptoms, as these are the most diagnosed mental health concerns (Lambert et al., 1996). The Interpersonal Relations subscale (11 items) includes items that measure difficulties and satisfaction in relationships. The Social Role Performance subscale (nine items) assesses conflict, distress, and inadequacy related to employment, family roles, and leisure activities. The OQ-45.2 also includes four critical items (Items 8, 11, 32, and 44) targeting suicidal ideation, homicidal ideation, and substance use. The Cronbach’s alpha for the OQ-45.2 in the current study was calculated at .943.
Data Analysis
We calculated descriptive statistics on the total sample population, including the mean, standard deviations, and frequencies. Subsequently, we conducted preliminary descriptive analyses to test for statistical assumptions that included missing data, collinearity issues, and multivariate normality (Byrne, 2016). In the first analysis, we used confirmatory factor analysis (CFA) to test the factor structure of the OQ-45.2 with this population (N = 615) and subsequently used exploratory factor analysis (EFA) to evaluate revised OQ models.
We conducted CFA utilizing the original three-factor oblique model (Lambert et al., 2004) as the a priori model to test the hypothesized structure of the latent variables. In addition, based on the results of the study, we tested a series of alternative structural models outlined by Bludworth and colleagues (2010). Given the non-normal distribution, we utilized MPlus (Version 8.4) with a robust maximum likelihood (MLR) parameter estimation (Satorra & Bentler, 1994). To address missing data, we employed a full information maximum likelihood (FIML) to approximate the population parameters and produce the estimates from the sample data (Enders, 2010). Results of the CFA were evaluated using several fit indices: (a) the chi-square test of model fit (χ2; nonsignificance at p > .05 indicate a good fit [Hu & Bentler, 1999]); (b) the CFI (values larger than .95 indicate a good fit [Bentler, 1990]); (c) TLI (values larger than .95 indicate a good fit [Tucker & Lewis, 1973]); (d) RMSEA with 90% CI (values between .05 and .08 indicate a good fit [Browne & Cudeck, 1993]); and (e) standardized root-mean-square residual (SRMR; values below .08 indicate good fit [Hu & Bentler, 1999]).
Following the CFA, we conducted EFA because of poor model fit across all models and several items with outer loadings of less than 0.5 (Tabachnick & Fidell, 2019). Kline (2016) recommended researchers should not be constrained by the original factor structure when CFA indicates low outer loadings and should consider conducting an EFA because the data may not fit the original number of factors suggested. Accordingly, we conducted an EFA to test the number of factors derived from the 45-item OQ-45.2 within our population. We exceeded the recommended ratio (i.e., 10:1) of participants to the number of items (12.6:1; Costello & Osborne, 2005; Hair et al., 2010; Mvududu & Sink, 2013). We conducted a principal axis factoring with Promax rotation to determine whether factors were correlated using SPSS version 25.0. We chose parallel analysis (Horn, 1965) using the 95th percentile to determine the number of factors to retain given that previous researchers have acknowledged parallel analysis to be a superior method to extract significant factors as compared to conventional statistical indices such as Cattell’s scree test (Henson & Roberts, 2006). We used stringent criterion when identifying loading and cross-loading items such as items that indicated high (i.e., equal to or exceeding 1.00) or low communality values (i.e., less than 0.40; Costello & Osborne, 2005) and items with substantive cross-loadings (< .30 between two factor loadings; Tabachnick & Fidell, 2019) were removed. To ensure the most parsimonious model, we removed items individually from Factor 1, which has the greatest number of items, to reduce the size of the model while still capturing the greatest variance explained by the items on that factor.
Results
We screened the data and checked for statistical assumptions prior to conducting factor analysis. Little’s Missing Completely at Random (MCAR) test (Little, 1988), a multivariate extension of a simple t-test, evaluated the mean differences of the 45 items to determine the pattern and missingness of data (Enders, 2010). Given the significant chi-square, data were not missing completely at random
(χ2 = 912.062, df = 769, p < .001). However, results indicated a very small percentage of values (< 1%) were missing from each variable; therefore, supporting data were missing at random (MAR; Osborne, 2013). When data are MAR, an FIML approach to replace missing values provides unbiased parameter estimates and improves the statistical power of analyses (Enders, 2010). The initial internal consistency reliability estimates (coefficient alpha) for scores on the original OQ-45.2 model were all in acceptable ranges except for Factor 3 (see Henson & Roberts, 2006): total α = .943, Symptom Distress α = .932
(k = 25 items), Interpersonal Relations α = .802 (k = 11 items), and Social Role Performance α = .683
(k = 9 items). We also conducted Bartlett’s test of sphericity (p < .001) and the Kaiser-Meyer-Olkin value (.950), indicating the data was suitable for conducting a factor analysis. We evaluated multivariate normality of the dataset with Mardia’s multivariate kurtosis coefficient. Mardia’s coefficient of multivariate kurtosis was .458; therefore, we deemed the data to be non-normally distributed
(Hu & Bentler, 1995).
Confirmatory Factor Analysis
We tested the developer’s original OQ-45.2 three-factor oblique model, and because of the results subsequently tested a series of alternative structural models outlined by Bludworth and colleagues (2010). Specifically, the alternative structural models tested included: (a) a three-factor orthogonal model, (b) a one-factor model, (c) a four-factor hierarchical model, and (d) a four-factor bilevel model. Table 2 presents the fit indices results in the series of CFAs. The original three-factor oblique model allowed all three factors (Social Role Performance, Interpersonal Relations, and Symptom Distress) to correlate, but resulted in a poor fit: χ2 (942, N = 615) = 3.014, p < .001; CFI = .779; TLI = .768; RMSEA = .057, 90% CI [.055, .060]; SRMR = .063. We next uncorrelated the factors and tested a three-factor orthogonal model, which also presented a poor fit with worsened fit metrics: χ2 (945, N = 615) = 3.825, p < .001; CFI = .689; TLI = .674; RMSEA = .068, 90% CI [.065, .070]; SRMR = .202. Accordingly, because the factors demonstrated high intercorrelation (rs = .94, .93, .91) in the three-factor oblique model and lack of factorial validity based on the CFA results of both three-factor models, we suspected the OQ-45.2 to be a unidimensional, one-factor model. However, the CFA revealed a poor fit to the OQ-45.2 one-factor model: χ2 (945, N = 615) = 3.197,
p < .001; CFI = .758; TLI = .747; RMSEA = .060, 90% CI [.057, .062]; SRMR = .062.
Table 2
Goodness-of-Fit Indices for the Item-Level Models of the OQ-45.2
|
χ2 |
df |
p |
χ2/df |
CFI |
TLI |
RMSEA |
90% CI |
SRMR |
One-Factor |
3021.300 |
945 |
.000 |
3.197 |
.758 |
.747 |
.060 |
[.057, .062] |
.062 |
Three-Factor (orthogonal) |
3615.060 |
945 |
.000 |
3.825 |
.689 |
.674 |
.068 |
[.065, .070] |
.202 |
Three-Factor (oblique) |
2839.335 |
942 |
.000 |
3.014 |
.779 |
.768 |
.057 |
[.055, .060] |
.063 |
Four-Factor (hierarchical) |
2839.335 |
942 |
.000 |
3.014 |
.779 |
.768 |
.057 |
[.055, .060] |
.063 |
Four-Factor
(bilevel) |
2363.263 |
900 |
.000 |
2.626 |
.829 |
.812 |
.051 |
[.049, .054] |
.054 |
Note. N = 615. χ2 = chi-square; df = degrees of freedom; χ2/df = relative chi-square; CFI = comparative fit index;
TLI = Tucker-Lewis Index; RMSEA = root-mean-square error of approximation; 90% CI = 90% confidence interval;
SRMR = standardized root-mean-square residual.
We proceeded to test the OQ-45.2 as a four-factor hierarchical model. In this multidimensional model, the three first-order factors (Social Role Performance, Interpersonal Relations, and Symptom Distress) became a linear combination to sum a second-order general factor (g-factor) of Psychological Distress (Eid et al., 2017). Results evidenced an unacceptable overall fit to the data: χ2 (942, N = 615) = 3.014, p < .001; CFI = .779; TLI = .768; RMSEA = .057, 90% CI [.055, .060]; SRMR = .063. Last, we examined a four-factor bilevel model. In this model, the g-factor of Psychological Distress has a direct effect on items, whereas, in the hierarchal model, it had an indirect effect on items. Therefore, the items in the four-factor bilevel model load onto both their intended factors (Social Role Performance, Interpersonal Relations, and Symptom Distress) and the g-factor (Psychological Distress). Nevertheless, although the four-factor bilevel was cumulatively the best fitting OQ-45.2 factorial model, the results still yielded a poor fit:
χ2 (900, N = 615) = 2.626, p < .001; CFI = .829; TLI = .812; RMSEA = .051, 90% CI [.049, .054]; SRMR = .054.
Overall, all models demonstrated a significant chi-square (p < .001); however, this result is common in larger sample sizes (N > 400; Kline, 2016). Because the chi-square statistic is sensitive to sample size and model complexity, researchers have recommended using other fit indices (e.g., RMSEA, CFI) to determine overall model fit (Tabachnick & Fidell, 2019). Nevertheless, the levels of the CFI values (ranging from .689 to .829) and TLI values (ranging from .674 to .812) were low, and far below the recommended referential cutoff (> .90; Tucker & Lewis, 1973). Although the models’ RMSEA values were within the recommended range of .05 to .08 (Browne & Cudeck, 1993), and the majority of SRMR values were below .08 (Hu & Bentler, 1999), these were the only fit indices that met acceptable cutoffs. We further examined outer loadings for the 45 items within the factorial models and identified that all models had outer loadings (ranging from 5 to 14 items) below the 0.5 cutoff (Tabachnick & Fidell, 2019). When CFA produces low factor loadings and poor fit indices, researchers should not be constrained to the original specified number of factors and should consider conducting an EFA (Kline, 2016). Hence, we elected to conduct an EFA to explore the factor structure with this population.
Exploratory Factor Analysis
Results from the initial EFA using principal axis factoring with the 45 OQ items produced a solution that explained 55.564% of the total variance. After multiple iterations of item deletions, we concluded with a three-factor solution. We present the internal reliability estimates of two three-factor solutions: (a) a 16-item three-factor solution—the most parsimonious—and (b) an 18-item three-factor solution, including all critical items in Table 3. We present the first three-factor solution because it was derived using stringent criteria for creating the most parsimonious solution (Costello & Osborne, 2005; Henson & Roberts, 2006; Tabachnick & Fidell, 2019), whereas the second three-factor solution included conceptual judgment determining the inclusion of the critical items from the original OQ-45.2.
Table 3
Internal Consistency Estimates
|
Total |
Symptom Distress |
Interpersonal Relations |
Social Role Performance |
Original OQ-45 |
.943 |
.932 |
.802 |
.683 |
|
Total |
Factor 1 |
Factor 2 |
Factor 3 |
16-Item Model |
.894 |
.864 |
.840 |
.710 |
18-Item Model |
.896 |
.857 |
.840 |
.700 |
Three-Factor Solution
Results from the parallel analysis (Horn, 1965) indicated an initial four-factor solution. Through multiple iterations (n = 9) of examining factor loadings, removing items one at a time, and reexamining parallel analysis after each deletion, our results demonstrated that a three-factor solution was the most parsimonious. We removed a total of 29 items because of low communalities (< .5), low factor loadings (< .4), and substantive cross-loadings (> .3 between two factor loadings; Tabachnick & Fidell, 2019). Before accepting the removal of these items, we added each back to the model to determine its impact on the overall model. No items improved the model; therefore, we accepted the deletion of the 29 items. The final three-factor solution included 16 items with 57.99% of total variance explained, which indicates near acceptable variance in social science research, with 60% being acceptable (Hair et al., 2010). Factor 1 (seven items) explained 38.98% of the total variance; Factor 2 (six items) explained 11.37% of the total variance; and Factor 3 (three items) explained 7.64% of the total variance.
Three-Factor Solution With Critical Items
After finalizing the model, we added Item 8 (“I have thoughts of ending my life”) and Item 44 (“I feel angry enough at work/school to do something I might regret”) into the final model for purposes of clinical utility. Both items resulted in low factor loading (< .4). Item 8 correlated with other items on Factor 3, and Item 44 correlated with other items on Factor 1. This final 18-item three-factor solution reduced the variance explained by the items on the factors by 3.45%, indicating a questionable fit for social sciences (54.54%; Hair et al., 2010). Factor 1 (eight items) explained 36.83% of the total variance; Factor 2 (six items) explained 10.82% of the total variance; and Factor 3 (four items) explained 6.90% of the total variance. Internal consistency estimates are presented in Table 3 for all three models: (a) the original OQ-45.2 (α = .943); (b) the 16-item, three-factor solution (α = .894); and (c) the 18-item, three-factor solution (α = .896).
Test-Retest Reliability
To examine the stability of the new 16-item OQ scores over time, we assessed test-retest reliability over a 30-day interval using bivariate correlation (Pallant, 2016). Results yielded strong correlation coefficients between pre-OQ scores and post-OQ scores: (a) OQ Total Scores, r = .781, p < .001; (b) Factor 1, r = .782, p < .001; (c) Factor 2, r = .742, p < .001; and (d) Factor 3, r = .681, p < .001. The 18-item OQ scores also demonstrated significant support for test-retest reliability over a 30-day interval: (a) OQ Total Scores, r = .721, p < .001; (b) Factor 1, r = .658, p < .001; (c) Factor 2, r = .712, p < .001; and (d) Factor 3, r = .682, p < .001.
Discussion
We found that the current factor structure of the OQ-45.2 poorly fits the sample population of economically vulnerable individuals. Our preliminary results support Rice and colleagues’ (2014) claim: because of the unique stressors economically vulnerable individuals face, the OQ-45.2 does not adequately capture their psychological distress. The lack of support for the OQ-45.2’s current structure (i.e., three-factor oblique) creates doubt clinically when assessing clients’ distress. Therefore, we explored alternative structural models proposed by Bludworth and colleagues (2010) using a CFA, and subsequently an EFA, to reexamine the factor structure of the OQ-45.2.
The EFA resulted in a 16-item, three-factor solution with our sample, indicating marginal support for the validity and reliability of the items for this brief model of the OQ, meaning that this model lacked reliability (i.e., ability to produce similar results consistently) and validity (i.e., ability to actually measure what it intends to measure: distress). In social science research, total variance explained of 60% is adequate (Hair et al., 2010); therefore, the three-factor model that approaches 60% could be acceptable, indicating that this model captures more than half or more than chance of the construct distress for this population. Still, additional research is needed to support the factor structure with a similar population of low-income, diverse individuals. Economically vulnerable individuals experience unique stressors (Karney & Bradbury, 2005), and brief assessments are best practices (Beidas et al., 2015). Therefore, we encourage other researchers to reexamine the use of this brief version of the OQ with a sample of economically vulnerable individuals or develop a new instrument that may more accurately capture psychological distress in economically disadvantaged individuals.
Also, the 16-item model results differ from the original OQ-45.2 in that we were unable to find support for the social role factor with our sample population. We hypothesize this finding is largely due to the economic stressors this population faces (e.g., unreliable transportation, food scarcity, housing needs). Anecdotally, some participants commented during the initial intake session that several items (e.g., specifically items on the social role factor relating to employment) were not relevant to their situation because of under- or unemployment. Further, reducing OQ-45.2 to a 16-item assessment may provide a more user-friendly version requiring less time for respondents and more efficient use of clinical time; however, without further research, the current authors are hesitant to support its clinical use with this population of economically vulnerable individuals.
Similar to previous researchers (e.g., Kim et al., 2010; Rice et al., 2014), we also found evidence for the need for a substance use factor (e.g., Factor 3) in the 18-item abbreviated model; however, this model deviated from the original OQ-45.2. The findings of this study support the need for professional counselors to assess substance use as part of psychological distress, whether it be implementing the
18-item version of the OQ or adding an additional assessment that has greater reliability and validity of its items with this population.
Implications
We found initial, possible support for a brief version of the OQ-45.2 for economically vulnerable individuals. The abbreviated 16-item OQ assessment derived from this research requires less time to complete while capturing an individual’s distress on substance use, interpersonal relationships, and symptom distress. A brief instrument can provide professional counselors with a snapshot of the client’s concerns, which can assist in monitoring a client’s level of psychological distress throughout treatment. In clinical settings, counselors can utilize this instrument to briefly assess at intake the baseline distress of their clients and use it as a guide or conversation starter for discussing client distress. For example, a counselor may ask that the client complete the brief OQ-16 instrument with the intake paperwork. In review of all paperwork, the counselor may note to the client, “I noticed that you indicated high distress with interpersonal relationships. Is that a place you would like to begin, or do you have another place you want to begin?”
Further, we retained two critical items (i.e., Items 8 and 44) in the 18-item version of the OQ brief assessment, as psychological distress associated with economic vulnerability is linked to higher rates of suicide and homicide (Knifton & Inglis, 2020). Because of the clinical utility of this instrument, professional counselors may want to include those items to assess a client’s level of threat of harm to self or others. Dependent on the client’s answer to these critical items, professional counselors have a quick reference with which to intervene or focus the initial session to address safety. Therefore, the items of this assessment may possibly be used to start the initial dialogue regarding an individual’s psychological distress and/or suicidal intent; however, the assessment should not be used as the only tool or instrument to diagnose or treat psychological distress. We understand that these items can help professional counselors efficiently assess for suicidal or homicidal intent. Therefore, the counselor can opt to use the 16-item version and include an additional, more reliable assessment for measuring threat of harm to self and/or others. For example, counselors may opt to use an instrument such as the Ask Suicide-Screening Questions tool (Horowitz et al., 2012) to further evaluate suicidal intent.
In our experience, when following up with study participants based on a score higher than 1 on a scale of 1–5, many participants indicated they felt that way in the past but no longer feel that way now. In our use of the OQ-45.2, we find that participants tend to answer these questions based on their entire life versus the time frame indicated in the assessment instructions (the past week [7 days]). Therefore, professional counselors should be clear that respondents should answer based on the past week, rather than “ever experienced.” When offering the assessment to clients, we recommend that the counselor highlight the time frame in the instructions or clearly communicate that time frame to the client before they complete the instrument to gain the most accurate data.
Limitations and Suggestions for Future Research
As with all research, results should be considered in light of limitations. The large study sample consisted of diverse individuals; however, the majority were women, and all individuals were from the southeast region of the United States, minimizing the generalizability of these findings. In addition, although findings indicate initial, possible support for a revised three-factor model consisting of 16 items, future studies are warranted to strengthen the validity of this abbreviated version of the OQ-45.2. We suggest that future researchers test the 16-item assessment through CFA with a similar population to confirm the current study’s findings. All respondents volunteered to participate in a 6-month study, which may indicate more motivation to improve or represent a population with distress responses different from those who were recruited but chose not to participate in the study. Additionally, study participants were actively recruited, and may have experienced less distress than a help-seeking sample.
The OQ is available in a Spanish translation; however, we only included people who completed the English OQ-45.2 version in the current study. Future analyses should examine the factor structure of the Spanish OQ-45.2 as well. Next, future research on the OQ should include the development and testing of new items. Lastly, future research should aim to validate the reduced 16-item and 18-item OQ scores on a new sample and seek to establish a new criterion for clinical significance. Professional counselors may also benefit from the creation of a specific instrument assessing distress related to the unique stressors that economically vulnerable clients face. Until further analyses are conducted with a new sample population to confirm the abbreviated models, we encourage professional counselors to implement the brief version tentatively and with caution, and to follow up with the client regarding high scores on critical items prior to making clinical judgments regarding reported subscale scores.
Conclusion
Given the broad utility of the OQ-45.2 in research and mental health settings, researchers and professional counselors must understand the instrument’s structure for interpretation purposes and how the assessment should be adapted for various populations. Professional counselors can effectively support clients by assessing and recognizing how economic-related distress impacts their quality of life, which may directly relate to treatment outcomes. Findings from the current study add to previous literature that calls into question the original OQ-45.2 factor structure. Additionally, the current study’s findings support a revised 16-item, three-factor structure for economically vulnerable clients and we provide implications for use of this assessment in clinical practice. Future research should include a confirmatory analysis of the current findings.
Conflict of Interest and Funding Disclosure
This research was supported by a grant (90FM0078)
from the U.S. Department of Health and Human
Services (USDHHS), Administration for Children and
Families, Office of Family Assistance. Any opinions,
findings, conclusions, or recommendations are those
of the authors and do not necessarily reflect the views
of the USDHHS, Office of Family Assistance. The authors
reported no further funding or conflict of interest.
References
Beck, A. T., Ward, C. H., Mendelson, M., Mock, J., & Erbaugh, J. (1961). An inventory for measuring depression. Archives of General Psychiatry, 4(6), 561–571. https://doi.org/10.1001/archpsyc.1961.01710120031004
Beidas, R. S., Stewart, R. E., Walsh, L., Lucas, S., Downey, M. M., Jackson, K., Fernandez, T., & Mandell, D. S. (2015). Free, brief, and validated: Standardized instruments for low-resource mental health settings. Cognitive and Behavioral Practice, 22(1), 5–19. https://doi.org/10.1016/j.cbpra.2014.02.002
Bentler, P. M. (1990). Comparative fit indexes in structural models. Psychological Bulletin, 107(2), 238–246.
https://doi.org/10.1037/0033-2909.107.2.238
Bludworth, J. L., Tracey, T. J. G., & Glidden-Tracey, C. (2010). The bilevel structure of the Outcome Questionnaire–45. Psychological Assessment, 22(2), 350–355. https://doi.org/10.1037/a0019187
Browne, M. W., & Cudeck, R. (1993). Alternative ways of assessing model fit. In K. A. Bollen and J. S. Long (Eds.), Testing structural equation models. SAGE.
Byrne, B. M. (2016). Structural equation modeling with AMOS: Basic concepts, applications, and programming (3rd ed.). Routledge.
Carlson, R. G., Fripp, J., Munyon, M. D., Daire, A., Johnson, J. M., & DeLorenzi, L. (2014). Examining passive and active recruitment methods for low-income couples in relationship education. Marriage & Family Review, 50(1), 76–91. https://doi.org/10.1080/01494929.2013.851055
Carlson, R. G., Rappleyea, D. L., Daire, A. P., Harris, S. M., & Liu, X. (2017). The effectiveness of couple and individual relationship education: Distress as a moderator. Family Process, 56(1), 91–104.
https://doi.org/10.1111/famp.12172
Clark, M., Ausloos, C., Delaney, C., Waters, L., Salpietro, L., & Tippett, H. (2020). Best practices for counseling clients experiencing poverty: A grounded theory. Journal of Counseling & Development, 98(3), 283–294. https://doi.org/10.1002/jcad.12323
Costello, A. B., & Osborne, J. (2005). Best practices in exploratory factor analysis: Four recommendations for getting the most from your analysis. Practical Assessment, Research, and Evaluation, 10(7), 1–9.
https://doi.org/10.7275/jyj1-4868
Dakin, J., & Wampler, R. (2008). Money doesn’t buy happiness, but it helps: Marital satisfaction, psychological distress, and demographic differences between low- and middle-income clinic couples. The American Journal of Family Therapy, 36(4), 300–311. https://doi.org/10.1080/01926180701647512
Eid, M., Geiser, C., Koch, T., & Heene, M. (2017). Anomalous results in G-factor models: Explanations and alternatives. Psychological Methods, 22(3), 541–562. https://doi.org/10.1037/met0000083
Enders, C. K. (2010). Applied missing data analysis (1st ed.). Guilford.
Espiridion, E. D., Oladunjoye, A. O., Millsaps, U., & Yee, M. R. (2021). A retrospective review of the clinical significance of the Outcome Questionnaire (OQ) measure in patients at a psychiatric adult partial hospital program. Cureus, 13(3), e13830. https://doi.org/10.7759/cureus.13830
Foss-Kelly, L. L., Generali, M. M., & Kress, V. E. (2017). Counseling strategies for empowering people living in poverty: The I-CARE Model. Journal of Multicultural Counseling and Development, 45(3), 201–213.
https://doi.org/10.1002/jmcd.12074
Hair, J. F., Jr., Black, W. C., Babin, B. J., Anderson, R. E., & Tatham, R. L. (2010). Multivariate data analysis (6th ed.). Pearson.
Hatfield, D. R., & Ogles, B. M. (2004). The use of outcome measures by psychologists in clinical practice. Professional Psychology: Research and Practice, 35(5), 485–491. https://doi.org/10.1037/0735-7028.35.5.485
Henson, R. K., & Roberts, J. K. (2006). Use of exploratory factor analysis in published research: Common errors and some comment on improved practice. Educational and Psychological Measurement, 66(3), 393–416. https://doi.org/10.1177/0013164405282485
Hodgkinson, S., Godoy, L., Beers, L. S., & Lewin, A. (2017). Improving mental health access for low-income children and families in the primary care setting. Pediatrics, 139(1). https://doi.org/10.1542/peds.2015-1175
Horn, J. L. (1965). A rationale and test for the number of factors in factor analysis. Psychometrika, 30(2), 179–185. https://doi.org/10.1007/BF02289447
Horowitz, L. M., Bridge, J. A., Teach, S. J., Ballard, E., Klima, J., Rosenstein, D. L., Wharff, E. A., Ginnis, K., Cannon, E., Joshi, P., & Pao, M. (2012). Ask Suicide-Screening Questions (ASQ): A brief instrument for the pediatric emergency department. Archives of Pediatrics & Adolescent Medicine, 166(12), 1170–1176.
https://doi.org/10.1001/archpediatrics.2012.1276
Hu, L., & Bentler, P. M. (1995). Evaluating model fit. In R. H. Hoyle (Ed.), Structural equation modeling: Concepts, issues, and applications (pp. 76–99). SAGE.
Hu, L., & Bentler, P. M. (1999). Cutoff criteria for fit indexes in covariance structure analysis: Conventional criteria versus new alternatives. Structural Equation Modeling, 6(1), 1–55.
https://doi.org/10.1080/10705519909540118
Karney, B. R., & Bradbury, T. N. (2005). Contextual influences on marriage: Implications for policy and intervention. Current Directions in Psychological Science, 14(4), 171–174.
https://doi.org/10.1111/j.0963-7214.2005.00358.x
Kim, S.-H., Beretvas, S. N., & Sherry, A. R. (2010). A validation of the factor structure of OQ-45 scores using factor mixture modeling. Measurement and Evaluation in Counseling and Development, 42(4), 275–295.
https://doi.org/10.1177/0748175609354616
Kline, R. B. (2016). Principles and practice of structural equation modeling (4th ed.). Guilford.
Knifton, L., & Inglis, G. (2020). Poverty and mental health: Policy, practice and research implications. BJPsych Bulletin, 44(5), 193–196. https://doi.org/10.1192/bjb.2020.78
Kroenke, K., Spitzer, R. L., & Williams, J. B. W. (2001). The PHQ-9: Validity of a brief depression severity measure. Journal of General Internal Medicine, 16, 606–613. https://doi.org/10.1046/j.1525-1497.2001.016009606.x
Lam, J. R., Tyler, J., Scurrah, K. J., Reavley, N. J., & Dite, G. S. (2019). The association between socioeconomic status and psychological distress: A within and between twin study. Twin Research and Human Genetics, 22(5), 312–320. https://doi.org/10.1017/thg.2019.91
Lambert, M. J. (2017). Measuring clinical progress with the OQ-45 in a private practice setting. In S. Walfish, J. E. Barnett, & J. Zimmerman (Eds.), Handbook of private practice: Keys to success for mental health practitioners (pp. 78–93). Oxford University Press. https://doi.org/10.1093/med:psych/9780190272166.003.0007
Lambert, M. J., Burlingame, G. M., Umphress, V., Hansen, N. B., Vermeersch, D. A., Clouse, G. C., & Yanchar, S. C. (1996). The reliability and validity of the Outcome Questionnaire. Clinical Psychology and Psychotherapy, 3(4), 249–258. https://doi.org/10.1002/(SICI)1099-0879(199612)3:4<249::AID-CPP106>3.0.CO;2-S
Lambert, M. J., Gregersen, A. T., & Burlingame, G. M. (2004). The Outcome Questionnaire-45. In M. E. Maruish (Ed.), The use of psychological testing for treatment planning and outcomes assessment: Instruments for adults (3rd ed.; pp. 191–234). Routledge.
Lambert, M. J., Smart, D. W., Campbell, M. P., Hawkins, E. J., Harmon, C., & Slade, K. L. (2006). Psychotherapy outcome, as measured by the OQ-45, in African American, Asian/Pacific Islander, Latino/a, and Native American clients compared with matched Caucasian clients. Journal of College Student Psychotherapy, 20(4), 17–29. https://doi.org/10.1300/J035v20n04_03
Levy, H. C., Worden, B. L., Davies, C. D., Stevens, K., Katz, B. W., Mammo, L., Diefenbach, G. J., & Tolin, D. F. (2020). The dose-response curve in cognitive-behavioral therapy for anxiety disorders. Cognitive Behaviour Therapy, 49(6), 439–454. https://doi.org/10.1080/16506073.2020.1771413
Little, R. J. A. (1988). A test of missing completely at random for multivariate data with missing values. Journal of the American Statistical Association, 83(404), 1198–1202. https://doi.org/10.2307/2290157
Löwe, B., Decker, O., Müller, S., Brähler, E., Schellberg, D., Herzog, W., & Herzberg, P. Y. (2008). Validation and standardization of the Generalized Anxiety Disorder Screener (GAD-7) in the general population. Medical Care, 46(3), 266–274. https://doi.org/10.1097/MLR.0b013e318160d093
Mueller, R. M., Lambert, M. J., & Burlingame, G. M. (1998). Construct validity of the Outcome Questionnaire: A confirmatory factor analysis. Journal of Personality Assessment, 70(2), 248–262.
https://doi.org/10.1207/s15327752jpa7002_5
Muthén, L. K., & Muthén, B. O. (2015). Mplus: Statistical analysis with latent variables. User’s guide. (7th ed.)
https://www.statmodel.com/download/usersguide/MplusUserGuideVer_7.pdf
Mvududu, N. H., & Sink, C. A. (2013). Factor analysis in counseling research and practice. Counseling Outcome Research and Evaluation, 4(2), 75–98. https://doi.org/10.1177/2150137813494766
Näher, A.-F., Rummel-Kluge, C., & Hegerl, U. (2020). Associations of suicide rates with socioeconomic status and social isolation: Findings from longitudinal register and census data. Frontiers in Psychiatry, 10(898), 1–9. https://doi.org/10.3389/fpsyt.2019.00898
Osborne, J. W. (2013). Best practices in data cleaning. SAGE.
Pearson, M., Stanley, S. M., & Rhoades, G. K. (2015). Within My Reach leader manual. PREP for Individuals, Inc.
Rice, K. G., Suh, H., & Ege, E. (2014). Further evaluation of the Outcome Questionnaire–45.2. Measurement and Evaluation in Counseling and Development, 47(2), 102–117. https://doi.org/10.1177/0748175614522268
Santiago, C. D., Wadsworth, M. E., & Stump, J. (2011). Socioeconomic status, neighborhood disadvantage, and poverty-related stress: Prospective effects on psychological syndromes among diverse low-income families. Journal of Economic Psychology, 32(2), 218–230. https://doi.org/10.1016/j.joep.2009.10.008
Satorra, A., & Bentler, P. M. (1994). Corrections to test statistics and standard errors in covariance structure analysis. In A. von Eye & C. C. Clogg (Eds.), Latent variables analysis: Applications for developmental research (pp. 399–419). SAGE.
Semega, J., Kollar, M., Shrider, E. A., & Creamer, J. F. (2021). Income and poverty in the United States: 2019. Current population reports. U.S. Census Bureau.
https://www.census.gov/content/dam/Census/library/publications/2020/demo/p60-270.pdf
Stanford Center on Poverty and Inequality. (2015). State of the states: The poverty and inequality report. Pathways. http://inequality.stanford.edu/sites/default/files/SOTU_2015.pdf
Tabachnick, B. G., & Fidell, L. S. (2019). Using multivariate statistics (7th ed.). Pearson.
Tabet, S. M., Lambie, G. W., Jahani, S., & Rasoolimanesh, S. (2019). The factor structure of the Outcome Questionnaire–45.2 scores using confirmatory tetrad analysis—partial least squares. Journal of Psychoeducational Assessment. https://doi.org/10.1177/0734282919842035
Tucker, L. R., & Lewis, C. (1973). A reliability coefficient for maximum likelihood factor analysis. Psychometrika, 38, 1–10.
Dalena Dillman Taylor, PhD, LMHC, RPT-S, is an associate professor at the University of North Texas. Saundra M. Tabet, PhD, NCC, CCMHC, ACS, LMHC, is an assistant professor and CMHC Program Director at the University of San Diego. Megan A. Whitbeck, PhD, NCC, is an assistant professor at The University of Scranton. Ryan G. Carlson, PhD, is a professor at the University of South Carolina. Sejal Barden, PhD, is a professor at the University of Central Florida. Nicole Silverio is an assistant professor at the University of South Carolina. Correspondence may be addressed to Dalena Dillman Taylor, 1300 W. Highland St., Denton, TX 76201, Dalena.dillmantaylor@unt.edu.
Apr 1, 2021 | Volume 11 - Issue 2
Fei Shen, Yanhong Liu, Mansi Brat
The present study examined the relationships between childhood attachment, adult attachment, self-esteem, and psychological distress; specifically, it investigated the multiple mediating roles of self-esteem and adult attachment on the association between childhood attachment and psychological distress. Using 1,708 adult participants, a multiple-mediator model analysis following bootstrapping procedures was conducted in order to investigate the mechanisms among childhood and adult attachment, self-esteem, and psychological distress. As hypothesized, childhood attachment was significantly associated with self-esteem, adult attachment, and psychological distress. Self-esteem was found to be a significant mediator for the relationship between childhood attachment and adult attachment. In addition, adult attachment significantly mediated the relationship between self-esteem and psychological distress. The results provide insight on counseling interventions to increase adults’ self-esteem and attachment security, with efforts to decrease the negative impact of insecure childhood attachment on later psychological distress.
Keywords: childhood attachment, adult attachment, self-esteem, psychological distress, mediator
Attachment has been widely documented across disciplines, following Bowlby’s (1973) foundational work known as attachment theory. Attachment, in the context of child–parent interactions, is defined as a child’s behavioral tendency to use the primary caregiver as the secure base when exploring their surroundings (Bowlby, 1969; Sroufe & Waters, 1977). Research has shed light on the significance of childhood attachment in predicting individuals’ intrapersonal qualities such as self-esteem and emotion regulation during adulthood (Brennan & Morris, 1997), interpersonal orientations examined through attachment variation and adaptation across different developmental stages (Sroufe, 2005), and overall psychological well-being (Cassidy & Shaver, 2010; Wright et al., 2014).
Given its clinical significance, attachment has gained increased interest across disciplines. For example, childhood attachment was found to significantly predict coping and life satisfaction in young adulthood (Wright et al., 2017). Relatedly, a 30-year longitudinal study reinforces the vital role of childhood attachment in predicting individuals’ development of “the self and personality” (Sroufe, 2005, p. 352). Sroufe’s (2005) study reinforced the vital role of attachment across the life span. As an outcome variable, attachment is asserted to be associated with empathy (Ruckstaetter et al., 2017) and parenting practice in the adoptive population (Liu & Hazler, 2017). Considering the interplay between individuals’ relationship evolvement and their living contexts (Bowlby, 1973; Sroufe, 2005), attachment is examined at different stages generally labelled as childhood attachment and adult attachment, with the former focusing on the infant/child–parent relationship and the latter on adults’ generalized relationships with intimate others (e.g., romantic partners, close friends). Because of the abstract nature of attachment, it is commonly measured in the form of childhood attachment styles (Ainsworth et al., 1978) or adult attachment orientations (Turan et al., 2016).
Conceptual Framework
The present study is grounded in attachment theory, which is centered around a child’s ability to utilize their primary caregiver as the secure base when exploring surroundings, involving an appropriate balance between physical proximity, curiosity, and wariness (Bowlby, 1973; Sroufe & Waters, 1977). A core theoretical underpinning of attachment theory is the internal working model capturing a child’s self-concept and expectations of others (Bretherton, 1996). Internal working models of self and other are complementary. Namely, a child with strong internal working models is characterized with a perception of self as being worthy and deserving of love and a perception of others as being responsive, reliable, and nurturing (Bowlby, 1973; Sroufe, 2005).
In the context of attachment theory, childhood attachment is considered an outcome of consistent child–caregiver interactions and serves as the foundation for individuals’ later personality development (Bowbly, 1973; Sroufe, 2005). In line with child–caregiver interactions, Ainsworth et al. (1978) came up with three attachment styles based upon Bowlby’s seminal work, including secure, anxious-ambivalent, and anxious-avoidant attachment, following sequential phases of laboratory observations. Attachment theory was subsequently extended beyond the child–parent relationship to include later relationships in adulthood, given the parallels between these relationships (Cassidy & Shaver, 2010). Likewise, four distinct adult attachment styles (i.e., secure, dismissing, preoccupied, and fearful) are referred to based on the two-dimensional models of self and other (Konrath et al., 2014). Adult attachment styles are commonly examined under two orientations: attachment avoidance and attachment anxiety (Turan et al., 2016). Individuals showing low avoidance and low anxiety are considered securely attached, whereas those with high levels of anxiety and avoidance tend to be insecurely attached. Although childhood attachment and adult attachment are broadly considered distinct concepts in the literature, they share a spectrum of behaviors spanning from secure to insecure attachment. The levels of avoidance and anxiety involved in these behaviors are used as parameters to differentiate securely attached individuals from those who are insecurely attached.
Childhood Attachment, Self-Esteem, and Adult Attachment
Despite the conceptual overlaps, childhood attachment to caregivers and adult attachment to intimate others are commonly investigated as two distinct variables associated with individuals’ needs and features of different relationships. Childhood attachment captures a child’s distinct relationship with the primary caregiver (e.g., the mother figure) as well as their ability to differentiate the primary caregiver from other adults (Bowlby, 1969, 1973), whereas adult attachment may involve an individual’s multiple relationships (with parents, a romantic partner, or close friends). Noting the general stability of attachment from childhood to adulthood (Fraley, 2002), previous conceptual work stresses the importance of contexts in individuals’ attachment evolvement, highlighting that “patterns of adaptation” and “new experiences” reinforce each other in a reciprocal way (Sroufe, 2005, p. 349). For instance, an individual may develop secure attachment in adulthood because of healthy interpersonal experiences likely facilitated by trust, support, and nurturing received from significant others or their relationships, despite showing insecure attachment patterns in early childhood. A dynamic view of attachment development is thus warranted.
From a dynamic lens, researchers have generated evidence for the association between childhood attachment and adult attachment (Pascuzzo et al., 2013; Styron & Janoff-Bulman, 1997). For example, in a study of 879 college students (Styron & Janoff-Bulman, 1997), participants’ perception of their childhood attachment to both mother and father significantly predicted 7.9% of the variance in their adult attachment scores. Similarly, Pascuzzo et al. (2013) followed 56 adolescents at age 14 through age 22 and found that attachment insecurity to both parents and peers during adolescence was significantly associated with anxious romantic attachment in adulthood as measured by the Experience in Close Relationships Scale (ECR; Brennan et al., 1998). Studies that rely on retrospective data to assess childhood attachment (e.g., Styron & Janoff-Bulman, 1997) may be limited in validity because of time elapsed and potential compounding variables.
Childhood attachment is well recognized as the foundation for the growth of self-reliance and emotional regulation (Bowlby, 1973). Aligning with self-reliance, self-esteem appears to be frequently studied primarily through self-liking and self-competence (Brennan & Morris, 1997). Brennan and Morris (1997) defined self-liking as general self-evaluation based on perceived positive regard from others, and self-competence as concrete self-evaluation based on personal abilities and attributes. Previous research has suggested that secure attachment (to parents and peers) is significantly associated with higher levels of self-esteem (e.g., Wilkinson, 2004). In contrast, individuals who reported insecure attachment tended to endorse low self-esteem (Gamble & Roberts, 2005).
These results provide theoretical and empirical evidence for links between childhood attachment and adult attachment, but these links are likely to be indirect and mediated by other relevant variables from developmental perspectives. To our knowledge, no study has investigated the effect of self-esteem on the relationship between childhood attachment and adult attachment. The theoretical framework of attachment theory indicates that childhood attachment can have not only direct effects on adult attachment, but also indirect effects on adult attachment via self-esteem. In order to develop effective interventions tackling issues with adult attachment, it is important to examine potential mediators (e.g., self-esteem) between childhood attachment and adult attachment. To address this gap, the present study tests this hypothesized mediation function of self-esteem with a nonclinical sample of adults.
Self-Esteem, Attachment, and Psychological Distress
The extant literature comprises prolific information on the relationship between attachment and psychological well-being (Gnilka et al., 2013; Karreman & Vingerhoets, 2012; M. E. Kenny & Sirin, 2006; Turan et al., 2016; Wright et al., 2014). Existing evidence focuses on the relationship between adult attachment orientations and individuals’ psychological well-being (e.g., Karreman & Vingerhoets, 2012; Lynch, 2013; Roberts et al., 1996; Sowislo & Orth, 2013). Nevertheless, previous research has shed some light on the role of early childhood attachment in predicting psychological distresses in adulthood, including depression and anxiety (Bureau et al., 2009; Lecompte et al., 2014; Styron & Janoff-Bulman, 1997). Lecompte and colleagues (2014) conducted a longitudinal study of a sample of preschoolers (N = 68) with data collected at 4 years and again at 11–12 years; results of the study suggested that children with disorganized attachment at the baseline scored higher in both anxiety and depressive symptoms compared to those classified as securely attached.
Likewise, the effect of self-esteem on psychological distress is well established. A meta-analysis on 80 longitudinal studies published between 1994 and 2010 yielded consistent evidence supporting the relationship between low self-esteem and depressive symptoms (Sowislo & Orth, 2013). More recently, Masselink et al. (2018) examined data collected at four different points of participants’ development from early adolescence to young adulthood, which demonstrated that low self-esteem constitutes a persistent risk factor for participants’ depressive symptoms across developmental stages. Moreover, self-esteem scores in early adolescence significantly predicted the participants’ depressive symptoms at later stages, specifically during late adolescence and young adulthood.
Research has also supported the association between self-esteem, adult attachment, and psychological distress. Lopez and Gormley (2002) followed 207 college students from the beginning to the end of their freshman year and identified adjustment outcomes in association with the participants’ attachment styles and changes of their attachment styles measured by the ECR (e.g., secure-to-insecure attachment, insecure-to-secure attachment). The authors found that participants who remained securely attached scored higher in self-confidence and lower in both psychological distress and reactive coping compared to those who reported consistent insecure attachment. Moreover, participants who maintained secure attachment presented better outcomes in self-confidence and psychological well-being than the comparative group with secure-to-insecure or insecure-to-secure attachment changes (Lopez & Gormley, 2002). Adult attachment (measured by the ECR) was also found to be a mediator for the effects of traumatic events on post-traumatic symptomatology among a sample of female college students (Sandberg et al., 2010). In addition, Roberts et al. (1996) suggested attachment insecurity contributed to negative beliefs about oneself, which in turn activated cognitive structures of psychological distress, such as depression and anxiety, with a sample of 152 undergraduate students.
Taken together, the literature provides consistent support for the significant relationships between childhood attachment and various outcome variables in later adulthood, including adult attachment, self-esteem, and psychological distress. It further reveals a two-fold gap: (a) the variables tended to be investigated separately in previous studies, yet the mechanisms among these variables remained underexplored; and (b) little is known about the role of self-esteem and adult attachment in the association between childhood attachment and psychological distress. Disentangling the mechanisms, including potential mediating roles, involved in the variables will enrich the current knowledge based on attachment and can facilitate counseling interventions surrounding the effects of childhood attachment. In tackling the gap, three hypotheses were posed:
1. Childhood attachment is significantly associated with adult attachment, self-esteem, and psychological distress.
2. Self-esteem mediates the relationship between perceived childhood attachment and adult attachment.
3. Adult attachment mediates the relationship between self-esteem and
psychological distress.
Method
Participants
Of the 2,373 voluntary adult participants who took the survey, 1,708 (72%) completed 95% of all the questions and were retained for final analysis. Among the participants, 76.2% (n = 1,302) were female, 22.3% (n = 381) were male, and 1.3% (n = 25) chose not to specify their gender. The mean age of the participants was 29.89, ranging from 18 to 89 years old (SD = 12.44). A total of 66.3% (n = 1,133) of participants described themselves as White/European American, 8.7% (n = 148) as African American, 10.2% (n = 175) as Asian/Pacific Islander, 2.6% (n = 44) as American Indian/Native American, 7.3% (n = 124) as biracial or multiracial, 3.6% (n = 61) as other race, and 1.3% (n = 23) did not specify.
Sampling Procedures
The study was approved by the university’s IRB. We posted the recruitment information on various websites (e.g., Facebook, discussion board, university announcement board, Craigslist) in order to recruit a diverse pool of participants. Individuals who were 18 years old or above and were able to fill out the questionnaire in English were eligible for participating in this project. Participants were directed to an online Qualtrics survey consisting of the measures discussed in the following section. An informed consent form was included at the beginning of the survey outlining the confidentiality, voluntary participation, and anonymity of the study. Participants were prompted to enter their email addresses to win one of ten $15 e-gift cards. Participants’ email addresses were not included in the survey questions and data analysis.
Measures
Psychological Distress
Psychological distress was measured using the 10-item Kessler Psychological Distress Scale (K10; Kessler et al., 2003). Participants were asked about their emotional states in the past four weeks (e.g., “How often did you feel nervous?”). Responses were rated on a 5-point scale ranging from 0 (None of the time) to 4 (All of the time). Scores were averaged, with a higher score indicating a higher level of psychological distress. Previous studies using K10 have provided evidence of validity (Andrews & Slade, 2001). The internal consistency for K10 has been well established with a Cronbach’s alpha coefficient ranging from .88 (Easton et al., 2017) to .94 (Donker et al., 2010). In this study, the Cronbach’s alpha coefficient was .94.
Childhood Attachment
Childhood attachment was measured using the Parental Attachment subscale of the Inventory of Parent and Peer Attachment (IPPA; Armsden & Greenberg, 1987). Previous research has demonstrated evidence that this measure has great convergent and concurrent validity (M. E. Kenny & Sirin, 2006). The IPPA has been used to recall childhood attachment in adult populations (Aspelmeier et al., 2007; Cummings-Robeau et al., 2009). This 25-item subscale directs participants to recall their attachment to the parent(s) or caregiver(s) who had the most influence on them during childhood. The subscale consists of three dimensions, including 10 items on trust, nine items on communication, and six items on alienation. Some sample items are: “My parent(s)/primary caregiver(s) accepts me as I am” for trust, “I tell my parent(s)/primary caregiver(s) about my problems and troubles)” for communication, and “I do not get much attention from my parent(s)/primary caregiver(s)” for alienation. Participants rated the items using a 5-point Likert scale ranging from 1 (Almost never or never true) to 5 (Almost always or always true). Items were averaged to form the subscale, with a higher score reflecting more secure childhood attachment. The subscale has demonstrated high internal consistency with a Cronbach’s alpha of .93 (Armsden & Greenberg, 1987). In the present study, Cronbach’s alpha for the subscale was .96.
Adult Attachment
Adult attachment was measured using the ECR (Brennan et al., 1998). The ECR consists of 36 items with 18 items assessing each of the two orientations: attachment anxiety and attachment avoidance. In order to avoid confounding factors, we only assessed adult attachment with close friends or romantic partners, as relationships with parents can confound the childhood attachment outcomes. Responses were rated on a 7-point Likert scale ranging from 1 (Strongly disagree) to 7 (Strongly agree). Two scores were averaged, with a higher score reflecting a higher level of attachment anxiety or avoidance. In terms of validity, the ECR subscales have been found to be positively associated with psychological distress and intention to seek counseling, and negatively associated with social support (Vogel & Wei, 2005). The ECR has a high internal consistency for both the anxiety (α = .91) and avoidance (α = .94) dimensions (Brennan et al., 1998). For this study, Cronbach’s alphas for attachment anxiety and attachment avoidance were .93 and .92, respectively.
Self-Esteem
Rosenberg’s Self-Esteem Scale (RSES; Rosenberg, 1965) is a 10-item scale designed to assess an adult’s self-esteem. The scale assesses both self-competency (e.g., “I feel that I have a number of good qualities”) and self-liking (e.g., “I certainly feel useless at times”). Responses were coded using a 4-point Likert scale ranging from 1 (Strongly disagree) to 4 (Strongly agree). Negatively worded statements were reverse-coded. Scores were averaged, with a higher score reflecting a higher level of self-esteem. RSES has been frequently used in various studies with high reliability and validity (Brennan & Morris, 1997; Chen et al., 2017). In this study, the Cronbach’s alpha coefficient was .89.
Data Analysis
Descriptive statistics were computed using SPSS version 23 followed by a multiple-mediator model analysis using Mplus version 7.4 (Muthén & Muthén, 2012). Missing data were treated with the full information maximum likelihood estimation in Mplus, which was one of the most pragmatic approaches in producing unbiased parameter estimates (Acock, 2005). The multiple-mediator model includes childhood attachment as the predictor, self-esteem and adult attachment anxiety and avoidance as mediators, and psychological distress as the outcome variable (see Figure 1). The mediation analysis was conducted using bootstrapping procedures (J = 2,000), which was a resampling method to construct a confidence interval for the indirect effect (Preacher & Hayes, 2008). Several model fit indices based on Kline’s (2010) guidelines were employed, including the ratio of chi-square to degree of freedom (χ2/df), root-mean-square error of approximation (RMSEA), Tucker-Lewis index (TLI), comparative fit index (CFI), and standardized root-mean-square residual (SRMR). Indicators of good model fit are a nonsignificant chi-square value, a CFI and TLI of .90 or greater, RMSEA of .08 or less, and an SRMR of .05 or less (Hooper et al., 2008).
Figure 1
Multiple-Mediator Model: Self-Esteem, Anxious Adult Attachment, and Avoidant Adult Attachment as Multiple Mediators Between Childhood Attachment and Psychological Distress
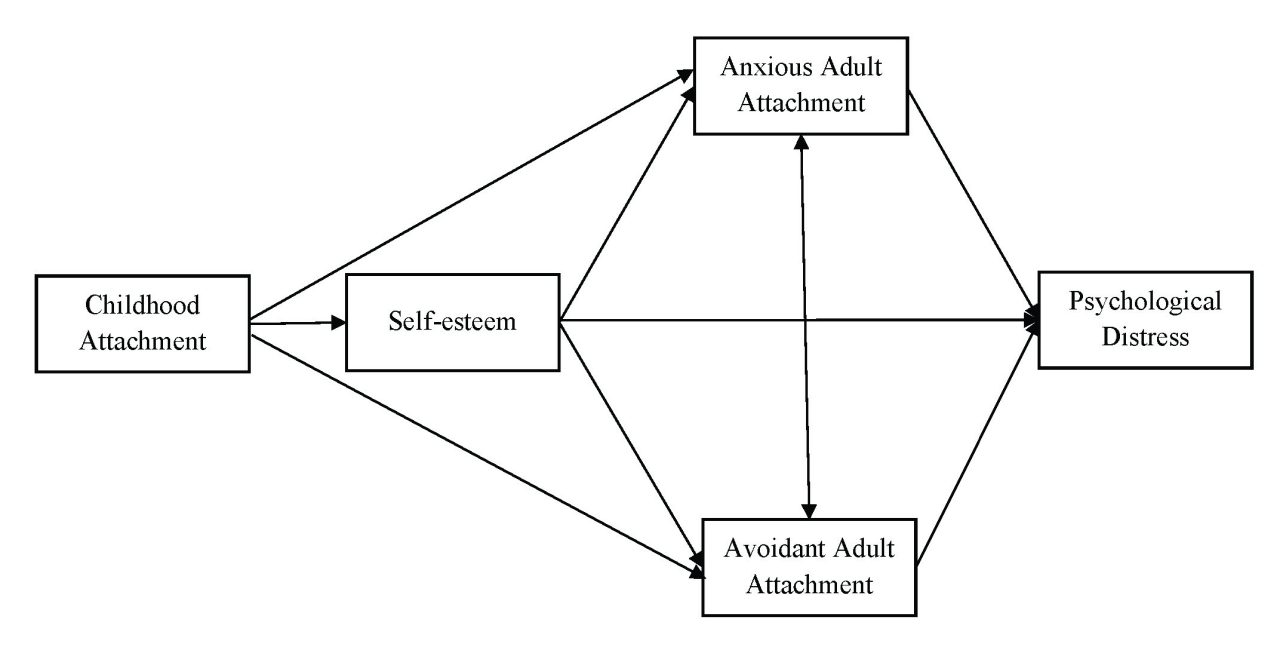
Results
Descriptive Statistics and Correlations
The descriptive statistics of each variable are reported in Table 1.
Table 1
Descriptive Statistics for Variables (N = 1,708)
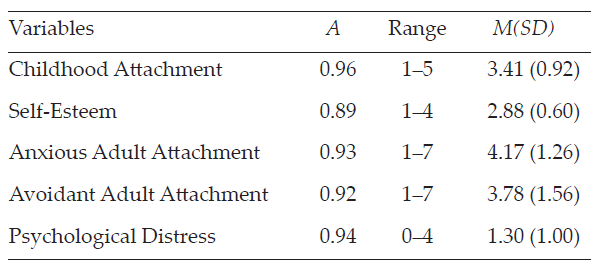
Pearson’s correlations between variables were computed. All bivariate statistics are presented in Table 2 and provided full support for our Hypothesis 1. For instance, childhood attachment was positively associated with self-esteem (r = .38, p < .001) and negatively correlated with adult attachment anxiety (r = -.26, p < .001) and avoidance (r = -.45, p < .001), as well as with psychological distress (r = -.35, p < .001). Significant negative correlations were found between self-esteem and adult attachment anxiety (r = -.49, p < .001) and avoidance (r = -.46, p < .001), and between self-esteem and psychological distress (r = -.63, p < .001). Both adult attachment anxiety (r = .57, p < .001) and avoidance (r = .42, p < .001) were positively associated with psychological distress. Significant correlation was found between adult attachment anxiety and avoidance (r = .31, p < .001).
Table 2
Correlation Matrix of Variables (N = 1,708)
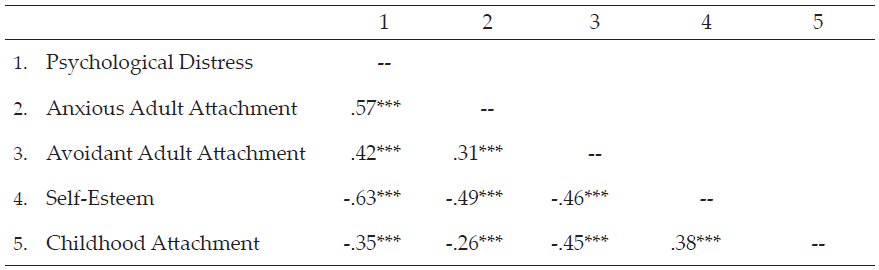
*p < .05. **p < .01. ***p < .001 (two-tailed).
The Multiple-Mediator Model
The multiple-mediator model involving self-esteem and adult attachment as mediators, with bootstrapping procedures, yielded satisfactory fit indices: χ2(1) = 12.24, p < .001, CFI = 1.00, TLI = 0.96, SRMR = .01. However, the index of RMSEA = .08, 90% CI [0.05, 0.12] indicated a mediocre fit, with the upper value of 90% CI larger than the suggested cutoff score of 0.08. D. A. Kenny et al. (2015) suggested that the models with small degrees of freedom had the average width of the 90% CI above 0.10, unless the sample size was extremely large. The nonsignificant χ2 value was interpreted as a good fit index.
The present study further revealed that secure childhood attachment was associated with high self-esteem (β = .25, p < .001) and low levels of anxiety (β = -.12, p < .001) and avoidance (β = -.41, p < .001) of adult attachment. Meanwhile, high self-esteem was associated with low anxiety (β = -.95, p < .001) and low avoidance (β = -.64, p < .001) of adult attachment. In addition, high self-esteem (β = -.68, p < .001) and low adult attachment anxiety (β = .26, p < .001) and avoidance (β = .11, p < .001) were significantly associated with low psychological distress. The results supported both Hypotheses 2 and 3 in that self-esteem mediated the relationship between childhood attachment and adult attachment, and adult attachment mediated the relationship between self-esteem and psychological distress.
The mediating role of self-esteem was examined using bootstrapping procedures. Results demonstrated that self-esteem significantly mediated the association between childhood attachment and adult attachment anxiety (b = -.24, 95% CI [-0.27, -0.21]) and avoidance (b = -.16, 95% CI [-0.19, -0.14]).
The present study further supported the mediating role of adult attachment (i.e., anxiety and avoidance). The association between self-esteem and psychological distress was significantly mediated by both adult attachment anxiety (b = -.24, 95% CI [-0.29, -0.21]) and avoidance (b = -.07, 95% CI [-0.10, -0.05]). Mediation effects are denoted in Table 3.
Table 3
Mediation Analysis With Bootstrapping: Unstandardized and Standardized Estimates and Confidence Intervals for Mediation Effects
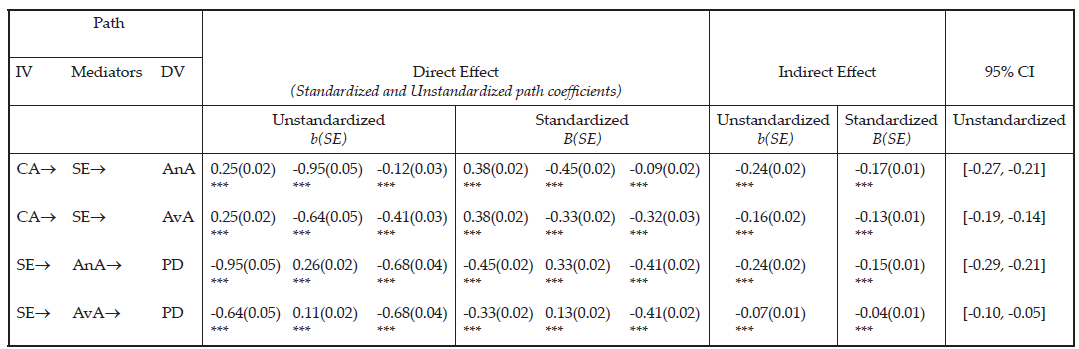
Note. Bootstrap J = 2,000, CI = confidence interval; IV = independent variable; DV = dependent variable; CA = Childhood Attachment; SE = Self-Esteem; AnA = Anxious Adult Attachment; AvA = Avoidant Adult Attachment; PD = Psychological Distress. Direct effect of path direction, IV® Mediator, Mediator ® DV, IV ® DV. Statistical significance was evaluated based on whether 95% bias corrected bootstrap CIs include zero or not. If zero was included in the CI, then it was not a significant indirect effect. Model fit: χ2(1) = 12.24, p < .001, CFI = 1.00, TLI = 0.96, SRMR = .01, RMSEA = .08 (90% CI [0.05, 0.12]).
Discussion
The present study highlights the significance of childhood attachment and its associations with self-esteem and psychological distress in adulthood. Participants who reported secure childhood attachment scored higher on self-esteem and lower on psychological distress. Secure childhood attachment was also found to be associated with low adult attachment anxiety and avoidance. Our study builds upon previous research (e.g., Sroufe, 2005) to capture the complexity of key variables related to attachment and its evolvement from childhood to adulthood. The results shed further light on the mechanisms among childhood attachment, self-esteem, adult attachment, and psychological distress. Self-esteem was found to be a significant mediator between childhood attachment and adult attachment; meanwhile, adult attachment was found to be a mediator between self-esteem and psychological distress.
The findings support Hypothesis 1 in that individuals with more secure childhood attachment reported higher levels of self-esteem, lower levels of adult attachment anxiety and avoidance, and less psychological distress. The results echo attachment theory (Bowlby, 1973), positing childhood attachment as a predictor of later adjustment as well as self-esteem, indicating that the quality of attachment appears to be intimately related to how to cope with stress and how to perceive oneself (Wilkinson, 2004). The results are also consistent with previous research that highlighted secure childhood attachment as a protective factor against anxiety, depression, and later emotional and relational distress (e.g., Karreman & Vingerhoets, 2012).
Results also lend support to Hypothesis 2 in that self-esteem mediated the relationship between childhood attachment and adult attachment. Self-esteem as a mediator echoed previous research that indicated the influence of childhood attachment on one’s self-esteem may be mitigated by expanded social networks in adulthood (Steiger et al., 2014). For instance, it is likely that improving self-esteem through peer connections (e.g., friendship; romantic relationships) may contribute to individuals’ adaptation to close relationships and enhance attachment security in adulthood, despite their insecure attachment with primary caregivers in childhood (Fraley, 2002; Sroufe, 2005).
Congruent with Hypothesis 3, adult attachment was a mediator for the relationship between self-esteem and psychological distress. Previous research provided evidence that low self-esteem increases the risk of developing psychological distress such as depressive and anxious symptoms (Li et al., 2014); nevertheless, individuals may experience less psychological impact with secure attachment manifested through their close relationships. Little is known about the relationship between insecure adult attachment (i.e., anxious and avoidant attachment) and psychological distress, and the mediating role of adult attachment has rarely been addressed. In a sample of 154 women in a community context, Bifulco et al. (2006) found that fearful and angry-dismissive attachment partially mediated the relationship between childhood adversity and depression or anxiety. The present study extends the Bifulco et al. study to include a larger, gender-inclusive, and racially diverse population that captures a wider age range. Further, using continuous measurements, the present study counteracts the limitations of dichotomous measures used in Bifulco et al.’s study, thus reflecting the spectrum and complexity of attachment.
Implications for Counseling Practice
The present study sheds light on interventions for clients’ psychological distress. The results corroborated positive associations between psychological distress and insecure childhood attachment and attachment anxiety and avoidance during adulthood. Although adults can no longer change their childhood experiences, including their attachment-related adversities, interventions that target improving adult attachment may still mitigate the negative effect of childhood attachment on psychological distress later during adulthood. Considering the reciprocal influence noted between self-esteem and adult attachment (Foster et al., 2007), counseling strategies encompassing both self-esteem and adult attachment are thus desirable.
Specifically, counselors could conceptualize self-esteem in a relational context in which they may incorporate clients’ support systems (e.g., partner, close friends, parents) into the treatment. A key treatment goal may be utilizing close relationships to boost self-esteem. On the contrary, counselors may engage clients with low self-esteem in communicating their attachment needs while involving significant others (e.g., partners) to enact positive responses, such as attentive listening and validation of mutual needs. Counselors are encouraged to assess how childhood attachment experiences may have influenced the client’s adult attachment, as insecure attachment may lead to challenges with perceived trustworthiness of self and others, which could hinder growth in the interpersonal relationships. Clients may further benefit from reflecting over specific attachment behaviors and interactional patterns within close relationships (e.g., how they manage proximity to an attachment figure when they experience distress) in order to restructure and enhance their attachment security internally and externally (Cassidy et al., 2013).
The finding of self-esteem as a significant mediator supports the proposition that self-esteem is responsive to life events and that these can influence one’s perception and evaluation of self. Previous research indicated that individuals with low self-esteem may be easily triggered by stressful life events and consequently respond irrationally and negatively (Taylor & Montgomery, 2007). Counselors may consider adapting Fennell’s (1997) Cognitive Behavioral Therapy model comprising early experience, bottom line, and rules for living to help clients enhance self-esteem. Fennell’s model suggests that clients’ early experiences (e.g., childhood attachment, traumatic experience, cultural context) may have an influence on the development of a fundamental bottom line about themselves (e.g., “I am not good enough,” “I am worthless”). Counselors may further assist clients with mapping out the rules for living (e.g., dysfunctional assumptions) related to distorted thoughts on what they should do in order to cultivate their core beliefs (as being loved or accepted or vice versa). For example, if clients have formed insecure attachment during childhood (early experience), they may develop a bottom line that “I am not good enough.” In making efforts to feel accepted in the family, they may have the rules for living that “I have to receive all As in all my classes.” If clients fail to achieve the rules for living, they likely would develop anxious and depressive symptoms, which may activate the confirmation of the bottom line. To counteract the negative patterns, counselors may work with clients to process the impact of early experience (e.g., early insecure attachment) on their bottom line and revise the rules of living to develop healthier coping strategies. When clients develop alternative rules of living, counselors may further help them to re-evaluate the bottom line and enhance self-acceptance.
Limitations and Future Research Directions
Although the results supported all three hypotheses, the present study was subject to a few limitations. First, the self-report measures may have been subject to biases, especially for the memory of childhood attachment. Another limitation pertains to a retrospective assessment of perceptions of childhood attachment that may be changed over time because of life experiences (e.g., death, parental divorce). Relatedly, the cross-sectional study could not capture the changes over a period of time. Not knowing the types of childhood attachment (i.e., anxious attachment, avoidant attachment) presented as another limitation for researchers’ understanding of the variations of attachment and how each type might impact long-term outcomes. In the future, researchers may consider longitudinal studies to explore the variations and changes in attachment over the life span and examine what other mechanisms contribute to the changes to protect against the negative impact. Future research may also incorporate other-report data filled out by significant others (e.g., parents, romantic partners) to minimize social desirability and provide multiple perspectives.
Conclusion
Attachment theory provides a strong theoretical framework in understanding individuals’ psychological well-being over the life span (DeKlyen & Greenberg, 2008). Informed by attachment theory, the present study investigated the mediating roles of self-esteem and adult attachment (measured through the levels of anxiety and avoidance) on the relations between childhood attachment and psychological distress, and between self-esteem and psychological distress, respectively. The multiple-mediator analysis with bootstrapping supports both self-esteem and adult attachment as significant mediators. Our results also support the associations between childhood attachment with self-esteem, adult attachment, and psychological distress. The study contributes to the gap pertaining to adult attachment and provides practical implications for counselors working in various settings in their work with clients surrounding attachment security, self-esteem, and psychological well-being.
Conflict of Interest and Funding Disclosure
The authors reported no conflict of interest
or funding contributions for the development
of this manuscript.
References
Acock, A. C. (2005). Working with missing values. Journal of Marriage and Family Therapy, 67(4), 1012–1028.
https://doi.org/10.1111/j.1741-3737.2005.00191.x
Ainsworth, M. D. S., Blehar, M. C., Waters, E., & Wall, S. (1978). Patterns of attachment: A psychological study of the strange situation. Lawrence Erlbaum.
Andrews, G., & Slade, T. (2001). Interpreting scores on the Kessler Psychological Distress Scale (K10). Australian and New Zealand Journal of Public Health, 25(6), 494–497. https://doi.org/10.1111/j.1467-842x.2001.tb00310.x
Armsden, G. C., & Greenberg, M. T. (1987). The Inventory of Parent and Peer Attachment: Individual differences and their relationship to psychological well-being in adolescence. Journal of Youth and Adolescence, 16(5), 427–454. https://doi.org/10.1007/BF02202939
Aspelmeier, J. E., Elliott, A. N., & Smith, C. H. (2007). Childhood sexual abuse, attachment, and trauma symptoms in college females: The moderating role of attachment. Child Abuse & Neglect, 31(5), 549–566.
https://doi.org/10.1016/j.chiabu.2006.12.002
Bifulco, A., Kwon, J., Jacobs, C., Moran, P. M., Bunn, A., & Beer, N. (2006). Adult attachment style as mediator between childhood neglect/abuse and adult depression and anxiety. Social Psychiatry and Psychiatric Epidemiology, 41, 796–805. https://doi.org/10.1007/s00127-006-0101-z
Bowlby, J. (1969). Attachment and loss, Vol. 1: Attachment. Basic Books.
Bowlby, J. (1973). Attachment and loss. Vol. 2: Separation: Anxiety and anger. Basic Books.
Brennan, K. A., Clark, C. L., & Shaver, P. R. (1998). Self-report measurement of adult attachment: An integrative overview. In J. A. Simpson & W. S. Rholes (Eds.), Attachment theory and close relationships (pp. 46–76). Guilford.
Brennan, K. A., & Morris, K. A. (1997). Attachment styles, self-esteem, and patterns of seeking feedback from romantic partners. Personality and Social Psychology Bulletin, 23(1), 23–31.
https://doi.org/10.1177/0146167297231003
Bretherton, I. (1996). Internal working models of attachment relationships as related to resilient coping. In G. G. Noam & K. W. Fischer (Eds.), Development and vulnerability in close relationships (pp. 3–27). Lawrence Erlbaum.
Bureau, J. F., Easlerbrooks, M. A., & Lyons-Ruth, K. (2009). Attachment disorganization and controlling behavior in middle childhood: Maternal and child precursors and correlates. Attachment & Human Development, 11(3), 265–284. https://doi.org/10.1080/14616730902814788
Cassidy, J., Jones, J. D., & Shaver, P. R. (2013). Contributions of attachment theory and research: A framework for future research, translation, and policy. Development and Psychopathology, 25(4), 1415–1434.
https://doi.org/10.1017/S0954579413000692
Cassidy, J., & Shaver, P. R. (Eds.). (2010). Handbook of attachment: Theory, research, and clinical applications (2nd ed.). Guilford.
Chen, W., Zhang, D., Pan, Y., Hu, T., Liu, G., & Luo, S. (2017). Perceived social support and self-esteem as mediators of the relationship between parental attachment and life satisfaction among Chinese adolescents. Personality and Individual Differences, 108, 98–102. https://doi.org/10.1016/j.paid.2016.12.009
Cummings-Robeau, T. L., Lopez, F. G., & Rice, K. G. (2009). Attachment-related predictors of college students’ problems with interpersonal sensitivity and aggression. Journal of Social and Clinical Psychology, 28(3),
364–391. https://doi.org/10.1521/jscp.2009.28.3.364
DeKlyen, M., & Greenberg, M. T. (2008). Attachment and psychopathology in childhood. In J. Cassidy & P. R. Shaver (Eds.), Handbook of attachment: Theory, research, and clinical applications (2nd ed., pp. 637–665). Guilford.
Donker, T., Comijs, H., Cuijpers, P., Terluin, B., Nolen, W., Zitman, F., & Penninx, B. (2010). The validity of the Dutch K10 and extended K10 screening scales for depressive and anxiety disorders. Psychiatry Research, 176(1), 45–50. https://doi.org/10.1016/j.psychres.2009.01.012
Easton, S. D., Safadi, N, S., Wang, Y., & Hasson, R. G., III. (2017). The Kessler Psychological Distress Scale: Translation and validation of an Arabic version. Health and Quality of Life Outcomes, 15(1), 215.
https://doi.org/10.1186/s12955-017-0783-9
Fennell, M. J. V. (1997). Low self-esteem: A cognitive perspective. Behavioural and Cognitive Psychotherapy, 25(1), 1–26. https://doi.org/10.1017/S1352465800015368
Foster, J. D., Kernis, M. H., & Goldman, B. M. (2007). Linking adult attachment to self-esteem stability. Self and Identity, 6(1), 64–73. https://doi.org/10.1080/15298860600832139
Fraley, R. C. (2002). Attachment stability from infancy to adulthood: Meta-analysis and dynamic modeling of developmental mechanisms. Personality and Social Psychology Review, 6(2), 123–151.
https://doi.org/10.1207/S15327957PSPR0602_03
Gamble, S. A., & Roberts, J. E. (2005). Adolescents’ perceptions of primary caregivers and cognitive style: The roles of attachment security and gender. Cognitive Therapy and Research, 29, 123–141.
https://doi.org/10.1007/s10608-005-3160-7
Gnilka, P. B., Ashby, J. S., & Noble, C. M. (2013). Adaptive and maladaptive perfectionism as mediators of adult attachment styles and depression, hopelessness, and life satisfaction. Journal of Counseling & Development, 91(1), 78–86. https://doi.org/10.1002/j.1556-6676.2013.00074.x
Hooper, D., Coughlan, J., & Mullen, M. R. (2008). Structural equation modeling: Guidelines for determining model fit. Electronic Journal on Business Research Methods, 6(1), 53–60.
Karreman, A., & Vingerhoets, A. J. J. M. (2012). Attachment and well-being: The mediating role of emotion regulation and resilience. Personality and Individual Differences, 53(7), 821–826.
https://doi.org/10.1016/j.paid.2012.06.014
Kenny, D. A., Kaniskan, B., & McCoach, D. B. (2015). The performance of RMSEA in models with small degrees of freedom. Sociological Methods & Research, 44(3), 486–507. https://doi.org/10.1177/0049124114543236
Kenny, M. E., & Sirin, S. R. (2006). Parental attachment, self-worth, and depressive symptoms among emerging adults. Journal of Counseling & Development, 84(1), 61–71. https://doi.org/10.1002/j.1556-6678.2006.tb00380.x
Kessler, R. C., Barker, P. R., Colpe, L. J., Epstein, J. F., Gfroerer, J. C., Hiripi, E., Howes, M. J., Normand, S.-L. T., Manderscheid, R. W., Walters, E. E., & Zaslavsky, A. M. (2003). Screening for serious mental illness in the general population. Archives of General Psychiatry, 60(2), 184–189. https://doi.org/10.1001/archpsyc.60.2.184
Kline, R. B. (2010). Principles and practice of structural equation modeling (3rd ed.). Guilford.
Konrath, S. H., Chiopik, W. J., Hsing, C. K., & O’Brien, E. (2014). Changes in adult attachment styles in American college students over time: A meta-analysis. Personality and Social Psychology Review, 18(4), 326–348.
https://doi.org/10.1177/1088868314530516
Lecompte, V., Moss, E., Cyr, C., & Pascuzzo, K. (2014). Preschool attachment, self-esteem and the development of preadolescent anxiety and depressive symptoms. Attachment & Human Development, 16(3), 242–260. https://doi.org/10.1080/14616734.2013.873816
Li, S. T., Albert, A. B., & Dwelle, D. G. (2014). Parental and peer support as predictors of depression and self-esteem among college students. Journal of College Student Development, 55(2), 120–138.
https://doi.org/10.1353/csd.2014.0015
Liu, Y., & Hazler, R. J. (2017). Predictors of attachment security in children adopted from China by US families: Implication for professional counsellors. Asia Pacific Journal of Counselling and Psychotherapy, 8(2), 115–130. https://doi.org/10.1080/21507686.2017.1342675
Lopez, F. G., & Gormley, B. (2002). Stability and change in adult attachment style over the first-year college transition: Relations to self-confidence, coping, and distress patterns. Journal of Counseling Psychology, 49(3), 355–364. https://doi.org/10.1037/0022-0167.49.3.355
Lynch, M. F. (2013). Attachment, autonomy, and emotional reliance: A multilevel model. Journal of Counseling & Development, 91(3), 301–312. https://doi.org/10.1002/j.1556-6676.2013.00098.x
Masselink, M., Van Roekel, E., & Oldehinkel, A. J. (2018). Self-esteem in early adolescence as predictor of depressive symptoms in late adolescence and early adulthood: The mediating role of motivational and social factors. Journal of Youth and Adolescence, 47, 932–946. https://doi.org/10.1007/s10964-017-0727-z
Muthén, L. K., & Muthén, B. O. (2012). Mplus user’s guide (7th ed.). http://www.statmodel.com/download/users
guide/Mplus%20user%20guide%20Ver_7_r3_web.pdf
Pascuzzo, K., Cyr, C., & Moss, E. (2013). Longitudinal association between adolescent attachment, adult romantic attachment, and emotion regulation strategies. Attachment & Human Development, 15(1), 83–103.
https://doi.org/10.1080/14616734.2013.745713
Preacher, K. J., & Hayes, A. F. (2008). Asymptotic and resampling strategies for assessing and comparing indirect effects in multiple mediator models. Behavior Research Methods, 40(3), 879–891.
https://doi.org/10.3758/BRM.40.3.879
Roberts, J. E., Gotlib, I. H., & Kassel, J. D. (1996). Adult attachment security and symptoms of depression: The mediating roles of dysfunctional attitudes and low self-esteem. Journal of Personality and Social Psychology, 70(2), 310–320. https://doi.org/10.1037/0022-3514.70.2.310
Rosenberg, M. (1965). Society and the adolescent self-image. Princeton University Press.
Ruckstaetter, J., Sells, J., Newmeyer, M. D., & Zink, D. (2017). Parental apologies, empathy, shame, guilt, and attachment: A path analysis. Journal of Counseling & Development, 95(4), 389–400.
https://doi.org/10.1002/jcad.12154
Sandberg, D. A., Suess, E. A., & Heaton, J. L. (2010). Attachment anxiety as a mediator of the relationship between interpersonal trauma and posttraumatic symptomatology among college women. Journal of Interpersonal Violence, 25(1), 33–49. https://doi.org/10.1177/0886260508329126
Sowislo, J. F., & Orth, U. (2013). Does low self-esteem predict depression and anxiety? A meta-analysis of longitudinal studies. Psychological Bulletin, 139(1), 213–240. https://doi.org/10.1037/a0028931
Sroufe, L. A. (2005). Attachment and development: A prospective, longitudinal study from birth to adulthood. Attachment & Human Development, 7(4), 349–367. https://doi.org/10.1080/14616730500365928
Sroufe, L. A., & Waters, E. (1977). Attachment as an organizational construct. Child Development, 48(4), 1184–1199. https://doi.org/10.2307/1128475
Steiger, A. E., Allemand, M., Robins, R. W., & Fend, H. A. (2014). Low and decreasing self-esteem in adolescence predict adult depression two decades later. Journal of Personality and Social Psychology, 106(2), 325–338. https://doi.org/10.1037/a0035133
Styron, T., & Janoff-Bulman, R. (1997). Childhood attachment and abuse: Long-term effects on adult attachment, depression, and conflict resolution. Child Abuse & Neglect, 21(10), 1015–1023.
https://doi.org/10.1016/S0145-2134(97)00062-8
Taylor, T. L., & Montgomery, P. (2007). Can cognitive-behavioral therapy increase self-esteem among depressed adolescents? A systematic review. Children and Youth Services Review, 29(7), 823–839.
https://doi.org/10.1016/j.childyouth.2007.01.010
Turan, N., Kocalevent, R.-D., Quintana, S. M., Erdur-Baker, Ö., & Diestelmann, J. (2016). Attachment orientations: Predicting psychological distress in German and Turkish samples. Journal of Counseling & Development, 94(1), 91–102. https://doi.org/10.1002/jcad.12065
Vogel, D. L., & Wei, M. (2005). Adult attachment and help-seeking intent: The mediating roles of psychological distress and perceived social support. Journal of Counseling Psychology, 52(3), 347–357.
https://doi.org/10.1037/0022-0167.52.3.347
Wilkinson, R. B. (2004). The role of parental and peer attachment in the psychological health and self-esteem of adolescents. Journal of Youth and Adolescence, 33, 479–493.
https://doi.org/10.1023/B:JOYO.0000048063.59425.20
Wright, S. L., Firsick, D. M., Kacmarski, J. A., & Jenkins-Guarnieri, M. A. (2017). Effects of attachment on coping efficacy, career decision self-efficacy, and life satisfaction. Journal of Counseling & Development, 95(4), 445–456. https://doi.org/10.1002/jcad.12159
Wright, S. L., Perrone-McGovern, K. M., Boo, J. N., & White, A.V. (2014). Influential factors in academic and career self-efficacy: Attachment, supports, and career barriers. Journal of Counseling & Development, 92(1), 36–46. https://doi.org/10.1002/j.1556-6676.2014.00128.x
Fei Shen, PhD, is a staff therapist at Syracuse University. Yanhong Liu, PhD, NCC, is an assistant professor at Syracuse University. Mansi Brat, PhD, LPC, is a staff therapist at Syracuse University. Correspondence may be addressed to Fei Shen, 150 Sims Drive, Syracuse, NY 13210, fshen02@syr.edu.
Apr 1, 2021 | Volume 11 - Issue 2
Melissa J. Sitton, Tina Du Rocher Schudlich, Christina Byrne
A psychosocial approach to predicting self-injurious behavior (SIB) may allow for more accurate predictions and enhance intervention for individuals who engage in SIB. We examined psychosocial predictors of SIB within and between two populations: individuals with traits of borderline personality disorder (BPD; N = 60) and college students (N = 116). All participants met the inclusion criteria of engaging in SIB at least once in the past year. All participants completed measures of psychological distress, social functioning, and SIB. Methods of SIB did not vary across samples, but SIB rates did. Psychological distress and population type (BPD or student) predicted SIB, whereas social factors did not. Additionally, we found a significant interaction wherein psychological distress was more related to SIB in individuals with traits of BPD. Accordingly, we recommend that counselors consider population and psychological distress when assessing SIB risk in clients.
Keywords: self-injurious behavior, borderline personality disorder, college students, psychological distress, social functioning
Self-injurious behavior (SIB), the deliberate act of self-inflicted bodily harm, is of growing concern to counselors and clinicians. According to Nock (2010), SIB is a broad concept encompassing self-injury completed with suicidal intent (i.e., suicide attempts), without suicidal intent (i.e., nonsuicidal self-injury), or with ambivalence toward life (i.e., ambivalent, meaning neither strictly suicidal nor nonsuicidal). In other words, an individual can engage in SIB with differing goals that vary in intent from harming themselves to dying. The American Psychiatric Association (2013) considers suicide behavior disorder and nonsuicidal self-injury to be “conditions for further study” (p. 801). Individuals who engage in SIB over time are likely to do so with greater frequency, more methods, and increasing lethality (Andrews et al., 2013). Therefore, there is a great need for counselors and clinicians to assess their clients for SIB.
Although there are differing theories of the development and maintenance of SIB based on intent, particularly regarding the development of suicidal and nonsuicidal SIB, there are similar intrapersonal and interpersonal themes across theories. For instance, in their four-function model of nonsuicidal SIB, Nock and Prinstein (2004, 2005) proposed that intrapersonal (e.g., affective) and interpersonal (e.g., help-seeking) factors act as positive and negative reinforcers of nonsuicidal SIB. Similarly, in their renowned interpersonal–psychological theory of suicide, Joiner and colleagues (Joiner, 2005; Van Orden et al., 2010) proposed that individuals who attempt suicide are characterized both by a desire to die (i.e., interpersonal factors of perceived burdensomeness and thwarted belongingness) and the acquired capability to attempt (i.e., intrapersonal factors such as past SIB).
Notably, there is no specific theory to date regarding ambivalent SIB. Researchers and clinicians often differentiate SIB into two categories (Nock, 2010). In the first category, there is no explicit intent to die, and therefore it is considered nonsuicidal SIB. In the second category, there is no clear lack of suicidal intent, and therefore it is considered suicidal SIB. Thus, ambivalent SIB is often categorized as suicidal SIB, rather than as a unique experience. Regardless of how ambivalent SIB is classified, it is likely that both intrapersonal and interpersonal factors relate to ambivalent SIB given that both are relevant to suicidal and nonsuicidal SIB. Furthermore, individuals who engage in SIB often report multiple intents behind their past SIB (Andover et al., 2012; Klonsky & Olino, 2008). Because of these similarities and the clinical significance of each, we examined intrapersonal (i.e., psychological distress) and interpersonal (i.e., social functioning) predictors of SIB in the current study.
Predicting SIB With Psychosocial Functioning
The relations between psychological distress and SIB are well established in the literature. Researchers have found positive associations between SIB and depression (Andover et al., 2005; Kirkcaldy et al., 2007), anxiety (Andover et al., 2005; Klonsky & Olino, 2008), obsessive-compulsion (Kirkcaldy et al., 2007), and interpersonal sensitivity (Kim et al., 2015; Kirkcaldy et al., 2007). These studies and others examined specific experiences of psychological distress as it relates to SIB in adults and adolescents and in community and inpatient samples.
Previous studies have also demonstrated relations between social functioning and SIB. For instance, SIB is associated with less social support from family and friends (Rotolone & Martin, 2012; Tuisku et al., 2014). Similarly, SIB is related to more negative interactions or negative relational dynamics with family (Halstead et al., 2014; Van Orden et al., 2010) and friends (Adrian et al., 2011).
Predicting SIB in Different Populations
Some individuals may be at greater risk for developing SIB. In particular, SIB is especially prevalent in individuals with borderline personality disorder (BPD). According to the American Psychiatric Association (2013), BPD is characterized by “marked impulsivity” along with “a pervasive pattern of instability of interpersonal relationships, self-image, and affects” (p. 663). Notably, one diagnostic criterion of BPD is “recurrent suicidal behavior, gestures, threats, or self-mutilating behavior” (p. 663). Additionally, some risk factors for developing BPD (e.g., high emotion dysregulation, trauma exposure, etc.; Crowell et al., 2009) are also risk factors for engaging in SIB (Nock, 2009, 2010). Although lifetime rates of SIB in individuals with BPD vary, one study found that 92.2% of individuals who sought outpatient treatment for symptoms of BPD had engaged in nonsuicidal SIB within the past 2 months (Andión et al., 2012). Additionally, up to 75% of individuals with BPD reported at least one instance of suicidal SIB (Black et al., 2004). Furthermore, there appear to be differences in SIB engagement when comparing individuals with BPD to a community sample. For example, adults with BPD reported engaging in nonsuicidal SIB more recently and frequently, using more varied methods, and causing more physically severe injuries that require medical attention, compared to individuals without BPD who engaged in nonsuicidal SIB (Turner et al., 2015).
Although the rates and severity of SIB are higher in individuals with BPD than in the general population (Bentley et al., 2015), SIB is considered relatively common in other populations, including nonsuicidal SIB among college students (e.g., Whitlock et al., 2006, 2013). College students are thought to engage in SIB more than the general population (as suggested by Wilcox et al., 2012) with approximately 17%–41% of college students participating in nonsuicidal SIB (Whitlock et al., 2006) compared to 5.9% of adults in the general population (Klonsky, 2011). Most college students are also in the highest risk age group for nonsuicidal SIB (Rodham & Hawton, 2009), and suicide is the second leading cause of death during this period (18–25 years old; Centers for Disease Control and Prevention, 2017). Notably, college students and non–college students of the same age (i.e., 16–24 years old) do not appear to differ in rates of SIB (McManus & Gunnell, 2020).
Current Study
A wealth of research has identified important psychological and social factors that may be associated with the occurrence of SIB. However, it remains unclear how these factors intersect to predict SIB. Additionally, as Turner et al. (2015) suggested, most research on SIB has considered either individuals with BPD or nonclinical samples (e.g., college students) without considering potential differences in predictors between these populations.
The current study used a comprehensive psychosocial approach to examine psychological distress and social functioning in two samples: a high-risk, treatment-seeking sample of individuals with traits of BPD and a sample of college students. This allowed us to characterize how key factors may intersect in predicting SIB. Our objectives were to (a) examine SIB within and between the two populations, (b) evaluate which psychosocial factors predicted total lifetime SIB for both populations, and (c) determine whether the predictors of total lifetime SIB varied by population (i.e., test for an interaction between psychosocial predictors and sample).
Method
Participants and Procedure
This study included a sample of individuals with BPD traits and a college student sample. For both samples, our inclusion criteria required that participants have a history of SIB with at least one self-reported episode of SIB (i.e., SIB of any intent) in the past year. We required recent SIB so that the measures of current psychological and social functioning would be appropriate predictors, rather than examining current functioning with a retrospective report of SIB after several years.
Sample 1: Individuals With Traits of BPD
The first sample consisted of data from a larger study on dialectical behavior therapy (DBT) in teens and adults (Sitton et al., 2020). Participants sought treatment for BPD symptoms from community-based counselors, although not all participants had formal diagnoses of BPD. The counselors obtained informed consent from participants and collaborated with a local university for this larger IRB-approved study. Of the 62 participants in this larger study, 96.8% (n = 60) reported engaging in SIB in the past year and constituted the BPD-Tx sample.
BPD-Tx participants (n = 60) were mostly young adults (M = 23.53 years, SD = 6.85 years, range = 18–48 years old). Based on self-reports, there were 49 females (81.7%), eight males (13.3%), and three participants who identified as non-binary or androgynous (5%). This sample was mostly White/European American (83.1%), followed by multiracial (10.2%), Asian American (1.7%), and Hispanic/Latinx (1.7%), with an additional 3.4% identifying as “other” or not reporting. Most (80%) reported no counseling experience prior to receiving DBT from the community counselors (i.e., at the time of recruitment). Data on sexual orientation was not available for this sample.
Sample 2: Undergraduate College Students
The second sample consisted of undergraduate students in introductory psychology courses at a university in the Pacific Northwest. We recruited students to participate in a study called “Emotional and Behavioral Responses to Stress” and informed all participants that they might experience distress as part of the study. After giving their informed consent, participants completed the measures online in a campus computer lab so any questions or concerns could be immediately addressed by a research assistant trained in suicide prevention. Debriefing included an extensive form that included on- and off-campus mental health resources.
Of the 536 students who completed the survey, 43.8% reported engaging in SIB during their lifetime, and 116 students (21.6%) met the inclusion criteria of engaging in SIB in the past year. This proportion of students is high compared to some student samples (e.g., Whitlock et al., 2006; Wilcox et al., 2012), but it is comparable to the lifetime rate from at least one other university sample (Gratz et al., 2002).
Student participants included in this study (n = 116) were mostly young adults (M = 19.62 years, SD = 1.58 years, range = 18–27 years old). Based on self-reports, there were 89 females (78.4%), 23 males (19.8%), and four participants who identified as non-binary or androgynous (4%). This sample was mostly White/European American (69%), followed by multiracial (19.8%), Asian American (6%), and Hispanic/Latinx (4.3%). Participants’ sexual orientations were as follows: 60.3% heterosexual, 18.1% bisexual, 7.8% pansexual, 6.9% homosexual, 1.7% asexual, and 1.8% who identified as “other.” Most (77.6%) reported previous counseling experiences, with about one-fifth currently seeing a counselor (22.4%). Other studies have found rates of prior experience with counseling services to be closer to 55% in college students (e.g., Niegocki & Ægisdóttir, 2019). Most student participants reported seeking counseling services for stress- and mood-related symptoms, and none reported seeking treatment specifically for BPD.
Measures
Self-Injurious Behavior (SIB)
We used the Lifetime Suicide Attempt Self-Injury Interview (LSASI; Linehan & Comtois, 1996) to assess participants’ history of SIB, including frequency, method, and intent. This 20-item measure asks about the dates of the most recent and most severe SIB act (e.g., “When was the most recent time that you intentionally injured yourself?”) and assesses the total lifetime frequency for 11 methods of SIB, as well as the separate intent(s) of each SIB act (suicidal, nonsuicidal, or ambivalent). Higher scores indicate more SIB acts.
Internal consistency was adequate for both samples (BPD-Tx sample, Cronbach’s α = .75; student sample, Cronbach’s α = .73). Notably, the LSASI was created for clinical use rather than research use; therefore, there are no known studies of its reliability or validity. However, the LSASI was already in use by the counselors in the larger study of DBT described, and they chose to use it to assess SIB in the BPD-Tx sample. We used it for the student sample to be consistent with the existing sample data. Following Linehan and Comtois’s (1996) scoring instructions, we calculated a total lifetime frequency for each participant by summing all SIB of any intent.
Psychological Distress
The Symptom Checklist-90-Revised (SCL-90-R; Derogatis, 1975) is a broad-spectrum psychiatric symptom checklist. Participants rate their distress level in the past week on a Likert-type scale from 0 (not at all) to 4 (extremely) for each of 90 items (e.g., “How much were you distressed by feeling critical of others?”). This measure assesses nine factors of psychological distress. For this study, we were interested in the factors of Depression, Anxiety, Obsessive-Compulsion, and Interpersonal Sensitivity. The internal consistency of this measure was very high in the BPD-Tx sample (α = .97).
To reduce participant burden, we used the Brief Symptom Inventory (BSI; Derogatis & Spencer, 1982), a 53-item version of the SCL-90-R, for student participants. The internal consistency was very high in the student sample (α = .96).
To assess the comparability of the SCL-90-R and the BSI for subsequent analysis, we separately averaged all items for the factors of Anxiety, Depression, Obsessive-Compulsion, and Interpersonal Sensitivity to determine BPD-Tx participants’ scores of psychological distress using these two measures. We found strong correlations between the SCL-90-R factors and the BSI factors (Depression: r = .92, p < .001; Anxiety: r = .97, p < .001; Obsessive-Compulsion: r = .95, p < .001; Interpersonal Sensitivity: r = .90, p < .001; and Average Psychological Distress: r = .98, p < .001). Following Derogatis (1993), who found no significant difference in validity between the SCL-90-R and the BSI, we used only the BSI items to create symptom factors for both samples. The internal consistency of the BSI items for the
BPD-Tx sample was very high (α = .95).
Social Functioning
The Network of Relationships Inventory-Behavioral Systems Version (NRI; Furman & Buhrmester, 2009) is a 33-item self-report measure of social support and negative interactions in various relationships (i.e., one’s mother, father, friends, and romantic partner). Participants rate the frequency of positive support or negative interactions on a Likert-scale from 1 (little or none) to 5 (the most). The Positive Support scale includes five subscales: Seeks Secure Base, Provides Secure Base, Seeks Safe Haven, Provides Safe Haven, and Companionship. The Negative Interactions scale includes three subscales: Conflict, Antagonism, and Criticism. Higher scores indicate more of each factor. The internal consistency was high for both samples (BPD-Tx, α = .93; student sample, α = .94).
We calculated the mean score of the Positive Support subscales, including Seeks Secure Base, Seeks Safe Haven, and Companionship. We did not include Provides Secure Base or Provides Safe Haven because Furman and Buhrmester (2009) described these as “caretaking” factors rather than “attachment” or “affiliation” factors. We also calculated the mean score of all three Negative Interactions subscales.
Data Analysis Plan
To begin, we tested for the assumptions of analysis, following guidelines proposed by Tabachnick and Fidell (2019). We defined outliers as data points beyond three standard deviations from the mean. We evaluated outliers within each group and replaced them with the value that was three standard deviations above the group mean. We chose this more liberal approach to outliers to maximize variability in the data. It was especially important to maintain variability in the outcome variable of total SIB given that higher levels of SIB have great clinical significance. For skewness and kurtosis of the composite variables, we used ±2 as our acceptable range of values. We transformed variables that did not meet our criteria for normality. We also utilized the missing completely at random test and found no systematic patterns to missing data, and thus used the group means to replace missing values for analysis.
To assess SIB in the two samples, we examined the intent of SIB acts separately for each sample and analyzed if SIB rates differed based on demographic information. To examine psychosocial predictors of SIB, we conducted a multiple linear regression. We used total SIB (including suicidal, nonsuicidal, and ambivalent SIB) as the outcome variable. We also examined differences in predictors of total SIB between the BPD-Tx and student samples by including interaction terms (e.g., psychological distress x sample). Statistically significant interactions were graphed to aid interpretation (Howell, 2013).
For the multiple linear regression analysis, we used effect coding for sample type (Daly et al., 2016), which allows comparison of a sample mean to the overall mean instead of using one sample as a reference group. Additionally, we centered the predictor variables around the grand mean for the whole sample to reduce the risk of multicollinearity. We inspected the tolerance and variance inflation factors, and used multiple sources (e.g., correlations between variables, p-values, and the standard error of the regression coefficients) to determine if multicollinearity was an issue.
Results
We used SPSS 24.0 to analyze the data. Using one-way analysis of variance (ANOVA), we found no differences between the samples based on gender or ethnicity (all p values > .05). However, using an independent samples t-test, we found that the BPD-Tx sample (M = 23.53, SD = 6.85) was older on average than the student sample: M = 19.62, SD = 1.58, t(173) = 5.85, p < .001. Additionally, the BPD-Tx sample (13.3%) reported prior experience with counseling (dichotomous variable) less often than the student sample (77.6%) on average: χ2(1) = 59.39, p < .001.
Sample Differences in SIB
We conducted descriptive analyses for all SIB variables. See Table 1 for descriptive statistics of the different intents of SIB (nonsuicidal, ambivalent, and suicidal), total SIB (including the untransformed total score), and the reported number of SIB methods. Table 1 also includes difference scores of SIB acts based on independent sample t-tests in consideration of the two samples. Individuals in the BPD-Tx sample engaged in more nonsuicidal, ambivalent, and total SIB in their lifetime compared to the student sample. Although there appeared to be no difference between samples in suicidal SIB, it is worth noting that this variable did not meet our criteria for normality in either sample even after transformation.
Table 1
Means (With Standard Deviations) and Difference Scores for Self-Injurious Behavior (SIB) by Sample
Variable |
BPD-Tx
(N = 60) |
Student
(N = 116) |
t(df) |
p |
Nonsuicidal SIB |
3.13 (1.81) |
2.34 (1.55) |
t(174) = 3.01 |
.003 |
Ambivalent SIB |
1.92 (2.02) |
1.07 (1.33) |
t(86.25) = 2.94 |
.004 |
Suicidal SIB |
0.66 (0.90) |
0.45 (0.81) |
t(174) = 1.61 |
.110 |
Total SIB |
3.87 (1.84) |
2.86 (1.43) |
t(96.56) = 3.73 |
< .001 |
Total SIB (untransformed) |
166.31 (268.69) |
44.10 (75.60) |
t(63.88) = 3.45 |
.001 |
Number of SIB methods |
3.28 (1.53) |
3.28 (2.11) |
t(174) = -0.004 |
.997 |
Note. BPD-Tx = participants with traits of borderline personality disorder; Total SIB (untransformed) =
untransformed values after adjusting the outliers in the raw reported values. Significant p values are in bold.
Although the normality of suicidal SIB was improved using a transformation, we were unable to meet our
acceptable range of ±2 for kurtosis (BPD-Tx kurtosis = 4.22; student kurtosis = 2.71).
In the BPD-Tx sample, we found no differences in SIB frequency based on gender, age, ethnicity, or counseling experience using one-way ANOVA. In the student sample, we found no differences in SIB frequency based on age, ethnicity, living situation, or counseling experience using one-way ANOVA. However, SIB frequency differed by gender such that those who identified as non-binary (M = 4.64, SD = 1.35) reported significantly higher rates of SIB than both males (M = 2.80, SD = 1.31) and females (M = 2.95, SD = 1.20). There were no differences in SIB frequency or severity based on sexual orientation in the student sample.
Psychosocial Predictors of SIB
We compared the two samples on the predictor variables first by using independent sample t-tests. We found that BPD-Tx participants reported less psychological distress (M = 2.21, SD = 0.78) than student participants: M = 2.78, SD = 0.89, t(174) = −4.16, p < .001. The BPD-Tx participants (M = 3.25, SD = 0.49) also reported less positive social support than student participants: M = 3.44, SD = 0.54, t(174) = −2.26, p = .025. Lastly, BPD-Tx participants (M = 1.22, SD = 0.43) reported more negative interactions than student participants: M = 1.07, SD = 0.43, t(174) = 2.15, p = .033.
We conducted bivariate correlations between all predictor variables and the outcome variable for each sample. In the BPD-Tx sample, total SIB was positively correlated with average psychological distress (r = .37, p = .004). In the student sample, total SIB was negatively correlated with positive social support (r = −.18, p = .049). In both samples, average psychological distress was positively associated with negative interactions (BPD-Tx: r = .36, p = .005; student: r = .24, p = .008). No other variables were significantly correlated in either sample.
Next, we conducted a multiple linear regression using total SIB as the outcome variable for both samples together. We entered seven predictors simultaneously: psychological distress, positive social support, negative interactions, sample type, and the interactions between sample type and the three other predictors. Together, these seven variables significantly predicted total SIB: F(7,168) = 5.01, p < .001, MSE = 2.33, r2 = .17. As shown in Table 2, psychological distress (sr2 = .06), sample type (sr2 = .12), and the interaction between psychological distress and sample type (sr2 = .03) were each significant unique predictors of total SIB. Specifically, based on the positive β weights, more psychological distress and being in the BPD-Tx sample were both associated with higher lifetime rates of SIB. Notably, multicollinearity did not appear to be an issue in this regression given the moderate to low correlations between factors, sufficiently high tolerance values, acceptable variance inflation factor values (ranging from 1.25–1.55), and the low standard error of regression coefficients relative to their scale.
Table 2
Multiple Regression Analysis Predicting Total Self-Injurious Behavior for the Whole Sample (N = 176)
|
|
CI |
Variable |
B |
SE B |
β |
t |
p |
sr2 |
Lower |
Upper |
Psych. distress |
0.57 |
0.16 |
.31 |
3.55 |
.001 |
.06 |
0.25 |
0.89 |
Pos. social support |
−0.48 |
0.25 |
−.16 |
−1.96 |
.052 |
.02 |
−0.97 |
0.00 |
Neg. interactions |
−0.26 |
0.30 |
−.07 |
−0.85 |
.399 |
.003 |
−0.85 |
0.34 |
Sample type |
0.68 |
0.14 |
.39 |
4.87 |
< .001 |
.12 |
0.40 |
0.95 |
Psych. distress x sample |
0.40 |
0.16 |
.21 |
2.46 |
.015 |
.03 |
0.08 |
0.71 |
Pos. social support x sample |
0.00 |
0.25 |
.00 |
0.00 |
.997 |
.001 |
−0.49 |
0.49 |
Neg. interactions x sample |
−0.08 |
0.30 |
−.02 |
−0.25 |
.801 |
.001 |
−0.67 |
0.52 |
|
|
|
|
|
|
|
|
|
|
Note. Psych. = psychological; Pos. = positive; Neg. = negative; sr2 = squared semipartial correlation. Sample type was
coded so that BPD-Tx sample = 1, student sample = -1. Significant p values are in bold.
Sample Differences in SIB Predictors
To further probe the statistically significant interaction, we plotted the regression paths for psychological distress predicting total SIB by sample type. As shown in Figure 1, more psychological distress was related to higher lifetime rates of total SIB in both samples, which supports the main effect of psychological distress found in the multiple regression analysis. However, the relation between psychological distress and total SIB was stronger in the BPD-Tx sample than in the student sample (as evidenced by the steeper slope of the regression line representing the BPD-Tx sample compared to that of the student sample).
Figure 1
Regression Lines of Average Psychological Distress Predicting Total Self-Injurious Behavior (SIB) by Sample Type
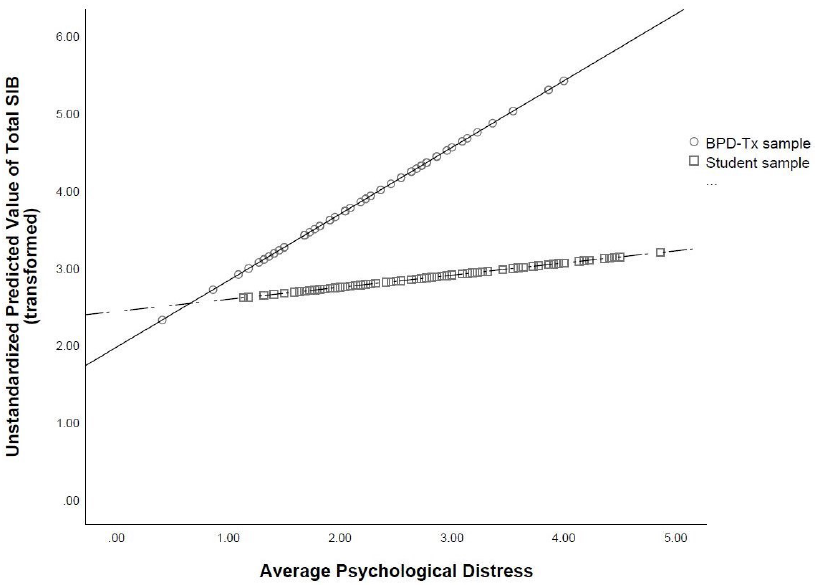
Discussion
The primary goals of the current study were to establish a more comprehensive set of predictors of SIB and to better understand how the experience of SIB varied by population (BPD-Tx vs. college students). This study was unique in its psychosocial approach to predictors. Additionally, we tested for interactions between sample type and the psychosocial predictors of SIB. This singular examination of interacting predictors has seldom been conducted in the literature, and thus is an important strength of this study.
SIB Engagement and Psychosocial Functioning
The results demonstrate a very high lifetime frequency of SIB in both samples. Although most studies do not report the lifetime frequency rates of SIB of their participants, the frequency of SIB in our student sample was comparable to that found in another study of students using the same SIB methods with nonsuicidal intent (Croyle & Waltz, 2007). The frequency rate of SIB in the BPD-Tx sample appeared to be lower than found in some other studies with individuals with BPD (e.g., Turner et al., 2015).
Additionally, we found that the lifetime frequency rates of SIB were higher in the BPD-Tx sample than in the student sample, which aligns with previous studies (e.g., Klonsky & Olino, 2008; Turner et al., 2015). This makes sense given the maladaptive behaviors often seen in individuals with BPD. Additionally, given that the BPD-Tx sample was older than student participants on average, it is also possible that their increased lifetime rates of SIB reflected a greater number of years to engage in it. Alternatively, the higher SIB frequency reported by the BPD-Tx participants may serve an interpersonal function. According to Linehan (1993), nonsuicidal SIB is commonly used by individuals with BPD to communicate with and gain attention from others.
Interestingly, despite higher rates of total SIB, BPD-Tx participants reported less psychological distress than did student participants. This was contrary to many other studies showing a strong association between psychological distress and engagement in nonsuicidal SIB for individuals with BPD (e.g., Sadeh et al., 2014; Turner et al., 2015). One possible explanation for the lower rates of psychological distress reported by BPD-Tx participants is that their baseline level of psychological distress was higher, leading these negative emotions to be considered normal and therefore not “distressing.” Additionally, given that fewer BPD-Tx participants reported prior experience with counseling than student participants, it could be that BPD-Tx participants reported less psychological distress because of a lack of emotional self-awareness. This aligns with Turner et al.’s (2015) finding that participants with BPD who engage in nonsuicidal SIB reported less awareness of their emotional states. Another explanation is that the BPD-Tx participants were recruited from a community-based clinic wherein they were preparing to start DBT. Although the data used in the current study represents pretest data gathered prior to treatment, it is possible that the BPD-Tx participants were experiencing lowered distress at the time of data collection because of the hope and positive expectations that are often associated with starting a new treatment (Dew & Bickman, 2005).
Socially, the BPD-Tx participants reported less positive support than student participants. This finding aligns with the biosocial theory of BPD (Linehan, 1993), which suggests that individuals with BPD may experience or perceive an invalidating environment. Alternatively, BPD-Tx participants may be more likely to interpret interactions with others as negative, which aligns with Peters et al.’s (2015) finding that individuals with traits of BPD often demonstrated maladaptive responses to emotional experiences, leading them to interact negatively with others.
Psychosocial Predictors of SIB
An important finding of the current study is that psychological distress predicted total SIB with a small to moderate effect size. This suggests that psychological distress (including experiences of anxiety, depression, obsessive-compulsion, and interpersonal sensitivity) is an important component of SIB of various intents. Specifically, psychological distress may act as a catalyst for SIB, wherein individuals engage in SIB to decrease their psychological distress. This explanation aligns with Nock and Prinstein’s (2004, 2005) theory of the intrapersonal negative reinforcement function of nonsuicidal SIB. Namely, that one might engage in SIB in order to reduce tension or psychological distress, particularly anxiety.
Contrary to the majority of extant literature (e.g., Wilcox et al., 2012), neither positive social support nor negative interactions predicted total SIB in the current study. We also did not find an interaction between either social variable and sample type, suggesting that social functioning might not be a direct, distinct predictor of total SIB for either population. However, it is possible that social functioning is indirectly related to total SIB. For example, we found a significant positive correlation between negative interactions and psychological distress in both samples. Given these correlations, negative interactions may contribute to experiences of psychological distress, which then predict total SIB. This proposed indirect relation is supported by Adrian et al.’s (2011) study, which found that emotion dysregulation partially mediated the relation between interpersonal problems (i.e., problems with one’s family and peers) and nonsuicidal SIB.
Another possible explanation for the lack of significant social predictors of SIB in the current study is the variability in the data that stems from inconsistent timing of social support. Specifically, it is unclear if positive support preceded SIB engagement, followed the SIB act, or both. Turner et al. (2016) found that perceived social support increased after participants disclosed their nonsuicidal SIB acts to others. However, they also found that this increased support was associated with increased nonsuicidal SIB urges and acts the following day, presumably because the SIB had achieved the desired interpersonal function. Thus, similar to Turner et al.’s (2016) study, the lack of a clear, linear relation between SIB and social support may have contributed to nonsignificant findings of social predictors in the current study.
Notably, the strongest single predictor of total SIB was sample type, with BPD-Tx participants showing greater frequency of total lifetime SIB than student participants. This aligns with Turner et al. (2015), who found that individuals with BPD traits engage in nonsuicidal SIB more often than do those without BPD traits.
Sample Differences in SIB Predictors
The relation between psychological distress and total SIB was stronger for the BPD-Tx sample than for the student sample. This finding is somewhat supported by previous literature; for example, Klonsky and Olino’s (2008) latent class analysis revealed that the group with the most nonsuicidal SIB also reported more symptoms of BPD and psychological distress and reported regularly engaging in nonsuicidal SIB to help regulate their emotions. In comparison, individuals with BPD traits in the current study reported engaging in more total SIB (as well as nonsuicidal SIB) but did not report greater levels of psychological distress than did the student participants. However, if our BPD-Tx participants used SIB for emotion regulation, too, then perhaps this strategy allowed them to experience lower levels of psychological distress day-to-day than student participants. This aligns with Sadeh et al.’s (2014) finding that BPD symptoms related to the affect-regulating function of SIB, especially nonsuicidal SIB.
Additionally, the significant interaction we found between psychological distress and sample type resembles Andover et al.’s (2005) finding that BPD symptoms accounted for the relation between anxiety and nonsuicidal SIB. However, in our study, psychological distress was a significant unique predictor of total SIB (in addition to the significant interaction between psychological distress and sample type). In other words, sample type seems to be a moderator between psychological distress and SIB in our study, as opposed to a mediator.
Counseling Implications
Our findings have several treatment implications. Many counselors will not be surprised by the high rates of SIB found in our BPD-Tx sample. However, we also found a clinically important high rate of SIB in college students. Given that past engagement in SIB is one of the strongest predictors of future SIB (including nonsuicidal and suicidal SIB; Tuisku et al., 2014), the high lifetime rates of SIB found in both samples in the current study are noteworthy for service providers. Specifically, our results suggest that universities and other institutions concerned with mental health in college students should consider utilizing SIB screening tools. Additionally, the high prevalence of students with a lifetime history of any SIB suggests the need for widespread intervention programs for student populations. For example, some research (e.g., Kannan et al., 2021) has examined the implementation of DBT skills groups in college counseling centers for students with a variety of presenting issues, including SIB. Such intervention programs could benefit a wider range of students and help improve quality of life for many, especially those struggling with SIB.
Given that psychological distress predicted total SIB, it may be beneficial for counselors to regularly assess the level of psychological distress in all clients, including those with BPD and college students. Clients with high psychological distress, including anxiety, depression, obsessive-compulsion, and interpersonal sensitivity, will likely engage in more SIB than those with low psychological distress, and thus the counselor may be able to intervene before the client escalates to a high frequency of SIB. Assessing and tracking affective distress levels may be common with suicide assessment and safety planning, but there may be less awareness about the need for this with SIB. Treatment protocols could also focus on lowering psychological distress to see if that will decrease SIB. For example, DBT, which emphasizes psychological distress tolerance, has been increasingly implemented in college campus counseling centers (see Chugani, 2015). However, given that the current study’s findings are not causal, we cannot definitively conclude that lowering psychological distress will affect SIB.
Importantly, the interaction between psychological distress and sample type is noteworthy given that it contributes to the small extant evidence of divergence between populations of individuals with symptoms of BPD and other, more community-based populations like college students. Specifically, we found differences in SIB prevalence, in lifetime frequency, and in one predictor (i.e., psychological distress) in our two samples. This aligns with Turner et al.’s (2015) findings that individuals who engaged in SIB with and without BPD differed in SIB frequency, severity, and comorbid affective symptomology.
It is also worth noting that the correlational analysis revealed a difference between these two samples in social functioning. In particular, there was a statistically significant negative correlation between total SIB and positive social support in the student sample, but not in the BPD-Tx sample. Because of this, although we only found one statistically significant interaction between psychosocial predictors and sample type, it is plausible that there are other notable differences in SIB risk factors between these two populations. Thus, when treating SIB, it may be worth assessing for other symptoms of BPD to form a more accurate representation of a client’s experience and to help form a specific treatment plan.
Limitations and Future Studies
One potential limitation of the current study is that we included only individuals who reported engaging in SIB in the past year because we wanted to examine current predictors of current SIB. However, it is possible that psychological distress and social support are more effective predictors of future SIB acts. In other words, the current study examined predictors of the frequency of SIB using current psychosocial functioning, yet the psychosocial variables might have been better at predicting whether or not an individual will engage in SIB in the future. This theory aligns with Heath et al.’s (2009) interpretation of their lack of results linking social support to lifetime rates of nonsuicidal SIB. Specifically, that social support may better relate to differences between those who will engage in SIB compared to those who will not, as opposed to the degree (i.e., frequency) of SIB. It is unclear how the results may have differed if we included a comparison group of individuals who do not engage in SIB or have never engaged in SIB.
A second limitation was the need to use specific measures to compare the student sample to the existing BPD-Tx sample data. Although the LSASI measure has the advantage of thoroughly examining SIB methods and intent, it was intended for clinical use rather than research. Additionally, the LSASI is a lifetime measure of SIB as opposed to assessing recent SIB; although our inclusion criteria required participants to have engaged in SIB at least once in the past year, it is unknown how recent or severe the SIB was in the past year relative to one’s lifetime. Because of this, a dichotomous measure of past-year engagement in SIB may have better suited our need for recent SIB assessment. Nonetheless, the LSASI provided a great depth and variability in the data that was not only valuable in the current research study, but also clinically important to the counselors with whom we collaborated in the larger DBT study.
A third limitation is that there may be other variables involved in predicting SIB that were not assessed, such as emotion regulation skills or trauma exposure. For example, SIB frequency might be more strongly related to one’s ability to regulate distress rather than the presence of distress itself. Given that emotional reactivity and trauma exposure are both risk factors for SIB (Nock, 2009, 2010) and for the development of BPD (Crowell et al., 2009), future studies may want to further explicate these relations.
It is also worth noting that the samples in the current study may include theoretically overlapping populations. Specifically, we did not screen the BPD-Tx group for current academic status, and therefore it is possible that some participants in the BPD-Tx group were also college students. We decided not to exclude BPD-Tx participants based on academic status in order to reduce barriers to study participation and so that the BPD-Tx sample would represent people who seek treatment for BPD in the community, not just those who are not college students. Additionally, although we screened the student sample for the explicit endorsement of BPD diagnosis, it is possible that some participants in the student sample had subthreshold symptoms of BPD (especially considering that SIB itself is a symptom of BPD) or simply had not received a diagnosis of BPD at the time of this study.
Future studies should continue to examine psychosocial predictors of SIB with larger and more diverse samples in order to explore the relations between psychological and social predictors. Additionally, future studies should explore other relevant factors with the psychosocial predictors (e.g., emotion regulation, trauma exposure) to determine if other factors may better explain (or mediate the relations with) SIB. Moreover, longitudinal and experience-sampling designs would allow researchers to gain better understanding of how changes in psychosocial functioning relate to decisions to engage in SIB as well as the exact sequence of events for SIB acts. Although some studies have recently begun using these techniques, a more psychosocial approach to predictors and consequences of SIB (also considering various intents) may provide more prudent information for intervention and treatment of individuals who engage in SIB.
Conclusion
The current study sought to identify psychosocial predictors of SIB in two clinically different populations and to compare predictors between these populations. We found high lifetime frequency rates of SIB in both samples, suggesting a need for more widespread assessment of SIB in young adults from different populations. We also found that population type itself was the strongest predictor of SIB—individuals with traits of BPD engaged in more SIB in their lifetimes than did college students. Additionally, psychological distress predicted SIB; however, we also found a significant interaction between population and psychological distress, which suggests that psychological distress may be more related to SIB in individuals with traits of BPD than in more community-based populations like college students. Consequently, counselors should consider population and psychological distress when assessing SIB risk in clients.
Conflict of Interest and Funding Disclosure
Data from the existing BPD sample was partially
funded by an internal grant awarded to coauthor
Christina Byrne. The authors reported no conflict
of interest or other funding for the development
of this manuscript.
References
Adrian, M., Zeman, J., Erdley, C., Lisa, L., & Sim, L. (2011). Emotional dysregulation and interpersonal difficulties as risk factors for nonsuicidal self-injury in adolescent girls. Journal of Abnormal Child Psychology, 39(3), 389–400. https://doi.org/10.1007/s10802-010-9465-3
American Psychiatric Association. (2013). Diagnostic and statistical manual of mental disorders (5th ed.). https://doi.org/10.1176/appi.books.9780890425596
Andión, Ó., Ferrer, M., Matali, J., Gancedo, B., Calvo, N., Barral, C., Valero, S., Di Genova, A., Diener, M. J., Torrubia, R., & Casas, M. (2012). Effectiveness of combined individual and group dialectical behavior therapy compared to only individual dialectical behavior therapy: A preliminary study. Psychotherapy, 49(2), 241–250. https://doi.org/10.1037/a0027401
Andover, M. S., Morris, B. W., Wren, A., & Bruzzese, M. E. (2012). The co-occurrence of non-suicidal self-injury and attempted suicide among adolescents: Distinguishing risk factors and psychosocial correlates. Child and Adolescent Psychiatry and Mental Health, 6. https://doi.org/10.1186/1753-2000-6-11
Andover, M. S., Pepper, C. M., Ryabchenko, K. A., Orrico, E. G., & Gibb, B. E. (2005). Self-mutilation and symptoms of depression, anxiety, and borderline personality disorder. Suicide and Life-Threatening Behavior, 35(5), 581–591. https://doi.org/10.1521/suli.2005.35.5.581
Andrews, T., Martin, G., Hasking, P., & Page, A. (2013). Predictors of continuation and cessation of nonsuicidal self-injury. Journal of Adolescent Health, 53(1), 40–46. https://doi.org/10.1016/j.jadohealth.2013.01.009
Bentley, K. H., Cassiello-Robbins, C. F., Vittorio, L., Sauer-Zavala, S., & Barlow, D. H. (2015). The association between nonsuicidal self-injury and the emotional disorders: A meta-analytic review. Clinical Psychology Review, 37, 72–88. https://doi.org/10.1016/j.cpr.2015.02.006
Black, D. W., Blum, N., Pfohl, B., & Hale, N. (2004). Suicidal behavior in borderline personality disorder: Prevalence, risk factors, prediction, and prevention. Journal of Personality Disorders, 18(3), 226–239. https://doi.org/10.1521/pedi.18.3.226.35445
Centers for Disease Control and Prevention. (2017). Leading causes of death reports. https://webappa.cdc.gov/sasweb/ncipc/leadcause.html
Chugani, C. D. (2015). Dialectical behavior therapy in college counseling centers: Current literature and implications for practice. Journal of College Student Psychotherapy, 29(2), 120–131.
https://doi.org/10.1080/87568225.2015.1008368
Crowell, S. E., Beauchaine, T. P., & Linehan, M. M. (2009). A biosocial developmental model of borderline personality: Elaborating and extending Linehan’s theory. Psychological Bulletin, 135(3), 495–510. https://doi.org/10.1037/a0015616
Croyle, K. L., & Waltz, J. (2007). Subclinical self-harm: Range of behaviors, extent, and associated characteristics. American Journal of Orthopsychiatry, 77(2), 332–342. https://doi.org/10.1037/0002-9432.77.2.332
Daly, A., Dekker, T., & Hess, S. (2016). Dummy coding vs effects coding for categorical variables: Clarifications and extensions. Journal of Choice Modelling, 21, 36–41. https://doi.org/10.1016/j.jocm.2016.09.005
Derogatis, L. R. (1975). The SCL-90-R. Clinical Psychometric Research.
Derogatis, L. R. (1993). BSI Brief Symptom Inventory: Administration, Scoring, and Procedure Manual (4th ed.). National Computer Systems.
Derogatis, L. R., & Spencer, P. (1982). The Brief Symptom Inventory: Administration, scoring and procedures manual—1. Clinical Psychometric Research.
Dew, S. E., & Bickman, L. (2005). Client expectancies about therapy. Mental Health Services Research, 7(1), 21–33. https://doi.org/10.1007/s11020-005-1963-5
Furman, W., & Buhrmester, D. (2009). Methods and measures: The Network of Relationships Inventory: Behavioral Systems Version. International Journal of Behavioral Development, 33(5), 470–478. https://doi.org/10.1177/0165025409342634
Gratz, K. L., Conrad, S. D., & Roemer, L. (2002). Risk factors for deliberate self-harm among college students. American Journal of Orthopsychiatry, 72(1), 128–140. https://doi.org/10.1037/0002-9432.72.1.128
Halstead, R. O., Pavkov, T. W., Hecker, L. L., & Seliner, M. M. (2014). Family dynamics and self-injury behaviors: A correlation analysis. Journal of Marital and Family Therapy, 40(2), 246–259.
https://doi.org/10.1111/j.1752-0606.2012.00336.x
Heath, N. L., Ross, S., Toste, J. R., Charlebois, A., & Nedecheva, T. (2009). Retrospective analysis of social factors and nonsuicidal self-injury among young adults. Canadian Journal of Behavioural Science/Revue Canadienne des sciences du comportement, 41(3), 180–186. https://doi.org/10.1037/a0015732
Howell, D. C. (2013). Statistical methods for psychology (8th ed.). Cengage.
Joiner, T. (2005). Why people die by suicide. Harvard University Press.
Kannan, D., Chugani, C. D., Muhomba, M., & Koon, K. (2021). A qualitative analysis of college counseling center staff experiences of the utility of dialectical behavior therapy programs on campus. Journal of College Student Pychotherapy, 35(1), 1–17. https://doi.org/10.1080/87568225.2019.1620662
Kim, K. L., Cushman, G. K., Weissman, A. B., Puzia, M. E., Wegbreit, E., Tone, E. B., Spirito, A., & Dickstein, D. P. (2015). Behavioral and emotional responses to interpersonal stress: A comparison of adolescents engaged in non-suicidal self-injury to adolescent suicide attempters. Psychiatry Research, 228(3), 899–906.
https://doi.org/10.1016/j.psychres.2015.05.001
Kirkcaldy, B. D., Brown, J. M., & Siefen, G. R. (2007). Profiling adolescents attempting suicide and self-injurious behavior. International Journal on Disability and Human Development, 6(1), 75–86. https://doi.org/10.1515/IJDHD.2007.6.1.75
Klonsky, E. D. (2011). Non-suicidal self-injury in United States adults: Prevalence, sociodemographics, topography and functions. Psychological Medicine, 41(9), 1981–1986. https://doi.org/10.1017/S0033291710002497
Klonsky, E. D., & Olino, T. M. (2008). Identifying clinically distinct subgroups of self-injurers among young adults: A latent class analysis. Journal of Consulting and Clinical Psychology, 76(1), 22–27. https://doi.org/10.1037/0022-006X.76.1.22
Linehan, M. M. (1993). Cognitive-behavioral treatment of borderline personality disorder. Guilford.
Linehan, M. M., & Comtois, K. A. (1996). Lifetime parasuicide count. Unpublished manuscript, Department of Psychology, University of Washington, Seattle.
McManus, S., & Gunnell, D. (2020). Trends in mental health, non-suicidal self-harm and suicide attempts in 16–24-year-old students and non-students in England, 2000–2014. Social Psychiatry and Psychiatric Epidemiology, 55(1), 125–128. https://doi.org/10.1007/s00127-019-01797-5
Niegocki, K. L., & Ægisdóttir, S. (2019). College students’ coping and psychological help-seeking attitudes and intentions. Journal of Mental Health Counseling, 41(2), 144–157. https://doi.org/10.17744/mehc.41.2.04
Nock, M. K. (2009). Why do people hurt themselves? New insights into the nature and functions of self-injury. Current Directions in Psychological Science, 18(2), 78–83. https://doi.org/10.1111/j.1467-8721.2009.01613.x
Nock, M. K. (2010). Self-injury. Annual Review of Clinical Psychology, 6, 339–363.
https://doi.org/10.1146/annurev.clinpsy.121208.131258
Nock, M. K., & Prinstein, M. J. (2004). A functional approach to the assessment of self-mutilative behavior. Journal of Consulting and Clinical Psychology, 72(5), 885–890. https://doi.org/10.1037/0022-006X.72.5.885
Nock, M. K., & Prinstein, M. J. (2005). Contextual features and behavioral functions of self-mutilation among adolescents. Journal of Abnormal Psychology, 114(1), 140–146. https://doi.org/10.1037/0021-843X.114.1.140
Peters, J. R., Smart, L. M., & Baer, R. A. (2015). Dysfunctional responses to emotion mediate the cross-sectional relationship between rejection sensitivity and borderline personality features. Journal of Personality Disorders, 29(2), 231–240. https://doi.org/10.1521/pedi_2014_28_151
Rodham, K., & Hawton, K. (2009). Epidemiology and phenomenology of nonsuicidal self-injury. In M. K. Nock (Ed.), Understanding nonsuicidal self-injury: Origins, assessment, and treatment (pp. 37–62). American Psychological Association. https://doi.org/10.1037/11875-003
Rotolone, C., & Martin, G. (2012). Giving up self-injury: A comparison of everyday social and personal resources in past versus current self-injurers. Archives of Suicide Research, 16(2), 147–158.
https://doi.org/10.1080/13811118.2012.667333
Sadeh, N., Londahl-Shaller, E. A., Piatigorsky, A., Fordwood, S., Stuart, B. K., McNiel, D. E., Klonsky, E. D., Ozer, E. M., & Yaeger, A. M. (2014). Functions of non-suicidal self-injury in adolescents and young adults with borderline personality disorder symptoms. Psychiatry Research, 216(2), 217–222.
https://doi.org/10.1016/j.psychres.2014.02.018
Sitton, M., Du Rocher Schudlich, T., Byrne, C., Ochrach, C. M., & Erwin, S. E. A. (2020). Family functioning and self-injury in treatment-seeking adolescents: Implications for counselors. The Professional Counselor, 10(3), 351–364. https://doi.org/10.15241/ms.10.3.351
Tabachnick, B. G., & Fidell, L. S. (2019). Using multivariate statistics (7th ed.). Pearson.
Tuisku, V., Kiviruusu, O., Pelkonen, M., Karlsson, L., Strandholm, T., & Marttunen, M. (2014). Depressed adolescents as young adults: Predictors of suicide attempt and non-suicidal self-injury during an 8-year follow-up. Journal of Affective Disorders, 152, 313–319. https://doi.org/10.1016/j.jad.2013.09.031
Turner, B. J., Cobb, R. J., Gratz, K. L., & Chapman, A. L. (2016). The role of interpersonal conflict and perceived social support in nonsuicidal self-injury in daily life. Journal of Abnormal Psychology, 125(4), 588–598. https://doi.org/10.1037/abn0000141
Turner, B. J., Dixon-Gordon, K. L., Austin, S. B., Rodriguez, M. A., Rosenthal, M. Z., & Chapman, A. L. (2015). Non-suicidal self-injury with and without borderline personality disorder: Differences in self-injury and diagnostic comorbidity. Psychiatry Research, 230(1), 28–35. https://doi.org/10.1016/j.psychres.2015.07.058
Van Orden, K. A., Witte, T. K., Cukrowicz, K. C., Braithwaite, S. R., Selby, E. A., & Joiner, T. E., Jr. (2010). The interpersonal theory of suicide. Psychological Review, 117(2), 575–600. https://doi.org/10.1037/a0018697
Whitlock, J., Eckenrode, J., & Silverman, D. (2006). Self-injurious behaviors in a college population. Pediatrics, 117(6), 1939–1948. https://doi.org/10.1542/peds.2005-2543
Whitlock, J., Muehlenkamp, J., Eckenrode, J., Purington, A., Baral Abrams, G., Barreira, P., & Kress, V. (2013). Nonsuicidal self-injury as a gateway to suicide in young adults. Journal of Adolescent Health, 52(4), 486–492. https://doi.org/10.1016/j.jadohealth.2012.09.010
Wilcox, H. C., Arria, A. M., Caldeira, K. M., Vincent, K. B., Pinchevsky, G. M., & O’Grady, K. E. (2012). Longitudinal predictors of past-year non-suicidal self-injury and motives among college students. Psychological Medicine, 42(4), 717–726. https://doi.org/10.1017/S0033291711001814
Melissa J. Sitton, MS, is a doctoral student at Southern Methodist University. Tina Du Rocher Schudlich, PhD, MHP, is a professor at Western Washington University. Christina Byrne, PhD, is an associate professor at Western Washington University. Correspondence may be addressed to Melissa J. Sitton, Department of Psychology, Southern Methodist University, P.O. Box 750442, Dallas, TX 75275-0442, msitton@smu.edu.