Aug 20, 2021 | Volume 11 - Issue 3
Alison M. Boughn, Daniel A. DeCino
This article introduces the development and implementation of the Psychological Maltreatment Inventory (PMI) assessment with child respondents receiving services because of an open child abuse and/or neglect case in the Midwest (N = 166). Sixteen items were selected based on the literature, subject matter expert refinement, and readability assessments. Results indicate the PMI has high reliability (α = .91). There was no evidence the PMI total score was influenced by demographic characteristics. A positive relationship was discovered between PMI scores and general trauma symptom scores on the Trauma Symptom Checklist for Children Screening Form (TSCC-SF; r = .78, p = .01). Evidence from this study demonstrates the need to refine the PMI for continued use with children. Implications for future research include identification of psychological maltreatment in isolation, further testing and refinement of the PMI, and exploring the potential relationship between psychological maltreatment and suicidal ideation.
Keywords: psychological maltreatment, child abuse, neglect, assessment, trauma
In 2012, the Centers for Disease Control (CDC; 2012) reported that the total cost of child maltreatment (CM) in 2008, including psychological maltreatment (PM), was $124 billion. Fang et al. (2012) estimated the lifetime burden of CM in 2008 was as high as $585 billion. The CDC (2012) characterized CM as rivaling “other high profile public health problems” (para. 1). By 2015, the National Institutes of Health reported the total cost of CM, based on substantiated incidents, was reported to be $428 billion, a 345% increase in just 7 years; the true cost was predictably much higher (Peterson et al., 2018). Using the sensitivity analysis done by Fang et al. (2012), the lifetime burden of CM in 2015 may have been as high as $2 trillion. If these trends continue unabated, the United States could expect a total cost for CM, including PM, of $5.1 trillion by 2030, with a total lifetime cost of $24 trillion. More concerning, this increase would not account for any impact from the COVID-19 pandemic.
Mental health first responders and child protection professionals may encounter PM regularly in their careers (Klika & Conte, 2017; U.S. Department of Health and Human Services [DHHS], 2018). PM experiences are defined as inappropriate emotional and psychological acts (e.g., excessive yelling, threatening language or behavior) and/or lack of appropriate acts (e.g., saying I love you) used by perpetrators of abuse and neglect to gain organizational control of their victims (American Professional Society on the Abuse of Children [APSAC], 2019; Klika & Conte, 2017; Slep et al., 2015). Victims may experience negative societal perceptions (i.e., stigma), fear of retribution from caregivers or guardians, or misdiagnosis by professional helpers (Iwaniec, 2006; López et al., 2015). They often face adverse consequences that last their entire lifetime (Spinazzola et al., 2014; Tyrka et al., 2013; Vachon et al., 2015; van der Kolk, 2014; van Harmelen et al., 2010; Zimmerman & Mercy, 2010). PM can be difficult to identify because it leaves no readily visible trace of injury (e.g., bruises, cuts, or broken bones), making it complicated to substantiate that a crime has occurred (Ahern et al., 2014; López et al., 2015). Retrospective data outlines evaluation processes for PM identification in adulthood; however, childhood PM lacks a single definition and remains difficult to assess (Tonmyr et al., 2011). These complexities in identifying PM in children may prevent mental health professionals from intervening early, providing crucial care, and referring victims for psychological health services (Marshall, 2012; Spinazzola et al., 2014). The Psychological Maltreatment Inventory (PMI) is the first instrument of its kind to address these deficits.
Child Psychological Maltreatment
Although broadly conceptualized, child PM experiences are described as literal acts, events, or experiences that create current or future symptoms that can affect a victim without immediate physical evidence (López et al., 2015). Others have extended child PM to include continued patterns of severe events that impede a child from securing basic psychological needs and convey to the child that they are worthless, flawed, or unwanted (APSAC, 2019). Unfortunately, these broad concepts lack the specificity to guide legal and mental health interventions (Ahern et al., 2014). Furthermore, legal definitions of child PM vary from jurisdiction to jurisdiction and state to state (Spinazzola et al., 2014). The lack of consistent definitions and quantifiable measures of child PM may create barriers for prosecutors and other helping professionals within the legal system as well as a limited understanding of PM in evidence-based research (American Psychiatric Association [APA], 2013; APSAC, 2019; Klika & Conte, 2017). These challenges are exacerbated by comorbidity with other forms of maltreatment.
Co-Occurring Forms of Maltreatment
According to DHHS (2018), child PM is rarely documented as occurring in isolation compared to other forms of maltreatment (i.e., physical abuse, sexual abuse, or neglect). Rather, researchers have found PM typically coexists with other forms of maltreatment (DHHS, 2018; Iwaniec, 2006; Marshall, 2012). Klika and Conte (2017) reported that perpetrators who use physical abuse, inappropriate language, and isolation facilitate conditions for PM to coexist with other forms of abuse. Van Harmelen et al. (2011) argued that neglectful acts constitute evidence of PM (e.g., seclusion; withholding medical attention; denying or limiting food, water, shelter, and other basic needs).
Consequences of PM Experienced in Childhood
Mills et al. (2013) and Greenfield and Marks (2010) noted PM experiences in early childhood might manifest in physical growth delays and require access to long-term care throughout a victim’s lifetime. Children who have experienced PM may suffer from behaviors that delay or prevent meeting developmental milestones, achieving academic success in school, engaging in healthy peer relationships, maintaining physical health and well-being, forming appropriate sexual relationships as adults, and enjoying satisfying daily living experiences (Glaser, 2002; Maguire et al., 2015). Neurological and cognitive effects of PM in childhood impact children as they transition into adulthood, including abnormalities in the amygdala and hippocampus (Tyrka at al., 2013). Brown et al. (2019) found that adults who reported experiences of CM had higher rates of negative responses to everyday stress, a larger constellation of unproductive coping skills, and earlier mortality rates (Brown et al., 2019; Felitti et al., 1998). Furthermore, adults with childhood PM experiences reported higher rates of substance abuse than those compared to control groups (Felitti et al., 1998).
Trauma-Related Symptomology. Researchers speculate that children exposed to maltreatment and crises, especially those that come without warning, are at greater risk for developing a host of trauma-related symptoms (Spinazzola et al., 2014). Developmentally, children lack the ability to process and contextualize their lived experiences. Van Harmelen et al. (2010) discovered that adults who experienced child PM had decreased prefrontal cortex mass compared to those without evidence of PM. Similarly, Field et al. (2017) found those unable to process traumatic events produced higher levels of stress hormones (i.e., cortisol, epinephrine, norepinephrine); these hormones are produced from the hypothalamic-pituitary-adrenal (HPA) and sympathetic-adrenal-medullary (SAM) regions in the brain. Some researchers speculate that elevated levels of certain hormones and hyperactive regions within the brain signal the body’s biological attempt to reduce the negative impact of PM through the fight-flight-freeze response (Porges, 2011; van der Kolk, 2014).
Purpose of Present Study
At the time of this research, there were few formal measures using child self-report to assess how children experience PM. We developed the PMI as an initial quantifiable measure of child PM for children and adolescents between the ages of 8 and 17, as modeled by Tonmyr and colleagues (2011). The PMI was developed in multiple stages, including 1) a review of the literature, 2) a content validity survey with subject matter experts (SMEs), 3) a pilot study (N = 21), and 4) a large sample study (N = 166). An additional instrument, the Trauma Symptom Checklist for Children Screening Form (TSCC-SF; Briere & Wherry, 2016), was utilized in conjunction with the PMI to explore occurrences of general trauma symptoms among respondents. The following four research questions were investigated:
- How do respondent demographics relate to PM?
- What is the rate of PM experience with respondents who are presently involved in an open CM case?
- What is the co-occurrence of PM among various forms of CM allegations?
- What is the relationship between the frequency of reported PM experiences and the frequency of general trauma symptoms?
Method
Study 1: PMI Item Development and Pilot
Following the steps of scale construction (Heppner et al., 2016), the initial version of the PMI used current literature and definitions from facilities nationwide that provide care for children who have experienced maltreatment and who are engaged with court systems, mental health agencies, or social services. Our lead researcher, Alison M. Boughn, developed a list of 20 items using category identifications from Glaser (2002) and APSAC (2019). Items were also created using Slep et al.’s (2015) proposed inclusion language for the Diagnostic and Statistical Manual of Mental Disorders (DSM-5) diagnostic codes and codes from the International Classification of Diseases, 11th edition (ICD-11) definition criteria (APA, 2013). Both Boughn and Daniel A. DeCino, our other researcher, reviewed items for consistency with the research literature and removed four redundant items. The final 16 items were reevaluated for readability for future child respondents using a web-based, age range–appropriate readability checker (Readable, n.d.) and were then presented to local SMEs in a content validity survey to determine which would be considered essential for children to report as part of a child PM assessment.
Expert Validation
A multidisciplinary team (MDT) serving as SMEs completed an online content validity survey created by Boughn. The survey was distributed by a Child Advocacy Center (CAC) manager to the MDT. Boughn used the survey results to validate the PMI’s item content relevance. Twenty respondents from the following professions completed the survey: mental health (n = 6), social services (n = 6), law enforcement (n = 3), and legal services (n = 5). The content validity ratio (CVR) was then calculated for the 16 proposed items.
Results. The content validity survey scale used a 3-point Likert-type scale: 0 = not necessary; 1 = useful, but not essential; and 2 = essential. A minimum of 15 of the 20 SMEs (75% of the sample), or a CVR ≥ .5, was required to deem an item essential (Lawshe, 1975). The significance level for each item’s content validity was set at α = .05 (Ayre & Scally, 2014). After conducting Lawshe’s (1975) CVR and applying the ratio correction developed by Ayre and Scally (2014), it was determined that eight items were essential: Item 2 (CVR = .7), Item 3 (CVR = .9), Item 4 (CVR = .6), Item 6 (CVR = .6), Item 7 (CVR = .8), Item 10 (CVR = .6), Item 15 (CVR = .5), and Item 16 (CVR = .6).
Upon further evaluation, and in an effort to ensure that the PMI items served the needs of interdisciplinary professionals, some items were rated essential for specific professions; these items still met the CVR requirements (CVR = 1) for the smaller within-group sample. These four items were unanimously endorsed by SMEs for a particular profession as essential: Item 5 (CVR Social Services = 1; CVR Law Enforcement = 1), Item 11 (CVR Law Enforcement = 1), Item 13 (CVR Law Enforcement = 1), and Item 14 (CVR Law Enforcement = 1).
Finally, an evaluation of the remaining four items was completed to explore if items were useful, but not essential. Using the minimum CVR ≥ .5, it was determined that these items should remain on the PMI: Item 1 (CVR = .9), Item 8 (CVR = .8), Item 9 (CVR = .9), and Item 12 (CVR = .9). The use of Siegle’s (2017) Reliability Calculator determined the Cronbach’s α level for the PMI to be 0.83, indicating adequate internal consistency. Additionally, a split-half (odd-even) correlation was completed with the Spearman-Brown adjustment of 0.88, indicating high reliability (Siegle, 2017).
Pilot Summary
The focus of the pilot study was to ensure effective implementation of the proposed research protocol following each respondent’s appointment at the CAC research site. The pilot was implemented to ensure research procedures did not interfere with typical appointments and standard procedures at the CAC. Participation in the PMI pilot was voluntary and no compensation was provided for respondents.
Sample. The study used a purposeful sample of children at a local, nationally accredited CAC in the Midwest; both the child and the child’s legal guardian agreed to participate. Because of the expected integration of PM with other forms of abuse, this population was selected to help create an understanding of how PM is experienced specifically with co-occurring cases of maltreatment. Respondents were children who (a) had an open CM case with social services and/or law enforcement, (b) were scheduled for an appointment at the CAC, and (c) were between the ages of 8 and 17.
Measures. The two measures implemented in this study were the developing PMI and the TSCC-SF. At the time of data collection, CAC staff implemented the TSCC-SF as a screening tool for referral services during CAC victim appointments. To ensure the research process did not interfere with chain-of-custody procedures, collected investigative testimony, or physical evidence that was obtained, the PMI was administered only after all normally scheduled CAC procedures were followed during appointments.
PMI. The current version of the PMI is a self-report measure that consists of 16 items on a 4-point Likert-type scale that mirrors the language of the TSCC-SF (0 = never to 3 = almost all the time). Respondents typically needed 5 minutes complete the PMI. Sample items from the PMI included questions like: “How often have you been told or made to feel like you are not important or unlovable?” The full instrument is not provided for use in this publication to ensure the PMI is not misused, as refinement of the PMI is still in progress.
TSCC-SF. In addition to the PMI, Boughn gathered data from the TSCC-SF (Briere & Wherry, 2016) because of its widespread use among clinicians to efficiently assess for sexual concerns, suicidal ideation frequency, and general trauma symptoms such as post-traumatic stress, depression, anger, disassociation, and anxiety (Wherry et al., 2013). The TSCC-SF measures a respondent’s frequency of perceived experiences and has been successfully implemented with children as young as 8 years old (Briere, 1996). The 20-item form uses a 4-point Likert-type scale (0 = never to 3 = almost all the time) composed of general trauma and sexual concerns subscales. The TSCC-SF has demonstrated high internal consistency and alpha values in the good to excellent ranges; it also has high intercorrelations between sexual concerns and other general trauma scales (Wherry & Dunlop, 2018).
Procedures. Respondents were recruited during their scheduled CAC appointment time. Each investigating agency (law enforcement or social services) scheduled a CAC appointment in accordance with an open maltreatment case. At the beginning of each respondent’s appointment, Boughn provided them with an introduction and description of the study. This included the IRB approvals from the hospital and university, an explanation of the informed consent and protected health information (PHI) authorization, and assent forms. Respondents aged 12 and older were asked to read and review the informed consent document with their legal guardian; respondents aged from 8 to 11 were provided an additional assent document to read. Respondents were informed they could stop the study at any time. After each respondent and legal guardian consented, respondents proceeded with their CAC appointment.
Typical CAC appointments consisted of a forensic interview, at times a medical exam, and administration of the TSCC-SF to determine referral needs. After these steps were completed, Boughn administered the PMI to those who agreed to participate in this research study. Following the completion of the TSCC-SF, respondents were verbally reminded of the study and asked if they were still willing to participate by completing the PMI. Willing respondents completed the PMI; afterward, Boughn asked respondents if they were comfortable leaving the assessment room. In the event the respondent voiced additional concerns of maltreatment during the PMI administration, Boughn made a direct report to the respondent’s investigator (i.e., law enforcement officer or social worker assigned to the respondent’s case).
Boughn accessed each respondent’s completed TSCC-SF from their electronic health record in accordance with the PHI authorization and consent after the respondent’s appointment. Data completed on the TSCC-SF allowed Boughn to gather information related to sexual concerns, suicidal ideation, and trauma symptomology. Data gathered from the TSCC-SF were examined with each respondent’s PMI responses.
Results. Respondents were 21 children (15 female, six male) with age ranges from 8 to 17 years with a median age of 12 years. Respondents described themselves as White (47.6%), Biracial (14.2%), Multiracial (14.2%), American Indian/Alaskan Native (10.0%), Black (10.0%), and Hispanic/Latino (5.0%). CM allegations for the respondents consisted of allegations of sexual abuse (86.0%), physical abuse (10.0%), and neglect (5.0%).
Every respondent’s responses were included in the analyses to ensure all maltreatment situations were considered. The reliability of the PMI observed in the pilot sample (N = 21) demonstrated high internal consistency with all 16 initial items (α = .88). The average total score on the PMI in the pilot was 13.29, with respondents’ scores ranging from 1 to 30. A Pearson correlation indicated total scores for the PMI and General Trauma Scale scores (reported on the TSCC-SF) were significantly correlated (r = .517, p < .05).
Study 2: Full Testing of the PMI
The next phase of research proceeded with the collection of a larger data sample (N = 166) to explore the item construct validity and internal reliability (Siyez et al., 2020). Study procedures, data collection, and data storage followed in the pilot study were also implemented with the larger sample. Boughn maintained tracking of respondents who did not want to participate in the study or were unable to because of cognitive functioning level, emergency situations, and emotional dysregulation concerns.
Sample
Based on a power analysis performed using the Raosoft (2004) sample size calculator, the large sample study required a minimum of 166 respondents for statistical significance (Ali, 2012; Heppner et al., 2016). The sample size was expected to account for a 10% margin of error and a 99% confidence level. The calculation of a 99% confidence interval was used to ensure the number of respondents could effectively represent the population accessed within the CAC based on the data from the CM Report (DHHS, 2018). Large sample population data was gathered between September 2018 and May 2019.
Measures
The PMI and TSCC-SF were also employed in Study 2 because of their successful implementation in the pilot. Administration of the TSCC-SF ensured a normed and standardized measure could aid in providing context to the information gathered on the PMI. No changes were made to the PMI or TSCC-SF measures following the review of procedures and analyses in the pilot.
Procedures
Recruitment and data collection/analyses processes mirrored that of the pilot study. Voluntary respondents were recruited at the CAC during their scheduled appointments. Respondents completed an informed consent, child assent, PHI authorization form, TSCC-SF, and PMI. Following the completion of data collection, Boughn completed data entry in the electronic health record to de-identify and analyze the results.
Results
Demographics
All data were analyzed using Statistical Package for the Social Sciences version 24 (SPSS-24). Initial data evaluation consisted of exploration of descriptive statistics, including demographic and criteria-based information related to respondents’ identities and case details. Respondents were between 8 to 17 years of age (M = 12.39) and primarily female (73.5%, n = 122), followed by male (25.3%, n = 42). Additionally, two respondents (n = 2) reported both male and female gender identities. Racial identities were marked by two categories: White (59.6%, n = 99) and Racially Diverse (40.4%, n = 67) respondents. The presenting maltreatment concerns and the child’s relationship to the offender are outlined in Table 1 and Table 2, respectively.
Reliability and Validity of the PMI
The reliability of the PMI observed in its implementation in Study 2 (N = 166) showed even better internal consistency with all 16 initial items (α =.91) than observed in the pilot. Using the Spearman-Brown adjustment (Warner, 2013), split-half reliability was calculated, indicating high internal reliability (.92). Internal consistencies were calculated using gender identity and age demographic variables (see Table 3).
Table 1
Child Maltreatment Allegation by Type (N = 166)
Allegation |
f |
Rel f |
cf |
% |
Sexual Abuse |
113 |
0.68 |
166 |
68.07 |
Physical Abuse |
29 |
0.17 |
53 |
17.47 |
Neglect |
14 |
0.08 |
24 |
8.43 |
Multiple Allegations |
6 |
0.04 |
10 |
3.61 |
Witness to Violence |
3 |
0.02 |
4 |
1.81 |
Kidnapping |
1 |
0.01 |
1 |
0.60 |
Note. Allegation type reported at initial appointment scheduling
Table 2
Identified Offender by Relationship to Victim (N = 166)
Offender Relationship |
f |
Rel f |
cf |
% |
Other Known Adult |
60 |
0.36 |
166 |
36.14 |
Parent |
48 |
0.29 |
106 |
28.92 |
Other Known Child (≤ age 15 years) |
15 |
0.09 |
58 |
9.04 |
Sibling-Child (≤ age 15 years) |
10 |
0.06 |
43 |
6.02 |
Unknown Adult |
9 |
0.05 |
33 |
5.42 |
Step-Parent |
8 |
0.05 |
24 |
4.82 |
Multiple Offenders |
6 |
0.04 |
16 |
3.61 |
Grandparent |
6 |
0.04 |
10 |
3.61 |
Sibling-Adult (≥ age 16 years) |
3 |
0.02 |
4 |
1.81 |
Unknown Child (≤ age 15 years) |
1 |
0.01 |
1 |
0.60 |
Note. Respondent knew the offender (n =156); Respondent did not know offender (n =10)
Table 3
Internal Consistency Coefficients (α) by Gender Identity and Age (N = 166)
Gender |
n |
α |
M |
SD |
Female |
122 |
0.90 |
13.2 |
9.1 |
Male |
42 |
0.94 |
13.5 |
11.0 |
Male–Female |
2 |
0.26 |
8.5 |
2.5 |
Age |
|
|
|
|
8–12 |
83 |
0.92 |
12.75 |
10.06 |
13–17 |
83 |
0.90 |
13.69 |
9.01 |
Note. SD = Standard Deviation; M = Mean
Respondents’ Demographic Characteristics and PM Experiences
For Research Question (RQ) 1 and RQ2, descriptive data were used to generate frequencies and determine the impact of demographic characteristics on average PMI score. To explore this further in RQ1, one-way ANOVAs were completed for the variables of age, gender, racial identity, allegation type, and offender relationships. No significant correlations were found between demographic variables and the PMI items. On average, respondents reported a frequency score of 13.5 (M = 13.5, SD = 9.5) on the PMI. Eight respondents (5%) endorsed no frequency of PM while 95% (N = 158) experienced PM.
Co-Occurrence of PM With Other Forms of Maltreatment
For RQ3, frequency and descriptive data were generated, revealing average age rates of PM reported by maltreatment type. Varying sample representations were discovered in each form of maltreatment (see Table 4). Clear evidence was found that PM co-occurs with each form of maltreatment type; however, how each form of maltreatment interacts with PM is currently unclear given the multiple dimensions of each maltreatment case including, but not limited to, severity, frequency, offender, and victim characteristics.
Table 4
Descriptive and Frequency Data for Co-Occurrence of PM (N = 166)
Allegation |
n |
M |
SD |
95% CI |
Sexual Abuse |
113 |
13.04 |
9.01 |
[11.37, 14.72] |
Physical Abuse |
29 |
12.45 |
10.53 |
[8.44, 16.45] |
Neglect |
14 |
14.57 |
12.16 |
[7.55, 21.60] |
Multiple Allegations |
5 |
17.40 |
8.88 |
[6.38, 28.42] |
Witness to Violence |
3 |
7.67 |
5.03 |
[–4.84, 20.17] |
Kidnapping |
1 |
n/a |
n/a |
Missing |
Note. CI = Confidence Interval; SD = Standard Deviation; M = Mean; n/a = not applicable
PM Frequency and General Trauma Symptoms
For RQ4, Pearson’s correlation was used to calculate frequency score relationships between the PMI and TSCC-SF. There was a statistically significant relationship between the PMI and total frequency of general trauma symptoms on the TSCC-SF [r(164) = .78, p < .01, r² = .61] (Sullivan & Feinn, 2012). Cohen’s d, calculated from the means for each item as well as the pooled standard deviation, indicated a small effect relationship (d = .15) between general trauma and PMI frequencies (see Figure 1).
Figure 1
Correlation Between PMI and TSCC-SF General Trauma Subscale
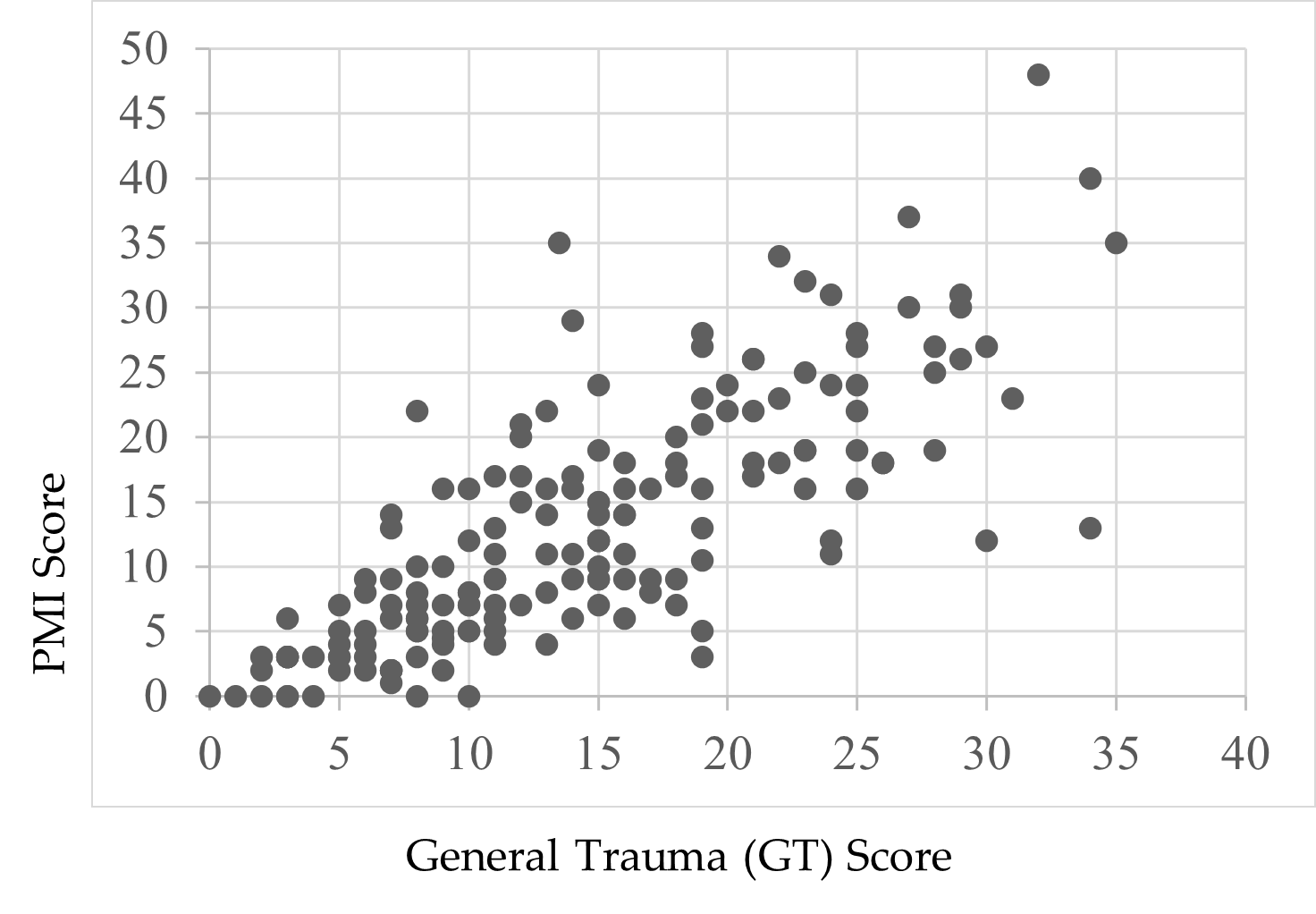
Note. Scores were endorsed by respondents’ self-reports.
Child Suicidal Ideation Reports and the PMI
Following a review of the findings of Thompson et al. (2005) and Wherry et al. (2013) that children who reported experiencing CM also experienced suicidal ideation, Boughn performed an additional two-way ANOVA that examined the effect of suicidal ideation on the PMI total score. A significant relationship—F(1, 164) = 49.52, p < .01, η2 = .23—between respondents’ PMI scores and thoughts of suicide was found. Respondents who did not report thoughts of suicide (59.0%, n = 98) indicated lower rates of PM (M = 9.37, SD = 7.97) compared to children who did report thoughts of suicide (41.0%, n = 68, M = 18.77, SD = 9.12). A preliminary review of this finding demonstrates the severity of PM’s impact on child victims.
Discussion
This study was designed with the aim of developing a tool to support accurate identification of PM among children and adolescents. Findings from its first large-scale implementation provide a foundational view to the occurrence of PM in terms of demographic characteristics, comorbidity of PM with other forms of abuse, and the relationship between PM and trauma. The analyses yielded both expected and unexpected results based on the extant research.
PM and Demographic Characteristics
Race
There was no significant effect when exploring the data related to racial demographics and PM. The respondent sample closely reflected the geographical area’s known racial demographics at the time of the study, reflecting a population approaching 80% White with residents of all other known races below 5% for each racial group (U.S. Census Bureau, 2020). Although researchers (Dakil et al., 2011) anticipated children identifying as racial minorities would be included in the representation of CM reports, evidence from this study potentially reveals a greater than expected gap in reporting for minority-race populations (Bernard & Harris, 2018; Font & Maguire-Jack, 2015). This suggests that there may be additional, unidentified barriers influencing the reporting of maltreatment among minority-race populations.
Gender
A lack of gender identity representation was evident in the data, consistent with prior research (Sivagurunathan et al., 2019). Respondents who self-identified with both male and female gender identities (1.2%) and as male (25.3%) were represented less frequently compared to female respondents (73.5%). This is not inherently a limitation of this study, as research shows that just 10% of males in the United States report their sexual abuse (Sivagurunathan et al., 2019). People who identify as male may face harmful cultural messages that enhance negative stigma for victims of abuse, causing increased feelings of weakness or vulnerability (Alaggia & Mishna, 2014). This finding may support claims that male trauma survivors feel stigmatized and report their experiences less frequently (Easton, 2012).
Additionally, children who identify outside traditional gender binary norms and definitions need more access to inclusive representation on screening assessments. Assessments like the TSCC-SF may be using antiquated gender- or biological sex–normed checkboxes, which leave certain groups underrepresented in research studies (Neukrug & Fawcett, 2015). These practices may present inaccurate findings, inadvertently reinforce discriminatory expectations, and generate inaccurate referrals. Non-binary youth encounter barriers that may compound their ability to effectively access supports in their daily life related to coming out, social violence, lack of peer and/or adult acceptance, discrimination, isolation, higher rates of suicide, and lack of representation in mainstream society (Bialer & McIntosh, 2016; Zimman, 2009). In this study, representation of non-binary respondents, specifically those who reported both male and female gender identities, was reported; this warrants further exploration to assess barriers among non-binary gender youth and their experiences with child PM (Bos et al., 2019).
Offender Relationships
Frequency data for a child’s relationship with the offender were not found to be significant either for known offenders (M = 13.35) or unknown offenders (M = 11.2). In this study, 94% of the respondents already knew their offender (n = 156). This finding is consistent with previous research that has found that although child abduction and stranger danger are real phenomena, children are more likely to experience CM as a result of relationships with familiar individuals (Walsh & Brandon, 2011).
Co-Occurrence of PM With Other Abuse
Only eight respondents (5%) endorsed no frequency of PM; the average total PM frequency rate for respondents in this study was 13.5 out of a possible 48, indicating extreme severity. In this study, we found evidence that PM is a co-occurring experience for children with open maltreatment cases, yet clinicians still lack formal, valid assessments to determine PM alone. Our findings support the National Children’s Alliance’s (NCA; 2016) call for clinicians to follow practice guidelines in accordance with state and national guidelines as they relate to mandatory reporting of CM concerns and determination of whether PM plus other forms of maltreatment may be present for child victims seeking services.
Comorbidity of PM and Trauma
PM-related experiences on the PMI and general trauma symptoms from the TSCC-SF warrant discussion. The PMI illustrated a significant relationship with the TSCC-SF general trauma subscale (Briere & Wherry, 2016). More than half (61%) of the variance on the PMI was connected to general trauma symptoms, suggesting that higher rates of PM experiences may increase trauma-related symptoms. For example, previous researchers have found adverse childhood experiences and signs of trauma-related symptoms lead to serious mental health diagnoses, early mortality, and/or significant biological health risks in children (Tyrka et al., 2013; Vachon et al., 2015; Zimmerman & Mercy, 2010). Further exploration to determine if and how PM influences other trauma-related symptoms in children throughout their life span would expand upon the results of this study.
Suicidal Ideation
Finally, our data revealed a significant effect between respondent endorsement of suicidal ideation and PMI total scores. PM experiences accounted for 23% of the variance for children who reported thoughts of suicide (41%, n = 68) compared to those who did not report thoughts of suicide (59%, n = 98). This finding is consistent with prior research exploring children’s experiences with maltreatment and suicidal thoughts (Thompson et al., 2005; Wherry et al., 2013).
Limitations
This study has several limitations. First, by developing the PMI using national definitions, some regional and localized nuances were not considered. Second, data collected for this study were from a single Midwest CAC; thus, the data are limited in geographic generalizability. Third, the majority of respondents were White, and a more diverse sample would have been more representative of the region in which data were collected. Fourth, 99% of respondents identified as either male or female and may reflect an underrepresentation of non-binary or gender fluid youth in the results of this study. Fifth, this study relied heavily on quantitative data, which limited the ability to analyze each individual’s experiences with PM as they might describe from their unique perspectives.
Implications for Research and Practice
The results of this study provide several areas for future research. While the PMI demonstrated good internal consistency across all items (α =.91), more research with diverse populations across the United States is needed. Research from other geographical locations may demonstrate how reporting patterns for PM interact with ethnicity, culture, and elements of social expectations (Spinazzola et al., 2014).
The initial results of this study indicate the PMI may be a useful tool for children to report PM experiences in CAC settings; however, future research at other CACs and similar treatment facilities is needed to determine the PMI’s true utility and scalability. Future analysis (i.e., exploratory factor analysis and confirmatory factor analysis) of the PMI may also identify factors and help refine the instrument.
More research with the PMI can expand researchers’ knowledge of PM and services needed to help children. Working with other CACs, child protection professionals, and the NCA may help bridge current gaps in interdisciplinary assessment and care and establish a stable and comprehensive understanding of PM (López et al., 2015). Furthermore, understanding how CACs are equipped to identify and handle PM cases may provide useful insights to help improve services for children in need. Although some CACs may have a variety of professionals working in specific roles, some CACs may be understaffed, causing staff to take on multiple and overlapping roles. It is important to understand if and how different combinations of trained professionals influence children reporting PM (Hart & Glaser, 2011; NCA, 2016).
More research with the PMI is needed for refinement and to ensure the instrument is not misused. Releasing the PMI at this stage to clinicians and researchers without a fully developed assessment manual may lead to inappropriate or ineffective administration of the PMI and potentially unethical practice that could place children at risk. Future research and refinement of the PMI may provide clinicians and researchers a reliable and valid tool that is grounded in consistent theory and practice.
Conclusion
The PMI was developed to assess child PM and offers researchers and clinicians useful findings. In supporting research (Arslan, 2017; Bernstein et al., 2013; Raparia et al., 2016), child PM is a serious and often harmful combination of experiences that requires professional intervention (APSAC, 2019). For children reporting PM experiences, the PMI may help mental health and other care providers determine which services are needed. Findings from this study suggest differences in demographic variables are minimal for PM. Overall PMI scores were correlated to the general trauma subscale on the TSCC-SF, and the PMI revealed higher rates of PM for children experiencing suicidal ideation. The findings are the beginning of a measure designed to illustrate the depth and frequency of PM for children. With the PMI, early PM intervention becomes possible for a once invisible form of maltreatment.
Conflict of Interest and Funding Disclosure
Data collected and content shared in this study
were part of a dissertation study, which was
awarded the 2020 Dissertation Excellence Award
by the National Board for Certified Counselors.
The Psychological Maltreatment Inventory (PMI)
items were not released in this publication to protect
victims of child maltreatment and to ensure future
publications can address comprehensive revisions
made to the PMI.
References
Ahern, E. C., Hershkowitz, I., Lamb, M. E., Blasbalg, U., & Winstanley, A. (2014). Support and reluctance in the pre-substantive phase of alleged child abuse victim investigative interviews: Revised versus standard NICHD protocols. Behavioral Sciences & the Law, 32(6), 762–774. https://doi.org/10.1002/bsl.2149
Alaggia, R., & Mishna, F. (2014). Self psychology and male child sexual abuse: Healing relational betrayal. Clinical Social Work Journal, 42(1), 41–48. https://doi.org/10.1007/s10615-013-0453-2
Ali, S. A. (2012). Sample size calculation and sampling techniques. Journal of the Pakistan Medical Association, 62(6), 624–626. https://jpma.org.pk/PdfDownload/3482
American Professional Society on the Abuse of Children. (2019). APSAC practice guidelines: The investigation and determination of suspected psychological maltreatment of children and adolescents. https://bit.ly/3jI7AhJ
American Psychiatric Association. (2013). Diagnostic and statistical manual of mental disorders (5th ed.).
Arslan, G. (2017). Psychological maltreatment, coping strategies, and mental health problems: A brief and effective measure of psychological maltreatment in adolescents. Child Abuse & Neglect, 68, 96–106. https://doi.org/10.1016/j.chiabu.2017.03.023
Ayre, C., & Scally, A. J. (2014). Critical values for Lawshe’s content validity ratio: Revisiting the original methods of calculation. Measurement and Evaluation in Counseling and Development, 47(1), 79–86. https://doi.org/10.1177%2F0748175613513808
Bernard, C., & Harris, P. (2018). Serious case reviews: The lived experience of Black children. Child & Family Social Work, 24(2), 256–263. https://doi.org/10.1111/cfs.12610
Bernstein, R. E., Measelle, J. R., Laurent, H. K., Musser, E. D., & Ablow, J. C. (2013). Sticks and stones may break my bones but words relate to adult physiology? Child abuse experience and women’s sympathetic nervous system response while self-reporting trauma. Journal of Aggression, Maltreatment & Trauma, 22(10), 1117–1136. https://doi.org/10.1080/10926771.2013.850138
Bialer, P. A., & McIntosh, C. A. (2016). Discrimination, stigma, and hate: The impact on the mental health and well-being of LGBT people. Journal of Gay & Lesbian Mental Health, 20(4), 297–298. https://doi.org/10.1080/19359705.2016.1211887
Bos, H., de Haas, S., & Kuyper, L. (2019). Lesbian, gay, and bisexual adults: Childhood gender nonconformity, childhood trauma, and sexual victimization. Journal of Interpersonal Violence, 34(3), 496–515. https://doi.org/10.1177%2F0886260516641285
Briere, J. (1996). Trauma Symptom Checklist for Children (TSCC), professional manual. Psychological Assessment Resources.
Briere, J., & Wherry, J. (2016). Development and validation of the TSCC Screening Form (TSCC-SF) and TSCYC Screening Form (TSCYC-SF). Psychological Assessment Resources.
Brown, S. M., Bender, K., Orsi, R., McCrae, J. S., Phillips, J. D., & Rienks, S. (2019). Adverse childhood experiences and their relationship to complex health profiles among child welfare–involved children: A classification and regression tree analysis. Health Services Research, 54(4), 902–911. https://doi.org/10.1111/1475-6773.13166
Centers for Disease Control. (2012). Child abuse and neglect cost the United States $124 billion [Press release]. https://bit.ly/3jYbpAF
Dakil, S. R., Cox, M., Lin, H., & Flores, G. (2011). Racial and ethnic disparities in physical abuse reporting and Child Protective Services interventions in the United States. Journal of the National Medical Association, 103(9–10), 926–931. https://doi.org/10.1016/S0027-9684(15)30449-1
Easton, S. D. (2012). Disclosure of child sexual abuse among adult male survivors. Clinical Social Work Journal, 41, 344–355. https://doi.org/10.1007/s10615-012-0420-3
Fang, X., Brown, D. S., Florence, C. S., & Mercy, J. A. (2012). The economic burden of child maltreatment in the United States and implications for prevention. Child Abuse & Neglect, 36(2), 156–165. https://doi.org/10.1016/j.chiabu.2011.10.006
Felitti, V. J., Anda, R. F., Nordenberg, D., Williamson, D. F., Spitz, A. M., Edwards, V., Koss, M. P., & Marks, J. S. (1998). Relationship of childhood abuse and household dysfunction to many of the leading causes of death in adults: The Adverse Childhood Experiences (ACE) study. American Journal of Preventive Medicine, 14(4), 245–258. https://doi.org/10.1016/S0749-3797(98)00017-8
Field, T. A., Jones, L. K., & Russell-Chapin, L. A. (Eds.). (2017). Neurocounseling: Brain-based clinical approaches. American Counseling Association.
Font, S. A., & Maguire-Jack, K. (2015). Decision-making in Child Protective Services: Influences at multiple levels of the social ecology. Child Abuse & Neglect, 47, 70–82. https://doi.org/10.1016/j.chiabu.2015.02.005
Glaser, D. (2002). Emotional abuse and neglect (psychological maltreatment): A conceptual framework. Child Abuse & Neglect, 26(6–7), 697–714. https://doi.org/10.1016/S0145-2134(02)00342-3
Greenfield, E. A., & Marks, N. F. (2010). Identifying experiences of physical and psychological violence in childhood that jeopardize mental health in adulthood. Child Abuse & Neglect, 34(3), 161–171. https://doi.org/10.1016/j.chiabu.2009.08.012
Hart, S. N., & Glaser, D. (2011). Psychological maltreatment – Maltreatment of the mind: A catalyst for advancing child protection toward proactive primary prevention and promotion of personal well-being. Child Abuse & Neglect, 35(10), 758–766. https://doi.org/10.1016/j.chiabu.2011.06.002
Heppner, P. P., Wampold, B. E., Owen, J., Thompson, M. N., & Wang, K. T. (2016). Research design in counseling (4th ed.). Cengage.
Iwaniec, D. (2006). The emotionally abused and neglected child: Identification, assessment and intervention: A practice handbook (2nd ed.). Wiley.
Klika, J. B., & Conte, J. R. (Eds.). (2017). The APSAC handbook on child maltreatment (4th ed.). SAGE.
Lawshe, C. H. (1975). A quantitative approach to content validity. Personnel Psychology, 28(4), 563–575. https://doi.org/10.1111/j.1744-6570.1975.tb01393.x
López, M., Fluke, J. D., Benbenishty, R., & Knorth, E. J. (2015). Commentary on decision-making and judgments in child maltreatment prevention and response: An overview. Child Abuse & Neglect, 49, 1–11. https://doi.org/10.1016/j.chiabu.2015.08.013
Maguire, S. A., Williams, B., Naughton, A. M., Cowley, L. E., Tempest, V., Mann, M. K., Teague, M., & Kemp, A. M. (2015). A systematic review of the emotional, behavioural and cognitive features exhibited by school-aged children experiencing neglect or emotional abuse. Child: Care, Health and Development, 41(5), 641–653. https://doi.org/10.1111/cch.12227
Marshall, N. A. (2012). A clinician’s guide to recognizing and reporting parental psychological maltreatment of children. Professional Psychology: Research and Practice, 43(2), 73–79. https://doi.org/10.1037/a0026677
Mills, R., Scott, J., Alati, R., O’Callaghan, M., Najman, J. M., & Strathearn, L. (2013). Child maltreatment and adolescent mental health problems in a large birth cohort. Child Abuse & Neglect, 37(5), 292–302. https://doi.org/10.1016/j.chiabu.2012.11.008
National Children’s Alliance. (2016). Putting standards into practice: A guide to implementing the 2017 standards for accredited members (revised 2016). http://www.nationalchildrensalliance.org/wp-content/uploads/2015/06/NCA2017-StandardsIntoPractice-web.pdf
Neukrug, E. S., & Fawcett, R. C. (2015). The essentials of testing and assessment: A practical guide for counselors, social workers, and psychologies, enhanced (3rd ed.). Cengage.
Peterson, C., Florence, C., & Klevens, J. (2018). The economic burden of child maltreatment in the United States, 2015. Child Abuse & Neglect, 86, 178–183.
https://doi.org/10.1016/j.chiabu.2018.09.018
Porges, S. W. (2011). The polyvagal theory: Neurophysiological foundations of emotions, attachment, communication, and self-regulation. W. W. Norton.
Raosoft. (2004). Sample size calculator. http://www.raosoft.com/samplesize.html
Raparia, E., Coplan, J. D., Abdallah, C. G., Hof, P. R., Mao, X., Mathew, S. J., & Shungu, D. C. (2016). Impact of childhood emotional abuse on neocortical neurometabolites and complex emotional processing in patients with generalized anxiety disorder. Journal of Affective Disorders, 190, 414–423. https://doi.org/10.1016/j.jad.2015.09.019
Readable. (n.d.). https://readable.com
Siegle, R. (2017). Educational research basics: Excel spreadsheet to calculate instrument reliability estimates. https://researchbasics.education.uconn.edu/excel-spreadsheet-to-calculate-instrument-reliability-estimates
Sivagurunathan, M., Orchard, T., & Evans, M. (2019). Barriers to utilization of mental health services amongst male child sexual abuse survivors: Service providers’ perspective. Journal of Child Sexual Abuse, 28(7), 819–839. https://doi.org/10.1080/10538712.2019.1610823
Siyez, D. M., Esen, E., Seymenler, S., & Öztürk, B. (2020). Development of wellness scale for emerging adults: Validity and reliability study. Current Psychology.
https://doi.org/10.1007/s12144-020-00672-w
Slep, A. M. S., Heyman, R. E., & Foran, H. M. (2015). Child maltreatment in DSM-5 and ICD-11. Family Process, 54(1), 17–32. https://doi.org/10.1111/famp.12131
Spinazzola, J., Hodgdon, H., Liang, L.-J., Ford, J. D., Layne, C. M., Pynoos, R., Briggs, E. C., Stolbach, B., & Kisiel, C. (2014). Unseen wounds: The contribution of psychological maltreatment to child and adolescent mental health and risk outcomes. Psychological Trauma: Theory, Research, Practice, and Policy, 6(Suppl 1), S18–S28. https://doi.org/10.1037/a0037766
Sullivan, G. M., & Feinn, R. (2012). Using effect size—or why the p value is not enough. Journal of Graduate Medical Education, 4(3), 279–282. https://doi.org/10.4300/JGME-D-12-00156.1
Thompson, R., Briggs, E., English, D. J., Dubowitz, H., Lee, L.-C., Brody, K., Everson, M. D., & Hunter, W. M. (2005). Suicidal ideation among 8-year-olds who are maltreated and at risk: Findings from the LONGSCAN studies. Child Maltreatment, 10(1), 26–36. https://doi.org/10.1177%2F1077559504271271
Tonmyr, L., Draca, J., Crain, J., & MacMillian, H. L. (2011). Measurement of emotional/psychological child maltreatment: A review. Child Abuse & Neglect, 35(10), 767–782.
https://doi.org/10.1016/j.chiabu.2011.04.011
Tyrka, A. R., Burgers, D. E., Philip, N. S., Price, L. H., & Carpenter, L. L. (2013). The neurobiological correlates of childhood adversity and implications for treatment. Acta Psychiatrica Scandinavica, 128(6), 434–447. https://doi.org/10.1111/acps.12143
U.S. Census Bureau. (2020). Quick facts. https://www.census.gov
U.S. Department of Health & Human Services. (2018). Child maltreatment 2016 (27th ed.). https://www.acf.hhs.gov/sites/default/files/documents/cb/cm2016.pdf
Vachon, D. D., Krueger, R. F., Rogosch, F. A., & Cicchetti, D. (2015). Assessment of the harmful psychiatric and behavioral effects of different forms of child maltreatment. JAMA Psychiatry, 72(11), 1135–1142. https://doi.org/10.1001/jamapsychiatry.2015.1792
van der Kolk, B. (2014). The body keeps the score: Brain, mind, and body in the healing of trauma. Penguin Books.
van Harmelen, A.-L., Elzinga, B. M., Kievit, R. A., & Spinhoven, P. (2011). Intrusions of autobiographical memories in individuals reporting childhood emotional maltreatment. European Journal of Psychotraumatology, 2(1), 7336. https://doi.org/10.3402/ejpt.v2i0.7336
van Harmelen, A.-L., van Tol, M.-J., van der Wee, N. J. A., Veltman, D. J., Aleman, A., Spinhoven, P., van Buchem, M. A., Zitman, F. G., Penninx, B. W. J. H., & Elzinga, B. M. (2010). Reduced medial prefrontal cortex volume in adults reporting childhood emotional maltreatment. Biological Psychiatry, 68(9), 832–838. https://doi.org/10.1016/j.biopsych.2010.06.011
Walsh, K., & Brandon, L. (2011). Their children’s first educators: Parents’ views about child sexual abuse prevention education. Journal of Child and Family Studies, 21, 734–746.
https://doi.org/10.1007/s10826-011-9526-4
Warner, R. M. (2013). Applied statistics: From bivariate through multivariate techniques (2nd ed.). SAGE.
Wherry, J. N., Baldwin, S., Junco, K., & Floyd, B. (2013). Suicidal thoughts/behaviors in sexually abused children. Journal of Child Sexual Abuse, 22(5), 534–551. https://doi.org/10.1080/10538712.2013.800938
Wherry, J. N., & Dunlop, C. E. (2018). TSCC and TSCYC screening forms in a clinical sample: Reliability, validity, and creating local clinical norms. Child Maltreatment, 23(1), 74–84.
https://doi.org/10.1177%2F1077559517725207
Zimman, L. (2009). ‘The other kind of coming out’: Transgender people and the coming out narrative genre. Gender and Language, 3(1), 53–80. https://doi.org/10.1558/genl.v3i1.53
Zimmerman, F., & Mercy, J. (2010). A better start: Child maltreatment prevention as a public
health priority. Zero to Three, 30(5), 4–10.
Alison M. Boughn, PhD, NCC, LIMHP (NE), LMHC (IA), LPC-MH (SD), ATR-BC, QMHP, TF-CBT, is an assistant professor and counseling department chair at Wayne State College. Daniel A. DeCino, PhD, NCC, LPC, is an assistant professor and Interim Program Coordinator at the University of South Dakota. Correspondence may be addressed to Alison M. Boughn, Wayne State College, 1111 Main Street, Wayne, NE 68787, albough1@wsc.edu.
Aug 20, 2021 | Author Videos, Volume 11 - Issue 3
Warren N. Ponder, Elizabeth A. Prosek, Tempa Sherrill
First responders are continually exposed to trauma-related events. Resilience is evidenced as a protective factor for mental health among first responders. However, there is a lack of assessments that measure the construct of resilience from a strength-based perspective. The present study used archival data from a treatment-seeking sample of 238 first responders to validate the 22-item Response to Stressful Experiences Scale (RSES-22) and its abbreviated version, the RSES-4, with two confirmatory factor analyses. Using a subsample of 190 first responders, correlational analyses were conducted of the RSES-22 and RSES-4 with measures of depressive symptoms, post-traumatic stress, anxiety, and suicidality confirming convergent and criterion validity. The two confirmatory analyses revealed a poor model fit for the RSES-22; however, the RSES-4 demonstrated an acceptable model fit. Overall, the RSES-4 may be a reliable and valid measure of resilience for treatment-seeking first responder populations.
Keywords: first responders, resilience, assessment, mental health, confirmatory factor analysis
First responder populations (i.e., law enforcement, emergency medical technicians, and fire rescue) are often repeatedly exposed to traumatic and life-threatening conditions (Greinacher et al., 2019). Researchers have concluded that such critical incidents could have a deleterious impact on first responders’ mental health, including the development of symptoms associated with post-traumatic stress, anxiety, depression, or other diagnosable mental health disorders (Donnelly & Bennett, 2014; Jetelina et al., 2020; Klimley et al., 2018; Weiss et al., 2010). In a systematic review, Wild et al. (2020) suggested the promise of resilience-based interventions to relieve trauma-related psychological disorders among first responders. However, they noted the operationalization and measure of resilience as limitations to their intervention research. Indeed, researchers have conflicting viewpoints on how to define and assess resilience. For example, White et al. (2010) purported popular measures of resilience rely on a deficit-based approach. Counselors operate from a strength-based lens (American Counseling Association [ACA], 2014) and may prefer measures with a similar perspective. Additionally, counselors are mandated to administer assessments with acceptable psychometric properties that are normed on populations representative of the client (ACA, 2014, E.6.a., E.7.d.). For counselors working with first responder populations, resilience may be a factor of importance; however, appropriately measuring the construct warrants exploration. Therefore, the focus of this study was to validate a measure of resilience with strength-based principles among a sample of first responders.
Risk and Resilience Among First Responders
In a systematic review of the literature, Greinacher et al. (2019) described the incidents that first responders may experience as traumatic, including first-hand life-threatening events; secondary exposure and interaction with survivors of trauma; and frequent exposure to death, dead bodies, and injury. Law enforcement officers (LEOs) reported that the most severe critical incidents they encounter are making a mistake that injures or kills a colleague; having a colleague intentionally killed; and making a mistake that injures or kills a bystander (Weiss et al., 2010). Among emergency medical technicians (EMTs), critical incidents that evoked the most self-reported stress included responding to a scene involving family, friends, or others to the crew and seeing someone dying (Donnelly & Bennett, 2014). Exposure to these critical incidents may have consequences for first responders. For example, researchers concluded first responders may experience mental health symptoms as a result of the stress-related, repeated exposure (Jetelina et al., 2020; Klimley et al., 2018; Weiss et al., 2010). Moreover, considering the cumulative nature of exposure (Donnelly & Bennett, 2014), researchers concluded first responders are at increased risk for post-traumatic stress disorder (PTSD), depression, and generalized anxiety symptoms (Jetelina et al., 2020; Klimley et al., 2018; Weiss et al., 2010). Symptoms commonly experienced among first responders include those associated with post-traumatic stress, anxiety, and depression.
In a collective review of first responders, Kleim and Westphal (2011) determined a prevalence rate for PTSD of 8%–32%, which is higher than the general population lifetime rate of 6.8–7.8 % (American Psychiatric Association [APA], 2013; National Institute of Mental Health [NIMH], 2017). Some researchers have explored rates of PTSD by specific first responder population. For example, Klimley et al. (2018) concluded that 7%–19% of LEOs and 17%–22% of firefighters experience PTSD. Similarly, in a sample of LEOs, Jetelina and colleagues (2020) reported 20% of their participants met criteria for PTSD.
Generalized anxiety and depression are also prevalent mental health symptoms for first responders. Among a sample of firefighters and EMTs, 28% disclosed anxiety at moderate–severe and several levels (Jones et al., 2018). Furthermore, 17% of patrol LEOs reported an overall prevalence of generalized anxiety disorder (Jetelina et al., 2020). Additionally, first responders may be at higher risk for depression (Klimley et al., 2018), with estimated prevalence rates of 16%–26% (Kleim & Westphal, 2011). Comparatively, the past 12-month rate of major depressive disorder among the general population is 7% (APA, 2013). In a recent study, 16% of LEOs met criteria for major depressive disorder (Jetelina et al., 2020). Moreover, in a sample of firefighters and EMTs, 14% reported moderate–severe and severe depressive symptoms (Jones et al., 2018). Given these higher rates of distressful mental health symptoms, including post-traumatic stress, generalized anxiety, and depression, protective factors to reduce negative impacts are warranted.
Resilience
Broadly defined, resilience is “the ability to adopt to and rebound from change (whether it is from stress or adversity) in a healthy, positive and growth-oriented manner” (Burnett, 2017, p. 2). White and colleagues (2010) promoted a positive psychology approach to researching resilience, relying on strength-based characteristics of individuals who adapt after a stressor event. Similarly, other researchers explored how individuals’ cognitive flexibility, meaning-making, and restoration offer protection that may be collectively defined as resilience (Johnson et al., 2011).
A key element among definitions of resilience is one’s exposure to stress. Given their exposure to trauma-related incidents, first responders require the ability to cope or adapt in stressful situations (Greinacher et al., 2019). Some researchers have defined resilience as a strength-based response to stressful events (Burnett, 2017), in which healthy coping behaviors and cognitions allow individuals to overcome adverse experiences (Johnson et al., 2011; White et al., 2010). When surveyed about positive coping strategies, first responders most frequently reported resilience as important to their well-being (Crowe et al., 2017).
Researchers corroborated the potential impact of resilience for the population. For example, in samples of LEOs, researchers confirmed resilience served as a protective factor for PTSD (Klimley et al., 2018) and as a mediator between social support and PTSD symptoms (McCanlies et al., 2017). In a sample of firefighters, individual resilience mediated the indirect path between traumatic events and global perceived stress of PTSD, along with the direct path between traumatic events and PTSD symptoms (Lee et al., 2014). Their model demonstrated that those with higher levels of resilience were more protected from traumatic stress. Similarly, among emergency dispatchers, resilience was positively correlated with positive affect and post-traumatic growth, and negatively correlated with job stress (Steinkopf et al., 2018). The replete associations of resilience as a protective factor led researchers to develop resilience-based interventions. For example, researchers surmised promising results from mindfulness-based resilience interventions for firefighters (Joyce et al., 2019) and LEOs (Christopher et al., 2018). Moreover, Antony and colleagues (2020) concluded that resilience training programs demonstrated potential to reduce occupational stress among first responders.
Assessment of Resilience
Recognizing the significance of resilience as a mediating factor in PTSD among first responders and as a promising basis for interventions when working with LEOs, a reliable means to measure it among first responder clients is warranted. In a methodological review of resilience assessments, Windle and colleagues (2011) identified 19 different measures of resilience. They found 15 assessments were from original development and validation studies with four subsequent validation manuscripts from their original assessment, of which none were developed with military or first responder samples.
Subsequently, Johnson et al. (2011) developed the Response to Stressful Experiences Scale (RSES-22) to assess resilience among military populations. Unlike deficit-based assessments of resilience, they proposed a multidimensional construct representing how individuals respond to stressful experiences in adaptive or healthy ways. Cognitive flexibility, meaning-making, and restoration were identified as key elements when assessing for individuals’ characteristics connected to resilience when overcoming hardships. Initially they validated a five-factor structure for the RSES-22 with military active-duty and reserve components. Later, De La Rosa et al. (2016) re-examined the RSES-22. De La Rosa and colleagues discovered a unidimensional factor structure of the RSES-22 and validated a shorter 4-item subset of the instrument, the RSES-4, again among military populations.
It is currently unknown if the performance of the RSES-4 can be generalized to first responder populations. While there are some overlapping experiences between military populations and first responders in terms of exposure to trauma and high-risk occupations, the Substance Abuse and Mental Health Services Administration (SAMHSA; 2018) suggested differences in training and types of risk. In the counseling profession, these populations are categorized together, as evidenced by the Military and Government Counseling Association ACA division. Additionally, there may also be dual identities within the populations. For example, Lewis and Pathak (2014) found that 22% of LEOs and 15% of firefighters identified as veterans. Although the similarities of the populations may be enough to theorize the use of the same resilience measure, validation of the RSES-22 and RSES-4 among first responders remains unexamined.
Purpose of the Study
First responders are repeatedly exposed to traumatic and stressful events (Greinacher et al., 2019) and this exposure may impact their mental health, including symptoms of post-traumatic stress, anxiety, depression, and suicidality (Jetelina et al., 2020; Klimley et al., 2018). Though most measures of resilience are grounded in a deficit-based approach, researchers using a strength-based approach proposed resilience may be a protective factor for this population (Crowe et al., 2017; Wild et al., 2020). Consequently, counselors need a means to assess resilience in their clinical practice from a strength-based conceptualization of clients.
Johnson et al. (2011) offered a non-deficit approach to measuring resilience in response to stressful events associated with military service. Thus far, researchers have conducted analyses of the RSES-22 and RSES-4 with military populations (De La Rosa et al., 2016; Johnson et al., 2011; Prosek & Ponder, 2021), but not yet with first responders. While there are some overlapping characteristics between the populations, there are also unique differences that warrant research with discrete sampling (SAMHSA, 2018). In light of the importance of resilience as a protective factor for mental health among first responders, the purpose of the current study was to confirm the reliability and validity of the RSES-22 and RSES-4 when utilized with this population. In the current study, we hypothesized the measures would perform similarly among first responders and if so, the RSES-4 would offer counselors a brief assessment option in clinical practice that is both reliable and valid.
Method
Participants
Participants in the current non-probability, purposive sample study were first responders (N = 238) seeking clinical treatment at an outpatient, mental health nonprofit organization in the Southwestern United States. Participants’ mean age was 37.53 years (SD = 10.66). The majority of participants identified as men (75.2%; n = 179), with women representing 24.8% (n = 59) of the sample. In terms of race and ethnicity, participants identified as White (78.6%; n = 187), Latino/a (11.8%; n = 28), African American or Black (5.5%; n = 13), Native American (1.7%; n = 4), Asian American (1.3%; n = 3), and multiple ethnicities (1.3%; n = 3). The participants identified as first responders in three main categories: LEO (34.9%; n = 83), EMT (28.2%; n = 67), and fire rescue (25.2%; n = 60). Among the first responders, 26.9% reported previous military affiliation. As part of the secondary analysis, we utilized a subsample (n = 190) that was reflective of the larger sample (see Table 1).
Procedure
The data for this study were collected between 2015–2020 as part of the routine clinical assessment procedures at a nonprofit organization serving military service members, first responders, frontline health care workers, and their families. The agency representatives conduct clinical assessments with clients at intake, Session 6, Session 12, and Session 18 or when clinical services are concluded. We consulted with the second author’s Institutional Review Board, which determined the research as exempt, given the de-identified, archival nature of the data. For inclusion in this analysis, data needed to represent first responders, ages 18 or older, with a completed RSES-22 at intake. The RSES-4 are four questions within the RSES-22 measure; therefore, the participants did not have to complete an additional measure. For the secondary analysis, data from participants who also completed other mental health measures at intake were also included (see Measures).
Table 1
Demographics of Sample
Characteristic |
Sample 1
(N = 238) |
Sample 2
(n = 190) |
Age (Years) |
|
|
Mean |
37.53 |
37.12 |
Median |
35.50 |
35.00 |
SD |
10.66 |
10.30 |
Range |
46 |
45 |
Time in Service (Years) |
|
|
Mean |
11.62 |
11.65 |
Median |
10.00 |
10.00 |
SD |
9.33 |
9.37 |
Range |
41 |
39 |
|
n (%) |
First Responder Type |
|
|
Emergency Medical
Technicians |
67 (28.2%) |
54 (28.4%) |
Fire Rescue |
60 (25.2%) |
45 (23.7%) |
Law Enforcement |
83 (34.9%) |
72 (37.9%) |
Other |
9 (3.8%) |
5 (2.6%) |
Two or more |
10 (4.2%) |
6 (3.2%) |
Not reported |
9 (3.8%) |
8 (4.2%) |
Gender |
|
|
Women |
59 (24.8%) |
47 (24.7%) |
Men |
179 (75.2%) |
143 (75.3%) |
Ethnicity |
|
|
African American/Black |
13 (5.5%) |
8 (4.2%) |
Asian American |
3 (1.3%) |
3 (1.6%) |
Latino(a)/Hispanic |
28 (11.8%) |
24 (12.6%) |
Multiple Ethnicities |
3 (1.3%) |
3 (1.6%) |
Native American |
4 (1.7%) |
3 (1.6%) |
White |
187 (78.6%) |
149 (78.4%) |
Note. Sample 2 is a subset of Sample 1. Time in service for Sample 1, n = 225;
time in service for Sample 2, n = 190.
Measures
Response to Stressful Experiences Scale
The Response to Stressful Experiences Scale (RSES-22) is a 22-item measure to assess dimensions of resilience, including meaning-making, active coping, cognitive flexibility, spirituality, and self-efficacy (Johnson et al., 2011). Participants respond to the prompt “During and after life’s most stressful events, I tend to” on a 5-point Likert scale from 0 (not at all like me) to 4 (exactly like me). Total scores range from 0 to 88 in which higher scores represent greater resilience. Example items include see it as a challenge that will make me better, pray or meditate, and find strength in the meaning, purpose, or mission of my life. Johnson et al. (2011) reported the RSES-22 demonstrates good internal consistency (α = .92) and test-retest reliability (α = .87) among samples from military populations. Further, the developers confirmed convergent, discriminant, concurrent, and incremental criterion validity (see Johnson et al., 2011). In the current study, Cronbach’s alpha of the total score was .93.
Adapted Response to Stressful Experiences Scale
The adapted Response to Stressful Experiences Scale (RSES-4) is a 4-item measure to assess resilience as a unidimensional construct (De La Rosa et al., 2016). The prompt and Likert scale are consistent with the original RSES-22; however, it only includes four items: find a way to do what’s necessary to carry on, know I will bounce back, learn important and useful life lessons, and practice ways to handle it better next time. Total scores range from 0 to 16, with higher scores indicating greater resilience. De La Rosa et al. (2016) reported acceptable internal consistency (α = .76–.78), test-retest reliability, and demonstrated criterion validity among multiple military samples. In the current study, the Cronbach’s alpha of the total score was .74.
Patient Health Questionnaire-9
The Patient Health Questionnaire-9 (PHQ-9) is a 9-item measure to assess depressive symptoms in the past 2 weeks (Kroenke et al., 2001). Respondents rate the frequency of their symptoms on a 4-point Likert scale ranging from 0 (not at all) to 3 (nearly every day). Total scores range from 0 to 27, in which higher scores indicate increased severity of depressive symptoms. Example items include little interest or pleasure in doing things and feeling tired or having little energy. Kroenke et al. (2001) reported good internal consistency (α = .89) and established criterion and construct validity. In this sample, Cronbach’s alpha of the total score was .88.
PTSD Checklist-5
The PTSD Checklist-5 (PCL-5) is a 20-item measure for the presence of PTSD symptoms in the past month (Blevins et al., 2015). Participants respond on a 5-point Likert scale indicating frequency of PTSD-related symptoms from 0 (not at all) to 4 (extremely). Total scores range from 0 to 80, in which higher scores indicate more severity of PTSD-related symptoms. Example items include repeated, disturbing dreams of the stressful experience and trouble remembering important parts of the stressful experience. Blevins et al. (2015) reported good internal consistency (α = .94) and determined convergent and discriminant validity. In this sample, Cronbach’s alpha of the total score was .93.
Generalized Anxiety Disorder-7
The Generalized Anxiety Disorder-7 (GAD-7) is a 7-item measure to assess for anxiety symptoms over the past 2 weeks (Spitzer et al., 2006). Participants rate the frequency of the symptoms on a 4-point Likert scale ranging from 0 (not at all) to 3 (nearly every day). Total scores range from 0 to 21 with higher scores indicating greater severity of anxiety symptoms. Example items include not being able to stop or control worrying and becoming easily annoyed or irritable. Among patients from primary care settings, Spitzer et al. (2006) determined good internal consistency (α = .92) and established criterion, construct, and factorial validity. In this sample, Cronbach’s alpha of the total score was .91.
Suicidal Behaviors Questionnaire-Revised
The Suicidal Behaviors Questionnaire-Revised (SBQ-R) is a 4-item measure to assess suicidality (Osman et al., 2001). Each item assesses a different dimension of suicidality: lifetime ideation and attempts, frequency of ideation in the past 12 months, threat of suicidal behaviors, and likelihood of suicidal behaviors (Gutierrez et al., 2001). Total scores range from 3 to 18, with higher scores indicating more risk of suicide. Example items include How often have you thought about killing yourself in the past year? and How likely is it that you will attempt suicide someday? In a clinical sample, Osman et al. (2001) reported good internal consistency (α = .87) and established criterion validity. In this sample, Cronbach’s alpha of the total score was .85.
Data Analysis
Statistical analyses were conducted using SPSS version 26.0 and SPSS Analysis of Moment Structures (AMOS) version 26.0. We examined the dataset for missing values, replacing 0.25% (32 of 12,836 values) of data with series means. We reviewed descriptive statistics of the RSES-22 and RSES-4 scales. We determined multivariate normality as evidenced by skewness less than 2.0 and kurtosis less than 7.0 (Dimitrov, 2012). We assessed reliability for the scales by interpreting Cronbach’s alphas and inter-item correlations to confirm internal consistency.
We conducted two separate confirmatory factor analyses to determine the model fit and factorial validity of the 22-item measure and adapted 4-item measure. We used several indices to conclude model fit: minimum discrepancy per degree of freedom (CMIN/DF) and p-values, root mean residual (RMR), goodness-of-fit index (GFI), comparative fit index (CFI), Tucker-Lewis index (TLI), and the root mean square error of approximation (RMSEA). According to Dimitrov (2012), values for the CMIN/DF < 2.0,p > .05, RMR < .08, GFI > .90, CFI > .90, TLI > .90, and RMSEA < .10 provide evidence of a strong model fit. To determine criterion validity, we assessed a subsample of participants (n = 190) who had completed the RSES-22, RSES-4, and four other psychological measures (i.e., PHQ-9, PCL-5, GAD-7, and SBQ-R). We determined convergent validity by conducting bivariate correlations between the RSES-22 and RSES-4.
Results
Descriptive Analyses
We computed means, standard deviations, 95% confidence interval (CI), and score ranges for the RSES-22 and RSES-4 (Table 2). Scores on the RSES-22 ranged from 19–88. Scores on the RSES-4 ranged from 3–16. Previous researchers using the RSES-22 on military samples reported mean scores of 57.64–70.74 with standard deviations between 8.15–15.42 (Johnson et al., 2011; Prosek & Ponder, 2021). In previous research of the RSES-4 with military samples, mean scores were 9.95–11.20 with standard deviations between 3.02–3.53(De La Rosa et al., 2016; Prosek & Ponder, 2021).
Table 2
Descriptive Statistics for RSES-22 and RSES-4
Variable |
M |
SD |
95% CI |
Score Range |
RSES-22 scores |
60.12 |
13.76 |
58.52, 61.86 |
19–88 |
RSES-4 scores |
11.66 |
2.62 |
11.33, 11.99 |
3–16 |
Note. N = 238. RSES-22 = Response to Stressful Experiences Scale 22-item; RSES-4 = Response
to Stressful Experiences Scale 4-item adaptation.
Reliability Analyses
To determine the internal consistency of the resiliency measures, we computed Cronbach’s alphas. For the RSES-22, we found strong evidence of inter-item reliability (α = .93), which was consistent with the developers’ estimates (α = .93; Johnson et al., 2011). For the RSES-4, we assessed acceptable inter-item reliability (α = .74), which was slightly lower than previous estimates (α = .76–.78; De La Rosa et al., 2016). We calculated the correlation between items and computed the average of all the coefficients. The average inter-item correlation for the RSES-22 was .38, which falls within the acceptable range (.15–.50). The average inter-item correlation for the RSES-4 was .51, slightly above the acceptable range. Overall, evidence of internal consistency was confirmed for each scale.
Factorial Validity Analyses
We conducted two confirmatory factor analyses to assess the factor structure of the RSES-22 and RSES-4 for our sample of first responders receiving mental health services at a community clinic (Table 3). For the RSES-22, a proper solution converged in 10 iterations. Item loadings ranged between .31–.79, with 15 of 22 items loading significantly ( > .6) on the latent variable. It did not meet statistical criteria for good model fit: χ2 (209) = 825.17, p = .000, 90% CI [0.104, 0.120]. For the RSES-4, a proper solution converged in eight iterations. Item loadings ranged between .47–.80, with three of four items loading significantly ( > .6) on the latent variable. It met statistical criteria for good model fit: χ2 (2) = 5.89, p = .053, 90% CI [0.000, 0.179]. The CMIN/DF was above the suggested < 2.0 benchmark; however, the other fit indices indicated a model fit.
Table 3
Confirmatory Factor Analysis Fit Indices for RSES-22 and RSES-4
Variable |
df |
χ2 |
CMIN/DF |
RMR |
GFI |
CFI |
TLI |
RMSEA |
90% CI |
RSES-22 |
209 |
825.17/.000 |
3.95 |
.093 |
.749 |
.771 |
0.747 |
.112 |
0.104, 0.120 |
RSES-4 |
2 |
5.89/.053 |
2.94 |
.020 |
.988 |
.981 |
0.944 |
.091 |
0.000, 0.179 |
Note. N = 238. RSES-22 = Response to Stressful Experiences Scale 22-item; RSES-4 = Response to Stressful Experiences Scale 4-item adaptation; CMIN/DF = Minimum Discrepancy per Degree of Freedom; RMR = Root Mean Square Residual;
GFI = Goodness-of-Fit Index; CFI = Comparative Fit Index; TLI = Tucker-Lewis Index; RMSEA = Root Mean Squared Error of Approximation.
Criterion and Convergent Validity Analyses
To assess for criterion validity of the RSES-22 and RSES-4, we conducted correlational analyses with four established psychological measures (Table 4). We utilized a subsample of participants (n = 190) who completed the PHQ-9, PCL-5, GAD-7, and SBQ-R at intake. Normality of the data was not a concern because analyses established appropriate ranges for skewness and kurtosis (± 1.0). The internal consistency of the RSES-22 (α = .93) and RSES-4 (α = .77) of the subsample was comparable to the larger sample and previous studies. The RSES-22 and RSES-4 related to the psychological measures of distress in the expected direction, meaning measures were significantly and negatively related, indicating that higher resiliency scores were associated with lower scores of symptoms associated with diagnosable mental health disorders (i.e., post-traumatic stress, anxiety, depression, and suicidal behavior). We verified convergent validity with a correlational analysis of the RSES-22 and RSES-4, which demonstrated a significant and positive relationship.
Table 4
Criterion and Convergent Validity of RSES-22 and RSES-4
|
M (SD) |
Cronbach’s α |
RSES-22 |
PHQ-9 |
PCL-5 |
GAD-7 |
SBQ-R |
RSES-22 |
60.16 (14.17) |
.93 |
— |
−.287* |
−.331* |
−.215* |
−.346* |
RSES-4 |
11.65 (2.68) |
.77 |
.918 |
−.290* |
−.345* |
−.220* |
−.327* |
Note. n = 190. RSES-22 = Response to Stressful Experiences Scale 22-item; RSES-4 = Response to Stressful Experiences Scale 4-item adaptation; PHQ-9 = Patient Health Questionnaire-9;
PCL-5 = PTSD Checklist-5; GAD-7 = Generalized Anxiety Disorder-7; SBQ-R = Suicidal Behaviors Questionnaire-Revised.
*p < .01.
Discussion
The purpose of this study was to validate the factor structure of the RSES-22 and the abbreviated RSES-4 with a first responder sample. Aggregated means were similar to those in the articles that validated and normed the measures in military samples (De La Rosa et al., 2016; Johnson et al., 2011; Prosek & Ponder, 2021). Additionally, the internal consistency was similar to previous studies. In the original article, Johnson et al. (2011) proposed a five-factor structure for the RSES-22, which was later established as a unidimensional assessment after further exploratory factor analysis (De La Rosa et al., 2016). Subsequently, confirmatory factor analyses with a treatment-seeking veteran population revealed that the RSES-22 demonstrated unacceptable model fit, whereas the RSES-4 demonstrated a good model fit (Prosek & Ponder, 2021). In both samples, the RSES-4 GFI, CFI, and TLI were all .944 or higher, whereas the RSES-22 GFI, CFI, and TLI were all .771 or lower. Additionally, criterion and convergent validity as measured by the PHQ-9, PCL-5, and GAD-7 in both samples were extremely close. Similarly, in this sample of treatment-seeking first responders, confirmatory factor analyses indicated an inadequate model fit for the RSES-22 and a good model fit for the RSES-4. Lastly, convergent and criterion validity were established with correlation analyses of the RSES-22 and RSES-4 with four other standardized assessment instruments (i.e., PHQ-9, PCL-5, GAD-7, SBQ-R). We concluded that among the first responder sample, the RSES-4 demonstrated acceptable psychometric properties, as well as criterion and convergent validity with other mental health variables (i.e., post-traumatic stress, anxiety, depression, and suicidal behavior).
Implications for Clinical Practice
First responders are a unique population and are regularly exposed to trauma (Donnelly & Bennett, 2014; Jetelina et al., 2020; Klimley et al., 2018; Weiss et al., 2010). Although first responders could potentially benefit from espousing resilience, they are often hesitant to seek mental health services (Crowe et al., 2017; Jones, 2017). The RSES-22 and RSES-4 were originally normed with military populations. The results of the current study indicated initial validity and reliability among a first responder population, revealing that the RSES-4 could be useful for counselors in assessing resilience.
It is important to recognize that first responders have perceived coping with traumatic stress as an individual process (Crowe et al., 2017) and may believe that seeking mental health services is counter to the emotional and physical training expectations of the profession (Crowe et al., 2015). Therefore, when first responders seek mental health care, counselors need to be prepared to provide culturally responsive services, including population-specific assessment practices and resilience-oriented care.
Jones (2017) encouraged a comprehensive intake interview and battery of appropriate assessments be conducted with first responder clients. Counselors need to balance the number of intake questions while responsibly assessing for mental health comorbidities such as post-traumatic stress, anxiety, depression, and suicidality. The RSES-4 provides counselors a brief, yet targeted assessment of resilience.
Part of what cultural competency entails is assessing constructs (e.g., resilience) that have been shown to be a protective factor against PTSD among first responders (Klimley et al., 2018). Since the items forming the RSES-4 were developed to highlight the positive characteristics of coping (Johnson et al., 2011), rather than a deficit approach, this aligns with the grounding of the counseling profession. It is also congruent with first responders’ perceptions of resilience. Indeed, in a content analysis of focus group interviews with first responders, participants defined resilience as a positive coping strategy that involves emotional regulation, perseverance, personal competence, and physical fitness (Crowe et al., 2017).
The RSES-4 is a brief, reliable, and valid measure of resilience with initial empirical support among a treatment-seeking first responder sample. In accordance with the ACA (2014) Code of Ethics, counselors are to administer assessments normed with the client population (E.8.). Thus, the results of the current study support counselors’ use of the measure in practice. First responder communities are facing unprecedented work tasks in response to COVID-19. Subsequently, their mental health might suffer (Centers for Disease Control and Prevention, 2020) and experts have recommended promoting resilience as a protective factor for combating the negative mental health consequences of COVID-19 (Chen & Bonanno, 2020). Therefore, the relevance of assessing resilience among first responder clients in the current context is evident.
Limitations and Future Research
This study is not without limitations. The sample of first responders was homogeneous in terms of race, ethnicity, and gender. Subsamples of first responders (i.e., LEO, EMT, fire rescue) were too small to conduct within-group analyses to determine if the factor structure of the RSES-22 and RSES-4 would perform similarly. Also, our sample of first responders included two emergency dispatchers. Researchers reported that emergency dispatchers should not be overlooked, given an estimated 13% to 15% of emergency dispatchers experience post-traumatic symptomatology (Steinkopf et al., 2018). Future researchers may develop studies that further explore how, if at all, emergency dispatchers are represented in first responder research.
Furthermore, future researchers could account for first responders who have prior military service. In a study of LEOs, Jetelina et al. (2020) found that participants with military experience were 3.76 times more likely to report mental health concerns compared to LEOs without prior military affiliation. Although we reported the prevalence rate of prior military experience in our sample, the within-group sample size was not sufficient for additional analyses. Finally, our sample represented treatment-seeking first responders. Future researchers may replicate this study with non–treatment-seeking first responder populations.
Conclusion
First responders are at risk for sustaining injuries, experiencing life-threatening events, and witnessing harm to others (Lanza et al., 2018). The nature of their exposure can be repeated and cumulative over time (Donnelly & Bennett, 2014), indicating an increased risk for post-traumatic stress, anxiety, and depressive symptoms, as well as suicidal behavior (Jones et al., 2018). Resilience is a promising protective factor that promotes wellness and healthy coping among first responders (Wild et al., 2020), and counselors may choose to routinely measure for resilience among first responder clients. The current investigation concluded that among a sample of treatment-seeking first responders, the original factor structure of the RSES-22 was unstable, although it demonstrated good reliability and validity. The adapted version, RSES-4, demonstrated good factor structure while also maintaining acceptable reliability and validity, consistent with studies of military populations (De La Rosa et al., 2016; Johnson et al., 2011; Prosek & Ponder, 2021). The RSES-4 provides counselors with a brief and strength-oriented option for measuring resilience with first responder clients.
Conflict of Interest and Funding Disclosure
The authors reported no conflict of interest
or funding contributions for the development
of this manuscript.
References
American Counseling Association. (2014). ACA code of ethics.
American Psychiatric Association. (2013). Diagnostic and statistical manual of mental disorders (5th ed.).
Antony, J., Brar, R., Khan, P. A., Ghassemi, M., Nincic, V., Sharpe, J. P., Straus, S. E., & Tricco, A. C. (2020). Interventions for the prevention and management of occupational stress injury in first responders: A rapid overview of reviews. Systematic Reviews, 9(121), 1–20. https://doi.org/10.1186/s13643-020-01367-w
Blevins, C. A., Weathers, F. W., Davis, M. T., Witte, T. K., & Domino, J. L. (2015). The Posttraumatic Stress Disorder Checklist for DSM-5 (PCL-5): Development and initial psychometric evaluation. Journal of Traumatic Stress, 28(6), 489–498. https://doi.org/10.1002/jts.22059
Burnett, H. J., Jr. (2017). Revisiting the compassion fatigue, burnout, compassion satisfaction, and resilience connection among CISM responders. Journal of Police Emergency Response, 7(3), 1–10. https://doi.org/10.1177/2158244017730857
Centers for Disease Control and Prevention. (2020, June 30). Coping with stress. https://www.cdc.gov/coronavirus/2019-ncov/daily-life-coping/managing-stress-anxiety.html
Chen, S., & Bonanno, G. A. (2020). Psychological adjustment during the global outbreak of COVID-19: A resilience perspective. Psychological Trauma: Theory, Research, Practice, and Policy, 12(S1), S51–S54. https://doi.org/10.1037/tra0000685
Christopher, M. S., Hunsinger, M., Goerling, R. J., Bowen, S., Rogers, B. S., Gross, C. R., Dapolonia, E., & Pruessner, J. C. (2018). Mindfulness-based resilience training to reduce health risk, stress reactivity, and aggression among law enforcement officers: A feasibility and preliminary efficacy trial. Psychiatry Research, 264, 104–115. https://doi.org/10.1016/j.psychres.2018.03.059
Crowe, A., Glass, J. S., Lancaster, M. F., Raines, J. M., & Waggy, M. R. (2015). Mental illness stigma among first responders and the general population. Journal of Military and Government Counseling, 3(3), 132–149. http://mgcaonline.org/wp-content/uploads/2013/02/JMGC-Vol-3-Is-3.pdf
Crowe, A., Glass, J. S., Lancaster, M. F., Raines, J. M., & Waggy, M. R. (2017). A content analysis of psychological resilience among first responders. SAGE Open, 7(1), 1–9. https://doi.org/10.1177/2158244017698530
De La Rosa, G. M., Webb-Murphy, J. A., & Johnston, S. L. (2016). Development and validation of a brief measure of psychological resilience: An adaptation of the Response to Stressful Experiences Scale. Military Medicine, 181(3), 202–208. https://doi.org/10.7205/MILMED-D-15-00037
Dimitrov, D. M. (2012). Statistical methods for validation of assessment scale data in counseling and related fields. American Counseling Association.
Donnelly, E. A., & Bennett, M. (2014). Development of a critical incident stress inventory for the emergency medical services. Traumatology, 20(1), 1–8. https://doi.org/10.1177/1534765613496646
Greinacher, A., Derezza-Greeven, C., Herzog, W., & Nikendei, C. (2019). Secondary traumatization in first responders: A systematic review. European Journal of Psychotraumatology, 10(1), 1562840. https://doi.org/10.1080/20008198.2018.1562840
Gutierrez, P. M., Osman, A., Barrios, F. X., & Kopper, B. A. (2001). Development and initial validation of the Self-Harm Behavior Questionnaire. Journal of Personality Assessment, 77(3), 475–490. https://doi.org/10.1207/S15327752JPA7703_08
Jetelina, K. K., Mosberry, R. J., Gonzalez, J. R., Beauchamp, A. M., & Hall, T. (2020). Prevalence of mental illnesses and mental health care use among police officers. JAMA Network Open, 3(10), 1–12. https://doi.org/10.1001/jamanetworkopen.2020.19658
Johnson, D. C., Polusny, M. A., Erbes, C. R., King, D., King, L., Litz, B. T., Schnurr, P. P., Friedman, M., Pietrzak, R. H., & Southwick, S. M. (2011). Development and initial validation of the Response to Stressful Experiences Scale. Military Medicine, 176(2), 161–169. https://doi.org/10.7205/milmed-d-10-00258
Jones, S. (2017). Describing the mental health profile of first responders: A systematic review. Journal of the American Psychiatric Nurses Association, 23(3), 200–214. https://doi.org/10.1177/1078390317695266
Jones, S., Nagel, C., McSweeney, J., & Curran, G. (2018). Prevalence and correlates of psychiatric symptoms among first responders in a Southern state. Archives of Psychiatric Nursing, 32(6), 828–835. https://doi.org/10.1016/j.apnu.2018.06.007
Joyce, S., Tan, L., Shand, F., Bryant, R. A., & Harvey, S. B. (2019). Can resilience be measured and used to predict mental health symptomology among first responders exposed to repeated trauma? Journal of Occupational and Environmental Medicine, 61(4), 285–292. https://doi.org/10.1097/JOM.0000000000001526
Kleim, B., & Westphal, M. (2011). Mental health in first responders: A review and recommendation for prevention and intervention strategies. Traumatology, 17(4), 17–24. https://doi.org/10.1177/1534765611429079
Klimley, K. E., Van Hasselt, V. B., & Stripling, A. M. (2018). Posttraumatic stress disorder in police, firefighters, and emergency dispatchers. Aggression and Violent Behavior, 43, 33–44.
https://doi.org/10.1016/j.avb.2018.08.005
Kroenke, K., Spitzer, R. L., & Williams, J. B. W. (2001). The PHQ-9: Validity of a brief depression severity measure. Journal of General Internal Medicine, 16, 606–613. https://doi.org/10.1046/j.1525-1497.2001.016009606.x
Lanza, A., Roysircar, G., & Rodgers, S. (2018). First responder mental healthcare: Evidence-based prevention, postvention, and treatment. Professional Psychology: Research and Practice, 49(3), 193–204. https://doi.org/10.1037/pro0000192
Lee, J.-S., Ahn, Y.-S., Jeong, K.-S. Chae, J.-H., & Choi, K.-S. (2014). Resilience buffers the impact of traumatic events on the development of PTSD symptoms in firefighters. Journal of Affective Disorders, 162, 128–133. https://doi.org/10.1016/j.jad.2014.02.031
Lewis, G. B., & Pathak, R. (2014). The employment of veterans in state and local government service. State and Local Government Review, 46(2), 91–105. https://doi.org/10.1177/0160323X14537835
McCanlies, E. C., Gu, J. K., Andrew, M. E., Burchfiel, C. M., & Violanti, J. M. (2017). Resilience mediates the relationship between social support and post-traumatic stress symptoms in police officers. Journal of Emergency Management, 15(2), 107–116. https://doi.org/10.5055/jem.2017.0319
National Institute of Mental Health. (2017). Post-traumatic stress disorder. https://www.nimh.nih.gov/health/statistics/post-traumatic-stress-disorder-ptsd.shtml
Osman, A., Bagge, C. L., Gutierrez, P. M., Konick, L. C., Kopper, B. A., & Barrios, F. X. (2001). The Suicidal Behaviors Questionnaire–revised (SBQ-R): Validation with clinical and nonclinical samples. Assessment, 8(4), 443–454. https://doi.org/10.1177/107319110100800409
Prosek, E. A., & Ponder, W. N. (2021). Validation of the Adapted Response to Stressful Experiences Scale (RSES-4) among veterans [Manuscript submitted for publication].
Spitzer, R. L., Kroenke, K., Williams, J. B. W., & Löwe, B. (2006). A brief measure for assessing generalized anxiety disorder (The GAD-7). Archives of Internal Medicine, 166(10), 1092–1097.
https://doi.org/10.1001/archinte.166.10.1092
Steinkopf, B., Reddin, R. A., Black, R. A., Van Hasselt, V. B., & Couwels, J. (2018). Assessment of stress and resiliency in emergency dispatchers. Journal of Police and Criminal Psychology, 33(4), 398–411.
https://doi.org /10.1007/s11896-018-9255-3
Substance Abuse and Mental Health Services Administration. (2018, May). First responders: Behavioral health concerns, emergency response, and trauma. Disaster Technical Assistance Center Supplemental Research Bulletin. https://www.samhsa.gov/sites/default/files/dtac/supplementalresearchbulletin-firstresponders-may2018.pdf
Weiss, D. S., Brunet, A., Best, S. R., Metzler, T. J., Liberman, A., Pole, N., Fagan, J. A., & Marmar, C. R. (2010). Frequency and severity approaches to indexing exposure to trauma: The Critical Incident History Questionnaire for police officers. Journal of Traumatic Stress, 23(6), 734–743.
https://doi.org/10.1002/jts.20576
White, B., Driver, S., & Warren, A. M. (2010). Resilience and indicators of adjustment during rehabilitation from a spinal cord injury. Rehabilitation Psychology, 55(1), 23–32. https://doi.org/10.1037/a0018451
Wild, J., El-Salahi, S., Degli Esposti, M., & Thew, G. R. (2020). Evaluating the effectiveness of a group-based resilience intervention versus psychoeducation for emergency responders in England: A randomised controlled trial. PLoS ONE, 15(11), e0241704. https://doi.org/10.1371/journal.pone.0241704
Windle, G., Bennett, K. M., & Noyes, J. (2011). A methodological review of resilience measurement scales. Health and Quality of Life Outcomes, 9, Article 8, 1–18. https://doi.org/10.1186/1477-7525-9-8
Warren N. Ponder, PhD, is Director of Outcomes and Evaluation at One Tribe Foundation. Elizabeth A. Prosek, PhD, NCC, LPC, is an associate professor at Penn State University. Tempa Sherrill, MS, LPC-S, is the founder of Stay the Course and a volunteer at One Tribe Foundation. Correspondence may be addressed to Warren N. Ponder, 855 Texas St., Suite 105, Fort Worth, TX 76102, warren@1tribefoundation.org.
Mar 30, 2018 | Volume 8 - Issue 1
Danielle Pester, A. Stephen Lenz, Joshua C. Watson, Julia Dell’Aquila, Anthony Nkyi
As the counseling profession continues its globalization onto Ghanaian college campuses, there is an increased need for psychometric assessments that support programming and interventions that promote degree matriculation and general student well-being. A sample of 696 young adult Ghanaian college students completed the Inventory of New College Student Adjustment (INCA) and related measures to estimate evidence of internal structure and relationships with conceptually related constructs. Confirmatory factor analyses were completed and inspection of fit indices revealed strong evidence for internal structure, and bivariate correlations indicated statistically significant positive associations with related medium effect sizes between the INCA subscales (Supportive Network and Belief in Self) and related measures. Implications for use of the INCA to support the professional activities of Ghanaian counselors working on college campuses are provided.
Keywords: Ghanaian counselors, college student adjustment, globalization, psychometric inventory, assessment
Higher education in Ghana has experienced tremendous growth over the past two decades, increasing access to institutions of higher education and student enrollment. In 2012, there were 138 accredited higher education institutions throughout Ghana, including public and private institutions, polytechnics, and training colleges (Atuahene, 2013; National Council for Tertiary Education [NCTE], 2014). This is an exponential degree of growth when compared to the existence of only three public universities in Ghana at the close of the 1990s (Atuahene, 2013). Although access and participation in university education has grown rapidly, the proportion of enrolled students versus those eligible to be enrolled remains low. According to the United Nations Educational, Scientific and Cultural Organization (2017), the percentage of enrolled students compared to those eligible to be enrolled in higher education in Ghana for 2015 was only 16.23%, indicating inadequate pre-college academic preparation, lack of affordability, low retention rates, and inadequate supports once enrolled (Atuahene, 2012). With its higher education system facing such challenges, resources and tools that can assist Ghanaian higher education institutions meet student needs as they enter university life, adjust to the unique set of demands, and access existing supports are imperative.
Because the demand for higher education in Ghana has traditionally been greater than its supply, most of the available resources have been focused on the expansion of facilities rather than the improvement of student experiences that may promote university persistence and degree matriculation. Only in recent years has the NCTE begun to rate institutions on the quality and relevance of their academic programs. Atuahene (2012) identified several distinctive factors associated with Ghanaian student dropout, including: (a) inadequate financial support for low income students, (b) student socioeconomic and geographic background, (c) student pre-college academic preparation, (d) unfavorable institutional policies and practices, and (e) a lack of academic advising. With these barriers in mind, there is currently an opportunity in Ghanaian higher education to develop resources that can support student adjustment and academic persistence.
Researchers (e.g., Carter, Locks, & Winkle-Wagner, 2013; Gray, Vitak, Easton, & Ellison, 2013; Pascarella & Terenzini, 2005; Robbins, Oh, Le, & Button, 2009) have found first-year adjustment to an academic setting to be a crucial component in student retention. Furthermore, they have found that positive adjustment within the first year of college can significantly impact a student’s academic persistence to degree completion. Andoh-Arthur, Asante, and Osafo (2015) studied the help-seeking behaviors of Ghanaian university students and found that the first-year student population was least likely to engage in help-seeking behaviors. They attributed this to the students’ unfamiliarity with their new identity as university students. Knowing this, Ghanaian students’ first year of university experience is a crucial time for university support personnel to proactively engage students regarding college adjustment issues. The capacity to identify new university students who are struggling to adjust to college life and who also may be at a higher risk for attrition is essential for Ghanaian university personnel as they seek to improve university retention rates.
Globalization of Counseling and Its Role in University Settings
The welcome statement of NBCC International proposes an organizational intention to increase the “availability of competent, reliable services to any part of the world that indicates an interest in acquiring them . . . with the utmost care and respect for the social, cultural, political, and economic realities of the various areas where we are invited” (Clawson, 2011, para. 2). Lorelle, Byrd, and Crockett (2012) identified the globalization of counseling as an inevitability, wherein professional counseling activities are progressively transitioning from a Western-based practice to one that gives international communities the opportunity for transformation as well. Lorelle et al. suggested that as the counseling profession is introduced on a local level, opportunities emerge for adaptation to local cultures and new contexts that yield new ways of understanding culturally defined standards of care. Among the many international settings adopting the values and activities synonymous with the counseling profession, Ghana appears poised to increase the capacity and scope of counseling activities through meaningful placement of services on university campuses.
Quarshie, Annor, Tagoe, Osei-Poku, and Andoh-Arthur (2016) identified a growing population of mental health professionals within the country of Ghana. This expansion of service provider capacity has been positively correlated with growth in the Ghanaian economy and represents a commitment to developing public mental health infrastructure using existing resources and expanding capacity over time (Ghana Health Professions Regulatory Bodies Act 857, 2013). Quarshie et al.’s (2016) analyses also detected that the majority of Ghanaian mental health professionals are housed on college, polytechnic, and university campuses. Situating these providers within these settings not only provides them support for their professional preparation programs, but also provides proximal contact with students who may be experiencing mental health symptoms while attempting to adjust to new demands within university settings. This action has important consequences for both the globalization of the counseling profession and the promotion of optimal development, degree matriculation, and access to a more equitable life for Ghanaian students. However, evidence-supported interventions require evidence-supported assessments that are population-specific, and currently there is a paucity of such assessments that can be utilized by mental health professionals to understand the adjustment experiences of students at Ghanaian universities.
Rationale and Purpose of the Study
Given that one aspect of counselor identity is the use of evidence-supported assessment practices, and another is evidence-supported intervention and programming (American Counseling Association, 2014; Lorelle et al., 2012), there is a call to complete activities to support the actions of Ghanaian mental health professionals charged with promoting adjustment among local university students. The Inventory of New College Student Adjustment (INCA; Watson & Lenz, 2017) is one viable instrument for assessing college student adjustment that is free to use and has yielded promising psychometric properties among ethnically diverse samples within the United States. It has been identified as a resource to help determine the appropriate support services needed for university students, as well as a resource to assess the overall effectiveness of campus initiatives focused on student adjustment. Although the INCA could be a valuable tool to address the current needs and trends in Ghanaian higher education, the degree of validity of INCA scores for a Ghanaian university student population is currently unknown. Therefore, the purpose of this study was to evaluate the transferability of validity evidence for scores on the INCA to a sample of Ghanaian students. Specifically, we intended to identify the degree of evidence related to internal structure of the INCA scores and their relationships with conceptually related variables.
Method
Participant Characteristics
Six hundred ninety-six Ghanaian college students (435 male [63%], 237 female [34%], 24 did not report gender [3%]), the majority of whom were young adults (M age = 22.45 years; SD = 4.37) completing undergraduate coursework at one large university in Ghana, Africa, participated in this study.
Measurement of Constructs
Inventory of New College Student Adjustment. The INCA (Watson & Lenz, 2017) was developed to assess the adjustment difficulties experienced by first-year college students and was normed using an ethnically diverse sample of 474 freshmen students in the United States. The INCA is a 14-item instrument using a 4-point Likert scale to assess participant responses from 1 (strongly disagree) to 4 (strongly agree). Scores can range from 14 to 56, with higher scores indicating higher levels of college adjustment. The 6-item Supportive Network subscale includes items such as “My friends support me as I work toward my goals” and “My family’s support makes me feel stronger.” The 8-item Belief in Self subscale includes items such as “My study habits are effective” and “I know what I will do after graduation.” Initial psychometric testing demonstrates good alpha reliability coefficients for scores on INCA subscales ranging from .77 (Belief in Self) to .83 (Supportive Network), indicating good internal consistency. Moreover, our sample reported alpha reliability coefficients of .74 for both the Belief in Self subscale and the Supportive Network subscale.
The Multidimensional Scale of Perceived Social Support. The Multidimensional Scale of Perceived Social Support (MSPSS; Zimet, Dahlem, Zimet & Farley, 1988) was developed to assess an individual’s perception of social support from family, friends, and significant others. Each of these sources of social support is considered a distinct subgrouping and is assessed individually. The MSPSS was normed using a subject pool of 275 undergraduate students in the United States with a nearly equal sample of male and female students (Zimet, et al., 1988). After further psychometric testing, reliability has been established for diverse samples beyond the original norming group (Stanley, Beck, & Zebb, 1998). The MSPSS is a 12-item instrument using a 7-point Likert-scale to assess participant responses from 1 (very strongly disagree) to 7 (very strongly agree). Scores can range from 12 to 84, with higher scores representing higher levels of perceived social support. For the purposes of this study, we used the Family Relationships subscale and Relationships with Friends subscale. The 4-item Family Relationships subscale includes items such as “My family really tries to help me” and “I get the emotional help and support I need from my family.” The 4-item Relationships with Friends subscale includes items such as “My friends really try to help me” and “I can count on my friends when things go wrong.” Zimet et al. (1988) reported high Cronbach’s alpha coefficients for scores on MSPSS subscales ranging from .85–.91, indicating good internal consistency. The reliability of the total scale for the initial sample was .88. Additionally, our sample reported coefficients ranging from .81 for the Family Relationships subscale and .88 for the Relationships with Friends subscale.
College Self-Efficacy Inventory. The College Self-Efficacy Inventory (CSEI; Solberg, O’Brien, Villareal, Kennel, & Davis, 1993) was developed to assess a student’s confidence in their ability to successfully complete college-related tasks. Originally developed to measure college self-efficacy in Hispanic college students, CSEI data has established reliability beyond the initial norming population to also include ethnically diverse college students (Gore Jr., Leuwerke, & Turley, 2005). The CSEI is a 20-item instrument using a 10-point scale to assess a participant’s confidence in their ability to successfully complete a task from 1 (not at all confident) to 10 (extremely confident). Scores can range from 20 to 200, with higher scores indicating higher levels of confidence in one’s ability to successfully complete college-related tasks. The 20-item scale includes items such as “Make new friends at college,” “Talk to university staff,” and “Take good class notes” (Barry & Finney, 2009). Gore et al. (2006) reported Cronbach’s alpha coefficients for scores on the CSEI subscales ranging from .62–.89. The reliability of the CSEI for the initial sample was .93 (Solberg et al., 1993). Additionally, we observed a Cronbach’s alpha coefficient of .88 for our sample.
Procedure
After ethical review board approval, students registered in classes at one large university in Ghana were asked to participate in this study. A survey administrator, who was not the course instructor, shared the opportunity to participate in this study with students and disseminated an information sheet explaining the purpose, processes, and voluntary nature of the study. After having time to review the information sheet, the students choosing to participate in the study were given a packet including a demographic questionnaire, the INCA, the MSPSS, and the CSEI. All measures except for the demographic questionnaire were counter-balanced in an effort to control for random responding, order effect, and fatigue. Participants filled out hard copy surveys in class and turned them in to the survey administrator, who supplied them to the authors. Participant answers to the survey packet were entered into an SPSS spreadsheet. After all data was documented, the original hard copy surveys were securely destroyed.
Data Analysis
Statistical power analysis. We conducted a power analysis to determine the suitability of our sample size for identifying model fit using the criteria outlined by Stevens (2009): n/p ≥ 30. Using this standard, our largest scale (Belief in Self), consisting of eight items, would necessitate a sample size of at least 240. With a sample size of 696 (i.e., 87 participants per item), we considered our sample size sufficient for making statistical inferences about model fit. We also acknowledge that this model is over-powered for hypothesis testing and may lead to type I error. Therefore, when interpreting analyses, a greater emphasis was placed on model fit indices over p-values for χ2 tests.
Preliminary data analysis. The dataset was analyzed for missing values prior to performing statistical analyses. A small percentage of missing values (684 out of 71,100; .009%) was detected, but no identifiable pattern within these absent values was present. We used the series mean imputation function in IBM SPSS, Version 23, to replace all missing values.
Evidence regarding internal structure. We analyzed model fit for the INCA subscales using the SPSS Analysis of Moment Structures Software, Version 22. We conducted our analyses of the INCA subscale factor structures based on the initial factor structure emerging from the analyses completed by Watson and Lenz (2017). Initially, we interpreted the C-minimum/degrees of freedom (CMIN/DF), p-values, root mean residual (RMR), goodness of fit index (GFI), comparative fit index (CFI), Tucker-Lewis index (TLI), and root mean square error of approximation (RMSEA) metrics of model fit. Standards presented by Dimitrov (2012) were used to interpret these values with criteria for a strong model fit represented by CMIN/DF < 2, p > .05, RMR < .08, GFI > .90, CFI > .90, TLI > .90, and RMSEA < .10. When model fit proved inconsistent with these standards, modification indices were evaluated to determine items with potential covaried error. Covarying items provides a scenario within the factorial model wherein two items share their assumed variance. If such instances were identified, the model was computed again to re-inspect fit indices. If a factor model continued to have an inadequate fit, we inspected individual item correlation loadings and considered items for removal from the model. Items were removed if correlation coefficients were found to be less than .70.
Evidence regarding relationships with conceptually related constructs. Bivariate correlations were computed between scores on the INCA, MSPSS, and CSEI to depict degree of convergent validity between scores on the INCA subscales (Supportive Network and Belief in Self) with conceptually related constructs of perceived social support and academic self-concept, via the MSPSS and CSEI, respectively. Pearson’s correlation coefficients were interpreted as small (.10), medium (.30), or large (.50) based on the conventions reported by Swank and Mullen (2017) and evaluated at the .05 level of statistical significance.
Results
All alpha coefficients, descriptive statistics, and bivariate correlations for variables included within the analyses can be found in Table 1.
Table 1
Alpha Coefficients, Descriptive Statistics, and Bivariate Correlations for Variables Included Within Analyses.
Scale-Construct |
α |
M |
SD |
1 |
2 |
3 |
INCA – Belief in Self |
.74 |
23.31 |
3.32 |
44* |
|
.34* |
INCA – Supportive Network |
.74 |
17.44 |
2.65 |
.44* |
|
1. MSPSS – Family Relationships |
.88 |
20.02 |
6.49 |
|
|
2. MSPSS – Relationships with Friends |
.81 |
16.70 |
5.91 |
|
|
3. CSEI – College Self-Efficacy |
.88 |
36.77 |
14.69
|
|
|
Note. * indicates statistical significance at .01 level |
Evidence Regarding Internal Structure
INCA – Belief in Self. The primary analysis of the Belief in Self subscale was significant for the hypothesized model, χ2(20) = 124.51, p < .01, and was suggestive of an unacceptable fit for the data: CMIN/DF = 6.22, RMR = .02, GFI = .95, CFI = .88, RMSEA = .08. After deleting item 6 and pairing the error terms for items 2 and 5 (“Past experiences help me cope with the demands of university life” and “Challenging courses make me a better student”) and 3 and 4 (“I believe I handle adversity well” and “My classmates value my opinions”), a good model fit emerged for scores on the Belief in Self subscale: χ2(12) = 28.58, p < .01. This finding was additionally supported by the fit indices: CMIN/DF = 2.38, RMR = .01, GFI = .98, CFI = .98, RMSEA = .04. Inspection of the alpha coefficient for scores for this sample (α = .74) was within the good range, indicating an acceptable degree of consistency and precision suitable for social sciences research activities.
INCA – Supportive Network. The primary analysis of the Supportive Network subscale was significant for the hypothesized model, χ2(9) = 102.28, p < .01, and was suggestive of an unacceptable fit for the data: CMIN/DF = 11.37, RMR = .03, GFI = .95, CFI = .80, RMSEA = .12. After pairing error terms for items 1 and 3 (“My friends support me as I work toward my goals” and “My friends help me to grow in important ways”) and items 4 and 6 (“My family’s support makes me feel stronger” and “I can be real with at least a few of my friends”), a good model fit emerged for scores on the Supportive Network subscale: χ2(7) = 14.03, p = .08. This finding was additionally supported by the fit indices: CMIN/DF = 3.41, RMR = .01, GFI = .98, CFI = .96, RMSEA = .05. Inspection of the alpha coefficient for scores for this sample (α = .74) was within the marginal range, indicating an acceptable degree of consistency and precision suitable for social sciences research activities.
Evaluation of Conceptually Related Measures
Family Relationships. The primary analysis of the Family Relationships subscale of the MSPSS was significant for the hypothesized model, χ2(2) = 45.47, p < .01, and was suggestive of an unacceptable fit for the data: CMIN/DF = 22.73, RMR = .10, GFI = .96, CFI = .97, RMSEA = .17. After pairing the error terms for items 3 and 4 (“I can talk about my problems with my family” and “My family is willing to help me make decisions”) a good model fit emerged for scores on the Family Relationships subscale: χ2(1) = 9.21, p <.01. This finding was additionally supported by the fit indices: CMIN/DF = 9.21, RMR = .04, GFI = .99, CFI = .99, RMSEA = .10. Inspection of the alpha coefficient for scores for this sample (α = .88) was within the good range, indicating an acceptable degree of consistency and precision suitable for social sciences research activities.
Relationships with Friends. The primary analysis of the Relationships with Friends subscale of the MSPSS was significant for the hypothesized model, χ2(2) = 49.52, p < .01, and was suggestive of an unacceptable fit for the data: CMIN/DF = 24.76, RMR = .15, GFI = .96, CFI = .95, RMSEA = .18. After pairing the error terms for items 1 and 2 (“My friends really try to help me” and “I can count on my friends when things go wrong”), a good model fit emerged for scores on the Relationships with Friends subscale: χ2(1) = 1.43, p = .23. This finding was additionally supported by the fit indices: CMIN/DF = 1.43, RMR = .02, GFI = .99, CFI = 1, RMSEA = .02. Inspection of the alpha coefficient for score for this sample (α = .81) was within the good range, indicating an acceptable degree of consistency and precision suitable for social sciences research activities.
College Self-Efficacy. The primary analysis of the College Self-Efficacy subscale of the CSEI was significant for the hypothesized model, χ2(9) = 66.70, p < .01, and was suggestive of an unacceptable fit for the data: CMIN/DF = 7.41, RMR = .34, GFI = .97, CFI = .98, RMSEA = .09. After pairing the error terms for items 1 and 2 (“Manage time effectively” and “Research a term paper”) and 3 and 5 (“Do well on your exams” and “Understand your textbooks”), a good model fit emerged for scores on the College Self-Efficacy subscale: χ2(7) = 22.45, p <.01. This finding was additionally supported by the fit indices: CMIN/DF = 3.20, RMR = .10, GFI = .98, CFI = .99, RMSEA = .05. Inspection of the alpha coefficient for scores for this sample (α = .88) was within the good range, indicating an acceptable degree of consistency and precision suitable for social sciences research activities.
Evidence Regarding Relationships With Conceptually Related Constructs
Bivariate correlation analysis of scores on the INCA Belief in Self subscale and CSEI resulted in a statistically significant positive relationship (r = .34, p < .01) indicative of a medium effect size. The correlation analysis of scores on the INCA Supportive Network subscale and MSPSS Family Relationships and Relationships with Friends subscales also resulted in statistically significant positive relationships (r = .448, p < .01, r = .448, p < .01, respectively) indicative of medium effect sizes. The strong positive relationships between scores on the two INCA subscales and conceptually related constructs are suggestive of support for convergent validity wherein the scores on the INCA tended to increase while scores on related measures increased too. Taken together, students who reported a greater belief in self also tended to report a greater sense of college self-efficacy. Similarly, participants who reported a greater belief in self during the first year of transition to college life also tended to report higher scores, indicating strong relationships with friends and family.
Discussion
The purpose of this study was to evaluate the validity evidence for the INCA using a Ghanaian college student population, with the hope that the instrument could be used by mental health professionals working in Ghanaian universities. Given the robust nature of our findings, we are heartened by the potential for the INCA and other emerging assessments to contribute to evidence-supported practices for optimal development and adjustment among students at Ghanaian universities. In light of our findings, several considerations warrant discussion.
Foremost, the INCA has potential uses that could address some of the most prominent issues facing higher education in Ghana today, particularly low matriculation rates. As the NCTE begins to rate institutions on the quality and relevance of their academic programs, the INCA can be used by university personnel to assess student adjustment so that necessary changes to student affairs programming can be made to improve the adjustment experiences of Ghanaian college students. Specifically, the INCA can be used by university personnel to gain a better understanding of the adjustment experiences of their first-year college students. This understanding can have important implications for program development at Ghanaian higher education institutions. As university personnel better understand the adjustment experiences of their first-year students, they can create programs that are more specialized to meet the needs of the Ghanaian student population, improve retention rates, and increase matriculation. Such activities have auspicious implications for not only promoting optimal development proximally, but encouraging access to a more equitable life, one characterized by fewer disparities than individuals within the emerging Ghanaian economy who do not have similar educational preparation and training.
Additionally, scores on the INCA can support early identification of first-year students who are struggling to adjust to university life. Because first-year students are least likely to engage in help-seeking behaviors (Andoh-Arthur et al., 2015), university personnel can develop proactive strategies to support struggling students and provide psychoeducation about the benefits of help-seeking behaviors. Such activities may include designing early detection protocols within orientation activities or integrating screening and referral within initial coursework activities.
In the cases of both program development and early identification, scores on the INCA have potential for evaluating outcomes in a manner that is culturally valid to a reasonable degree. Thus, the quantification of intervention outcomes by student affairs programmers and mental health professionals can provide an impetus for further understanding their students’ needs and the best strategies for meeting them. This is an important consideration in an era wherein Ghanaian mental health professionals are leveraging existing resources while extending their scope of influence within an emerging sociopolitical climate, which has expanded professional counseling activities through legislative action (Ghana Health Professions Regulatory Bodies Act 857, 2013). It is reasonable to conjecture that through the use of the INCA and other emerging assessments, the utilization and extension of personnel resources can not only be data-driven, but data-justified as well.
Finally, as the globalization of the counseling profession continues to be cultivated worldwide, it is important that counselors in international settings have valid psychometric tools that are population specific. Validation activities, such as the INCA project reported here, provide psychometrically robust assessments that Ghanaian mental health professionals can add to their growing corpus of resources. Although the use of assessment-based programming and outcome measurement do not define the whole of a counselor’s professional identity, it is a critical feature (American Counseling Association, 2014; Lorelle et al., 2012). Therefore, as the INCA and other assessments continue to be validated with Ghanaian student populations, the professionalization of Ghanaian mental health professionals grows lockstep.
Limitations and Recommendations for Future Research
Some important limitations and related recommendations for future research are indicated. First, although we sampled almost 700 Ghanaian students, the scope of our participant sample was limited to one campus. Therefore, we regard our findings as preliminary and most relevant to the student body from which they were affiliated. While it is reasonable that a substantial degree of validity generalization may be present, future studies completed at other Ghanaian universities are needed to estimate the transferability of INCA scores across regions. Second, internal consistency of INCA scores (α) were within the acceptable range (.70–.80), yet they did not reach a level that would warrant use for high stakes decision-making, such as program eligibility or dismissal. Further research evaluating content-oriented evidence (Lambie, Blount, & Mullen, 2017), cognitive processing, and response processes (Peterson, Peterson, & Powell, 2017) of INCA items and scores is needed to identify variables that may influence the reliability of items. It is possible that because INCA factors were developed from a Western theory of student adjustment, that consistency may be affected and indicative that some modification of item wording may be warranted (Lenz, Soler, Dell’Aquila, & Uribe, 2017). Thus, further evaluation related to cross-cultural adaptation and representation of constructs consisting within Ghanaian culture is warranted. Finally, this study only reported two sources of validity evidence. Although evidence across all sources of validity would not necessarily imply that INCA is inherently useful (Lenz & Wester, 2017), future research that elucidates INCA features associated with construct irrelevance and underrepresentation would further promote responsible testing and evaluation practice (Spurgeon, 2017).
Conclusion
In conclusion, this study evaluated the transferability of validity evidence for scores on the INCA to a sample of Ghanaian college students. The findings suggest the INCA is a valid psychometric assessment that has the potential to contribute to evidence-supported practices for optimal development and adjustment among students at Ghanaian universities. Specifically, the INCA can be used by Ghanaian university personnel to assess student adjustment, make any necessary changes to student affairs programming to improve the adjustment experiences of their college students, identify first-year students who are struggling to adjust to university life, and develop proactive strategies to support struggling students. Although initial results are promising, continued research is needed to validate the INCA at various universities across Ghana to continue to determine its degree of generalizability.
Conflict of Interest and Funding Disclosure
The authors reported no conflict of interest or funding contributions for the development of this manuscript.
References
American Counseling Association. (2014). 2014 ACA code of ethics. Retrieved from https://www.counseling.org/resources/aca-code-of-ethics.pdf
Andoh-Arthur, J., Asante, K. O., & Osafo, J. (2015). Determinants of psychological help-seeking intentions of university students in Ghana. International Journal for the Advancement of Counselling, 37, 330–345.
Atuahene, F. (2012). The impact of “tuition-paying” policy on retention and graduation rates at the University of Ghana. Journal of College Student Retention: Research, Theory & Practice, 14, 37–65.
Atuahene, F. (2013). Charting higher education development in Ghana: Growth, transformations, and challenges. In A. W. Wiseman & C. C. Wolhuter (Eds.), The development of higher education in Africa: Prospects and challenges (pp. 215–263). Bingley, UK: Emerald Group Publishing Limited.
Barry, C. L., & Finney, S. J. (2009). Can we feel confident in how we measure college confidence? A psychometric investigation of the College Self-Efficacy Inventory. Measurement and Evaluation in Counseling and Development, 42, 197–222. doi:10.1177.0748175609344095
Carter, D. F., Locks, A. M., & Winkle-Wagner, R. (2013). From when and where I enter: Theoretical and empirical considerations of minority students’ transition to college. In M. B. Paulson (Ed.), Higher education: Handbook of theory and research (Vol. 28; pp. 93–149). New York, NY: Agathon Press.
Clawson, T. W. (2011). Welcome to NBCC International. Retrieved from http://www.nbccinternational.org/Who_we_are/Welcome_Message
Dimitrov, D. M. (2012). Statistical methods for validation of assessment scale data in counseling and related fields. Alexandria, VA: American Counseling Association.
Ghana Health Professions Regulatory Bodies Act 857. (2013). Accra, Ghana: Ghana Publishing Company Limited, Assembly Press. Retrieved from http://www.pcghana.org/wp-content/uploads/2017/02/Health-
Professions-Regulatory-Bodies-Act.pdf
Gore Jr., P. A., Leuwerke, W. C., & Turley, S. E. (2005). A psychometric study of the College Self-Efficacy Inventory. Journal of College Student Retention: Research, Theory & Practice, 7, 227–244.
doi:10.2190/5CQF-F3P4-2QAC-GNVJ
Gray, R., Vitak, J., Easton, E. W., & Ellison, N. B. (2013). Examining social adjustment to college in the age of social media: Factors influencing successful transitions and persistence. Computers & Education, 67,
193–207. doi:10.1016/j.compedu.2013.02.021
Lambie, G. W., Blount, A. J., & Mullen, P. R. (2017). Establishing content-oriented evidence for psychological assessments. Measurement and Evaluation in Counseling and Development, 50, 210–216.
doi:10/1080/07481756.2017.1336930
Lenz, A. S., Soler, I. G., Dell’Aquila, J., & Uribe, P. M. (2017). Translation and cross-cultural adaptation of assessments for use in counseling research. Measurement and Evaluation in Counseling and Development, 50, 224–231. doi:10.1080/07481756.2017.1320947
Lenz, A. S., & Wester, K. L. (2017). Development and evaluation of assessments for counseling professionals. Measurement and Evaluation in Counseling and Development, 50, 201–209. doi:10.1080/07481756.2017.1361303
Lorelle, S., Byrd, R., & Crockett, S. (2012). Globalization and counseling: Professional issues for counselors. The Professional Counselor, 2, 115–123. doi:10.15241/sll.2.2.115
National Council for Tertiary Education. (2014). Annual report 2014. Retrieved from http://ncte.edu.gh/images/pdf/annual%20report%202014.pdf
Pascarella, E. T., & Terenzini, P. T. (2005). How college affects students: Volume 2: A third decade of research. San Francisco, CA: Jossey-Bass.
Peterson, C. H., Peterson, N. A., & Powell, K. G. (2017). Cognitive interviewing for item development: Validity evidence based on content and response processes. Measurement and Evaluation in Counseling and Development, 50, 217–223. doi:10.1080/07481756.2017.1339564
Quarshie, E. N. B., Annor, F., Tagoe, T., Osei-Poku, E., & Andoh-Arthur, J. (2016). Psychologists in Ghana: Analysis of the standing register. New Voices in Psychology, 12, 55–69.
Robbins, S. B., Oh, I. S., Le, H., & Button, C. (2009). Intervention effects on college performance and retention as mediated by motivational, emotional, and social control factors: Integrated meta-analytic path analyses. Journal of Applied Psychology, 94, 1163–1184. doi:10.1037/a0015738
Solberg, V. S., O’Brien, K., Villareal, P., Kennel, R., & Davis, B. (1993). Self-efficacy and Hispanic college students: Validation of the College Self-Efficacy Instrument. Hispanic Journal of Behavioral Sciences, 15, 80–95. doi:10.1177/07399863930151004
Spurgeon, S. L. (2017). Evaluating the unintended consequences of assessment practices: Construct irrelevance and construct underrepresentation. Measurement and Evaluation in Counseling and Development, 50, 275–281. doi:10.1080/07481756.2017.1339563
Stanley, M. A., Beck, J. G., & Zebb, B. J. (1998). Psychometric properties of the MSPSS in older adults. Aging & Mental Health, 2, 186–193. doi:10.1080/13607869856669
Stevens, J. P. (2009). Applied multivariate statistics for the social sciences (5th ed.). New York, NY: Routledge.
Swank, J. M., & Mullen, P. R. (2017). Evaluating evidence for conceptually related constructs using bivariate correlations. Measurement and Evaluation in Counseling and Development, 50, 270–274.
doi:10.1080/07481756.2017.1339562
United Nations Educational, Scientific and Cultural Organization. (2017). Ghana. Retrieved from http://uis.unesco.org/en/country/gh?theme=education-and-literacy
Watson, J. C., & Lenz, A. S. (2017). Development and evaluation of the Inventory for New College Student Adjustment. Manuscript submitted for publication.
Zimet, G. D., Dahlem, N. W., Zimet, S. G., & Farley, G. K. (1988). The multidimensional scale of perceived social support. Journal of Personality Assessment, 52, 30–41. doi:10.1207/s15327752jpa5201_2
Danielle Pester is a doctoral student at Texas A&M University-Corpus Christi. A. Stephen Lenz is an associate professor at Texas A&M University-Corpus Christi. Joshua C. Watson, NCC, is a professor at Texas A&M University-Corpus Christi. Julia Dell’Aquila is a doctoral student at Texas A&M University-Corpus Christi. Anthony Nkyi is a lecturer at the University of Cape Coast. Correspondence can be addressed to Danielle Pester, 6300 Ocean Drive, Corpus Christi, TX 78412, danielle.pester@tamucc.edu.
Mar 23, 2016 | Article, Volume 6 - Issue 1
Nicole A. Stargell, Victoria E. Kress, Matthew J. Paylo, Alison Zins
Excoriation disorder, sometimes colloquially referred to as skin picking disorder, is a newly added disorder in the Diagnostic and Statistical Manual of Mental Disorders (5th ed.; DSM-5; American Psychiatric Association [APA], 2013). Despite being a newly-classified DSM disorder, excoriation disorder is relatively common and affects between 1.4 and 5.4% of the general population (Grant et al., 2012). The purpose of this article is to provide professional counselors with a general understanding of how to assess, diagnose and treat excoriation disorder.
The prevalence of excoriation disorder may be underestimated, as it is sometimes overlooked, particularly because of comorbidity with other mental disorders (e.g., depression, anxiety, obsessive-compulsive disorder; Hayes, Storch, & Berlanga, 2009). Previously underestimated numbers of its prevalence also may be due to the covertness often associated with this disorder (Grant & Odlaug, 2009). Many people with excoriation disorder go to great lengths to hide their behavior from others (e.g., significant others, family members, health professionals) due to fear or embarassment.
Historically, excoriation disorder has been associated with obsessive-compulsive disorder (OCD), and it is now listed as a unique diagnosis in the obsessive-compulsive and related disorders section in the DSM-5 (Ravindran, da Silva, Ravindran, Richter, & Rector, 2009). According to the APA (2013), excoriation disorder involves the recurrent, excessive and often impulsive scratching, rubbing and picking of skin which leads to tissue damage and lesions. Those who have excoriation disorder frequently initiate attempts to eradicate these destructive behaviors, yet have difficulty doing so. In order for the diagnosis of excoriation disorder to be applied, individuals must experience clinically-significant distress or impairment in social, occupational or other important areas of functioning due to the routine nature of the skin picking behaviors (APA, 2013). Because of its physical manifestation, this phenomenon has frequently been discussed in medical research, but it is now receiving attention in mental health circles.
Etiology of Excoriation Disorder
Little is known about the etiology of excoriation disorder. Much of the current excoriation disorder research has been based on previous research conducted on trichotillomania. Excoriation disorder and trichotillomania are body-focused repetitive behaviors (BFRB) under the same DSM-5 classification, and the etiologies behind both disorders might be similar (Flessner, Berman, Garcia, Freeman, & Leonard, 2009). Most theorists suggest that excoriation disorder is rooted in both biological and psychological factors (Grant et al., 2012).
Biological factors related to excoriation disorder include genetic predispositions and neurological sensitivity to emotional stimuli, which result in emotional impulsivity and a need to self-soothe (Snorrason, Smári, & Ólafsson, 2011). In one study of 40 individuals who had excoriation disorder, 43% had a first-degree relative with the disorder (Neziroglu, Rabinowitz, Breytman, & Jacofsky, 2008). Specific genes (e.g., Hoxb8 and SAPAP3) have been identified as potential predictors of this disorder (Grant et al., 2012). In animal studies, mice with these genes engaged in excessive grooming to the point of skin lesions, behaviors similar to those of people who have excoriation disorder (Grant et al., 2012). Conversely, in another study, humans with the SAPAP3 gene only met criteria for excoriation disorder 20% of the time (Dufour et al., 2010). It is important to note that genetics appear to play a role in the development of excoriation disorder, but other factors contribute to the disorder’s etiology and maintenance as well (Grant et al., 2012; Lang et al., 2010).
In terms of psychological factors, skin picking behaviors help regulate uncomfortable emotions and can become a behaviorally-reinforced coping mechanism used to manage negative feelings (Lang et al., 2010). Some researchers suggest that excoriation disorder is rooted in higher levels of emotional impulsivity and that this characteristic supports and encourages the development of the disorder (Grant et al., 2012). Those with excoriation disorder experience obsessive thoughts about skin picking and engage in more impulsive, sensation-seeking behaviors (e.g., picking, rubbing) than those without the disorder (Snorrason et al., 2011). Those with excoriation disorder often have a greater difficulty with response inhibition and an increased difficulty suppressing an already initiated response as compared to control participants (Grant, Odlaug, & Chamberlain, 2011; Odlaug & Grant, 2010). For example, it might be more difficult for those with excoriation disorder to retract their hand if they already started reaching for an object to use to excoriate. This elevated level of impulsivity may be rooted in brain abnormalities; however, further research is necessary to clearly establish this connection (Grant et al., 2012).
Another common theory regarding the onset and maintenance of excoriation disorder is that skin picking behaviors can help regulate emotions and can become a behaviorally-reinforced coping mechanism used to manage elevated levels of anxiety, stress and arousal. Individuals who skin pick often display elevated stress responses to normal stimuli (Lang et al., 2010), and skin picking appears to temporarily sooth such stress. Additionally, obsessive thoughts about skin imperfections and anxiety over not picking can be temporarily relieved by completing the behaviors (Capriotti, Ely, Snorrason, & Woods, 2015). As such, there is a behavioral component—in addition to the genetic and biological components of the disorder—that must be considered when understanding the etiology, assessment, diagnosis and treatment of excoriation disorder.
Assessment and Diagnosis of Excoriation Disorder
The proposed etiologies (e.g., genetic predispositions, biological markers) and functions (e.g., soothing emotional reactivity, reducing obsessive thoughts) of excoriation disorder inform the diagnostic and assessment process. It is important that counselors have a thorough understanding of the DSM-5 criteria for excoriation disorder and understand that many clients with this disorder might hide physical markers and omit skin picking information unless asked directly (Grant & Odlaug, 2009). As such, counselors might use formal assessments, in addition to clinical judgment, in order to make an accurate diagnosis and best understand the client’s behaviors.
Assessment
A number of assessment tools can be used to assist in assessing, diagnosing and treating those who have excoriation disorder. Each measure can be utilized by counselors in developing a holistic conceptualization of the client and for engaging in differential diagnosis. Upon accurate diagnosis of excoriation disorder, assessment measures also can aid counselors in selecting appropriate treatment goals, interventions and modalities for each client, and they can be used to assess client behavior change.
Keuthen et al. (2001b) constructed three skin picking scales that can be used to assess excoriation disorder and aid in the assessment and treatment process. The first measure, the Skin Picking Scale (SPS), can be used to measure the client’s self-reported severity of skin picking behaviors. This measure consists of six items that relate to the frequency of picking urges, intensity of picking urges, time spent engaging in skin picking behaviors, interference of the behaviors in functioning, avoidance behaviors and the overall distress associated with the excoriation-related behaviors. Each item is assessed on a 5-point scale of 0 (none) to 4 (extreme), resulting in a range of total scores between 0 and 24. The SPS demonstrated high internal consistency with adequate convergent validity (Keuthen et al., 2001a). Pragmatically, this measure can be used to distinguish self-injurious skin picking from non-self-injurious skin picking. As treatment gains are made, corresponding scores should decrease.
The second measure is the Skin Picking Impact Scale (SPIS). The SPIS is a self-report questionnaire designed to assess the impacts or consequences of repetitive skin picking (e.g., negative self-evaluation, social interference; Keuthen et al., 2001a). Each of the scale’s 10 items are rated on a 6-point scale from 0 (none) to 5 (severe), resulting in a total score ranging from 0 to 50. The SPIS has high internal consistency (Keuthen et al., 2001a; Snorrason et al., 2013), and scores appear to correlate with duration of picking, satisfaction of picking and shame associated with picking.
The third measure is the Skin Picking Impact Scale-Shorter Version (SPIS-S). The SPIS-S is the shorter version of the SPIS consisting of only a 4-question scale (Snorrason et al., 2013). The SPIS and the SPIS-S have a similar factor structure and both have high internal consistency. These measures assess the impacts of picking behaviors on social life, perceived embarrassment associated with picking behaviors, consequences of picking behaviors and perception of attractiveness (Snorrason et al., 2013). The ultimate difference between the two scales is the brevity of the shorter version measure as compared to 10 items on the other measure. Snorrason and associates (2013) found acceptable discriminant and convergent validity for the SPIS and the SPIS-S; both measures may be considered for clinical use.
The Milwaukee Inventory for the Dimensions of Adult Skin Picking (MIDAS) is another skin picking assessment measure (Walther, Flessner, Conelea, & Woods, 2009). The MIDAS consists of 21 items and highlights the degree of focused picking (e.g., body sensations, reaction to negative emotions) and automatic picking behaviors (e.g., unaware of skin picking behaviors, concentrating on another activity, unintentional picking; Walther et al., 2009). Within the measure, each item is rated on a 5-point scale (i.e., 1–5; not true of my skin picking to always true for my skin picking), and a specific score is provided for focused and automatic picking. The MIDAS demonstrates adequate internal consistency and good validity (i.e., construct and discriminant), making it a reliable and valid measure for distinguishing types of skin picking behaviors (Walther et al., 2009). This assessment is especially useful in facilitating an understanding of the client’s motivations for skin picking, as well as potential ways to reduce the problematic behaviors.
The Skin Picking Impact Survey (SKIS; Tucker, Woods, Flessner, Franklin, & Franklin, 2011) is a self-report survey measure. The SKIS, which consists of 92 items, is used to explore multiple dimensions of skin picking behaviors. This survey consists of individual items that assess skin picking symptoms (e.g., presentation), levels of severity (e.g., urges, intensity, time spent, distress, avoidance), consequences (i.e., physical and psychosocial), treatment-seeking history, and demographic information. The SKIS demonstrated acceptable internal consistency (Tucker et al., 2011). Additional items are used to assess for comorbid disorders and other associated symptoms (e.g., depression, anxiety, stress).
Finally, a unique approach to assessing excoriation disorder is to utilize a functional analysis assessment (LaBrot, Dufrene, Ness, & Mitchell, 2014). Although not created primarily to assess skin picking behaviors, a functional analysis assessment is a behavioral technique used to explore the relationship between any stimuli and response (e.g., being cold and shivering; LaBrot et al., 2014). With regards to excoriation disorder, the functional analysis assessment consists of behavior scales and individual interviews with anyone close to the client (e.g., spouse, family member, classroom teacher). The interviews include a discussion of the client’s behaviors and antecedents to such behaviors (LaBrot et al., 2014). This interview also involves a direct observation of the client in the most problematic setting (e.g., home, work, school), and counselors should take note of the time of day or events that often lead up to skin picking behaviors.
A functional analysis assessment also might involve the use of a thought log to help explore thoughts that lead to skin picking behaviors (LaBrot et al., 2014). This connection between thoughts (i.e., obsessions) and behaviors (i.e., compulsions) is characteristic of the obsessive-compulsive DSM-5 classification under which excoriation disorder is housed. Counselors may suggest that clients self-monitor their skin picking behaviors in order to better understand the frequency, triggers, cues, and increases or reductions in thoughts and behaviors. For example, clients may be asked to place a journal or worksheet in places where picking often occurs (e.g., bathroom, bedroom) and then to report and rate the intensity of urges, precipitating events, alternative behaviors, and if picking behaviors actually occurred. When assessing skin picking, clients also should be invited to note any attempts to stop picking, consequences of the skin picking behaviors, and other behaviors that could potentially serve as incompatible replacements (LaBrot et al., 2014). The use of a functional analysis assessment allows the counselor to gain a more complete, contextual picture of the behaviors.
To gain a richer understanding of the client’s behaviors, counselors might (if approved by the client) gather assessment and baseline information from the client’s friends and family members (Grant & Stein, 2014). During the assessment process, counselors should explore all aspects of the client’s life, including recent life experiences, past traumas and current life stressors (LaBrot et al., 2014). An accurate diagnosis and collaborative treatment plan can be developed when this information is integrated to form a contextual understanding of the client’s skin picking experiences.
Diagnosis
A thorough assessment helps counselors to identify an accurate diagnosis. Armed with assessment data, counselors can determine the presence of excoriation disorder and any comorbid disorders. In order to accurately diagnose the disorder, counselors must be familiar with the DSM-5 diagnostic criteria and understand diagnostic considerations related to the disorder.
The onset of excoriation disorder varies significantly, but it most often begins in early adolescence or between the ages of 30 to 45 years old (Grant et al., 2012). Skin picking causes physical harm, and clients often make repeated attempts to reduce the behavior because of the distress and physical impairment it invites. By definition, excoriation disorder is not caused by a substance or medical condition and not accounted for by another disorder (APA, 2013). The diagnostic features of excoriation disorder remain the same regardless of age or other multicultural factors (Grant et al., 2012). The general features that a counselor should look for when diagnosing excoriation disorder include a preoccupation with picking behaviors, difficulty in controlling the behaviors and distress resulting from the behaviors.
Because this is a newer diagnosis, it is often overlooked, misdiagnosed (Grant et al., 2012), or overshadowed by comorbid diagnoses (APA, 2013; Grant & Stein, 2014; Hayes et al., 2009). It is important to distinguish between excoriation disorder and nonsuicidal self-injury, both of which involve self-inflicted damage to the body that provides relief from unwanted thoughts or feelings (APA, 2013). Nonsuicidal self-injury is typically motivated by negative thoughts or feelings about the self in relation to others, and bodily harm provides a feeling of relief or euphoria (APA, 2013; Shapiro, 2008). Conversely, excoriation disorder is an obsessive-compulsive and related disorder and is more ritualistic; unwanted thoughts and feelings are directly related to bumps or certain types of scabs on the body, and clients have a routine related to removal (e.g., examining, picking) and disposal (e.g., playing with or eating) of such bumps or scabs (APA, 2013; Capriotti et al., 2015; Walther et al., 2009).
Individuals with excoriation disorder generally have difficulty resisting the urge to pick and often believe their behavior cannot be altered or changed (Kress & Paylo, 2015). Typically, there are two types of picking behaviors: behaviors that are automatic and behaviors that are focused (Christenson & Mackenzie, 1994). Individuals who engage in picking behavior outside of their awareness, such as while watching television or while reading a book, are engaging in what is known as automatic picking. Those who are fully aware of their behavior and pick to regulate or to manage negative emotions due to specific thoughts or stressors are engaging in focused picking. Both types of picking typically cause client embarrassment, impair functioning and are difficult to manage and control (Odlaug, Chamberlain, & Grant, 2010).
Although focused skin picking might seem to be more directly tied to conscious obsessions than the automatic type, both types were reclassified under obsessive-compulsive and related disorders in the DSM-5 due to the universal obsessive and compulsive features of the disorder; these obsessions and compulsions also are shared with individuals who have trichotillomania (Snorrason, Belleau, & Woods, 2012) and body dysmorphic disorder (Tucker et al., 2011). In each of these disorders, obsessions lead to an overwhelming urge to act upon unhelpful thoughts, which is often followed by a brief sense of relief once the compulsion has been engaged and completed. However, the urge inevitably arises again (despite bodily damage and some potential shame), and the cycle continues.
Ultimately, excoriation disorder is characterized by recurrent and excessive tissue damage that is not better accounted for by nonsuicidal self-injury. Those who have excoriation disorder have difficulty controlling their picking behaviors and experience clinically significant distress or impairment as a result of these behaviors (APA, 2013). Assessment measures can be used in conjunction with the DSM-5 in order to make an accurate diagnosis that can inform clients’ treatment.
Treatment of Excoriation Disorder
Several evidence-based treatment options are available for use in treating those who have excoriation disorder (Kress & Paylo, 2015). Unfortunately, many providers fail to use evidence-based treatment approaches in their work with this population (Tucker et al., 2011). A relatively small number of randomized controlled treatment studies have been conducted on this population; however, the most evidence-based approaches include cognitive behavioral therapy, habit reversal training and pharmacotherapy (Capriotti et al., 2015; Kress & Paylo, 2015).
Cognitive Behavioral Therapy
Cognitive behavioral therapy (CBT) is an effective strategy for working with clients who have excoriation disorder (Grant et al., 2012; Schuck, Keijsers, & Rinck, 2011). Schuck et al. (2011) conducted a randomized study of college-age students who reported pathological skin picking; participants were provided four sessions of CBT and compared to those on a waitlist. These researchers observed a significant decrease in psychosocial impact of skin picking, severity of skin picking and perceived strength of skin picking cognitions in the group randomly assigned to the four sessions of CBT. These treatment effects were maintained at a two-month follow-up, thus suggesting that CBT is effective in reducing the severity of symptoms, effect of symptoms and dysfunctional cognitions associated with excoriation disorder.
Practically, CBT for clients with excoriation disorder is focused on using cognitive restructuring to counter dysfunctional thoughts (Schuck et al., 2011). Before engaging in CBT techniques, a counselor should ascertain the nature and location of the picking and provide clients with psychoeducation regarding the etiological and maintenance factors related to their disorder. Socratic questioning is one CBT technique used to help clients recognize their fundamental beliefs and automatic thoughts surrounding skin picking (Kress & Paylo, 2015). When applying this technique, the counselor generates a hypothesis about the client’s thoughts (that lead to skin picking), but leads the client to the information rather than suggesting it. The client is led to insight through a series of questions regarding the topic of interest. For example, the counselor might believe that a client’s skin picking obsessions become stronger when personal and professional obligations become overwhelming. The counselor might ask, “What feelings do you have when picking? What time of day do you typically pick? Are there ever days when you do not pick?” The counselor would use reflections to organize and expand upon the client’s responses until they gain new insight about their thoughts, patterns and beliefs regarding this behavior.
When using CBT, irrational thoughts are examined for validity and replaced with more rational thoughts and behaviors (Kress & Paylo, 2015). For example, an individual tempted to engage in skin picking after an argument with a spouse would challenge the need to pick with more rational thoughts, such as, “Even if I become anxious, I can tolerate the anxiety. Instead of picking, I can clean the house or exercise.” This type of change occurs over a period of time, and counselors and clients should celebrate small victories, such as delayed or reduced skin picking, as the more rational thoughts begin to become more salient.
CBT not only involves cognitive interventions, but also includes behavioral interventions such as homework, preventative measures, activity replacement and relapse prevention (Capriotti et al., 2015; Kress & Paylo, 2015; Schuck et al., 2011). Counselors may assign homework such as CBT thought logs to help clients track picking behaviors. While reviewing the logs in session, counselors can assist clients in developing preventative measures, such as wearing gloves or bandages to hinder skin picking, and activity replacement such as reading a book, cleaning or watching television instead of skin picking. CBT also places a heavy emphasis on relapse prevention, or the preparation to prevent future urges to pick.
Habit Reversal Training
Habit reversal training (HRT) is an effective strategy for working with clients who have excoriation disorder (Capriotti et al., 2015; Grant et al., 2012; Teng, Woods, & Twohig, 2006). HRT is a behavioral approach that involves helping clients gain awareness of their skin picking and then replace the picking with more adaptive behaviors (Grant et al., 2012; Ravindran et al., 2009; Snorrason & Bjorgvinsson, 2012; Teng et al., 2006). The first step of treatment is awareness training, which helps clients who are often unaware of their skin picking to associate factors, such as time of day and specific situations, to skin picking behavior (Teng et al., 2006). To facilitate this awareness, a counselor may point out in-session skin picking behavior. After developing an awareness of antecedent situations (i.e., the situations that precede picking incidents), the counselor and client collaboratively develop a competing response, or another behavior that is inconsistent with skin picking, to substitute for the skin picking behaviors (Teng et al. 2006). An example of an alternative behavior would be clenching one’s fist each time a client notices that he or she is picking. This competing response, which should be one that is easily applicable in a number of situations, diminishes the urge or reduces its intensity.
The next step in HRT is the establishment of a contingency management system or token economy involving rewards and punishments. This type of treatment approach allows the clients’ behaviors to be rewarded as they make successive approximations toward the goal. Rewards and punishments must be meaningful to clients, and they also must be specific and timely. At first, rewards are extrinsic, such as verbal praise or toys with children. As target behaviors are reached (e.g., reduced skin picking), clients begin to access more intrinsic reinforcers (e.g., an increased sense of self-esteem, feelings of belonging within the community/society). Finally, clients are coached to consistently implement these operant conditioning strategies outside of session and to eventually apply them to new behaviors (Capriotti et al., 2015; Teng et al., 2006). The client gradually realizes that skin picking is not a necessary coping skill, as other, more adaptive behaviors can be used to effectively reduce stress.
Teng et al. (2006) conducted a controlled study in which they compared HRT treatment to a waitlist control group. These researchers demonstrated a significant reduction in skin picking behaviors at the termination of treatment and upon follow-up assessment, as compared to the control group. The research on HRT suggests that it is a promising approach for use with those who have excoriation disorder.
Acceptance and Commitment Therapy
Acceptance and commitment therapy (ACT), when used in conjunction with HRT, demonstrates clinical promise in treating those who have excoriation disorder (Capriotti et al., 2015; Flessner, Busch, Heideman, & Woods, 2008). Capriotti et al. (2015), through the use of multiple clinical case studies, demonstrated that Acceptance-Enhanced Behavioral Therapy (i.e., ACT plus HRT) decreased excoriation symptomology in three of four participants in their case study research. These results support the findings of a similar case study done by Flessner et al. (2008), which demonstrated decreased symptomatology of excoriation disorder when ACT was incorporated with HRT.
ACT uses mindfulness techniques to teach acceptance of negative thoughts and emotions and then combines behavior-change techniques to address unhealthy behaviors (Flessner et al., 2008). Initially, the counselor helps the client investigate previous attempts to curb skin picking behaviors (such as avoidance or relaxation while picking). Then, the client and counselor work to distinguish between urges to pick (i.e., thoughts, feelings, sensations) and actual skin picking, emphasizing that even if urges are acted upon, they will soon return. As such, the focus is on increased distress tolerance and acceptance of urges (Kress & Paylo, 2015). The difficulty of controlling urges can be illustrated through metaphors in which the client gains control and a position of power over an undesirable, yet steadfast external circumstance (e.g., working is unavoidable, but you can find a job you enjoy).
Next, using ACT treatment, the client’s ability to control his or her own thoughts and behaviors is highlighted. This emphasis on controlling behavior stands in contrast to most clients’ natural inclination to focus on controlling or avoiding external situations. Next, the counselor and client work to modify and change the thoughts and feelings associated with urges to pick (Flessner et al., 2008). The client and counselor address six processes that contribute to healthy, flexible living: present-moment awareness; acceptance (as opposed to avoidance); nonjudgmental awareness of one’s thoughts; values clarification; changing, rather than reducing, unhelpful thoughts; and short- and long-term behavioral goals. Lastly, treatment progress is reviewed, and the client and counselor engage in relapse management (Flessner et al., 2008; Twohig, Hayes, & Masuda, 2006). Counselors also can integrate medication management when therapeutically indicated.
Pharmacotherapy
If pharmacotherapy is used to treat excoriation disorder, it should be used in conjunction with counseling; medication can control physical symptoms, but contributing mental health factors must be addressed in order to holistically help the client make enduring behavior changes (Grant et al., 2012). Selective serotonin reuptake inhibitors, specifically Fluoxetine (Prozac), have been shown to be effective in treating excoriation disorder and other BFRB (Grant et al., 2012; Simeon et al., 1997). However, this effect has not been consistent across clients (Grant & Odlaug, 2009). Therefore, additional research on the effectiveness of medication is needed. Counselors should provide intentional treatments for clients while taking into account unique client considerations.
Special Considerations
Although those with excoriation disorder might go to great efforts to conceal their wounds from others (Grant & Odlaug, 2009), they are likely to admit to skin picking behaviors when effectively questioned by a mental health professional (APA, 2013). It is important to ensure the clients with excoriation disorder are physically well (i.e., free from medical complications associated with picking), and a referral to medical professionals to ensure physical safety and appropriate medical care may be necessary (Grant et al., 2012).
Excoriation disorder occurs more often in those who also have OCD (APA, 2013). Several additional disorders are often found to be comorbid with excoriation disorder, including trichotillomania, major depressive disorder, anxiety, psychotic disorders, neurodevelopmental disorders and factitious disorder (APA, 2013; Hayes et al., 2009). Picking behaviors also could be due to a general medical condition or substance use (e.g., such as with methamphetamine addictions), and these should be ruled out (APA, 2013).
Those with first-degree family members who have excoriation disorder are more likely to also develop skin picking behaviors (APA, 2013). However, skin picking often begins during puberty, and the onset is often associated with the development of skin irregularities (e.g., acne; APA, 2013, Tucker et al., 2011). It was found that approximately 87% of college-aged students in Turkey who had acne or other skin blemishes displayed some skin picking behaviors, but only about 2% reported clinically-significant symptoms of excoriation disorder (Calikusu, Kucukgoncu, Tecer, & Bestepe, 2012). The aforementioned finding suggests that dermatological factors, such as acne, might explain the onset of excoriation disorder, but not necessarily the maintenance of such behaviors. As such, family history of such behaviors should be considered by counselors when assessing and treating this disorder.
Collaborative relationships with other professionals can be helpful when working with a client who has excoriation disorder. If clients are provided with psychopharmaceutical interventions, counselors should take care to communicate with the prescribing physician in order to help the client maintain proper medication schedules and to potentially provide psychoeducational support to the client (Grant et al., 2012). Although consultation with a dermatologist is not always necessary, this valuable resource should be integrated into treatment when possible, and open communication can ensure that clients are receiving the support that they need (Calikusu et al., 2012; Grant et al., 2012).
Finally, although excoriation disorder is now an official DSM diagnosis, the research literature on effective treatments is still in its infancy stage (Capriotti et al., 2015). Additional research also is needed to determine the prognosis of excoriation disorder. As previously indicated, researchers have found psychopharmaceutical and cognitive behavioral interventions to be promising (Flessner et al., 2008; Grant et al., 2012; Schuck, et al., 2011; Simeon et al., 1997), but additional outcome research still needs to be conducted on this disorder (Capriotti et al., 2015). Further research on this new DSM-5 disorder will provide more concrete information regarding assessment and treatment options for this population.
Summary
The etiology of excoriation disorder is still being explored, and several theories are currently supported as viable options. Both biological and psychological factors appear to contribute to the development and maintenance of this disorder (Grant et al., 2012). Skin picking behaviors are often found in those who have higher levels of emotional impulsivity, and these behaviors might serve as a way for individuals to regulate their emotions.
There are several formal measures that can be used to aid in the assessment and diagnosis of excoriation disorder. In addition to formal quantitative measures, the functional analysis assessment is a helpful method that can be used to increase both the client’s and the counselor’s understanding of the behaviors (LaBrot et al., 2014). Regardless of the assessment procedures employed, counselors should explore all aspects of the client’s life in order to create a comprehensive treatment approach.
Since excoriation disorder is a new diagnosis in the DSM-5, it is often overlooked or misdiagnosed. Counselors should fully assess a client’s presenting concerns in order to determine an accurate and helpful diagnosis. Counselors also should note that this disorder is often comorbid with other mental disorders (APA, 2013; Grant et al., 2011; Hayes et al., 2009).
In terms of the treatment of excoriation disorder, CBT is one of the more evidence-based approaches (Grant et al., 2012; Schuck et al., 2011), as is HRT (Grant et al., 2012; Teng et al., 2006). ACT has been used with success with HRT (Capriotti et al., 2015; Flessner et al., 2008). Psychopharmacotherapy also holds promise as an effective adjunct to psychosocial treatments (Grant et al., 2012; Simeon et al., 1997).
Conflict of Interest and Funding Disclosure
The authors reported no conflict of interest
or funding contributions for the development
of this manuscript.
References
American Psychiatric Association. (2013). Diagnostic and statistical manual of mental disorders (5th ed.). Washington, DC: Author.
Calikusu, C., Kucukgoncu, S., Tecer, Ö., & Bestepe, E. (2012). Skin picking in Turkish students: Prevalence, characteristics, and gender differences. Behavior Modification, 36, 49–66. doi:10.1177/0145445511420282
Capriotti, M. R., Ely, L. J., Snorrason, I., & Woods, D. W. (2015). Acceptance-enhanced behavior therapy for excoriation (skin-picking) disorder in adults: A clinical case series. Cognitive and Behavioral Practice, 22(2), 230-239. doi:10.1016/j.cbpra.2014.01.008
Christenson, G. A., & Mackenzie, T. B. (1994). Trichotillomania. In M. Hersen & R. T. Ammerman (Eds.), Handbook of prescriptive treatment for adults (pp. 217–235). New York, NY: Plenum.
Dufour, B. D., Adeola, O., Cheng, H. W., Donkin, S. S., Klein, J. D., Pajor, E. A., & Garner, J. P. (2010). Nutritional up-regulation of serotonin paradoxically induces compulsive behavior. Nutritional Neuroscience, 13,
256–264. doi:10.1179/147683010X12611460764688
Flessner, C. A., Berman, N. C., Garcia, A. M., Freeman, J. B., & Leonard, H. L. (2009). Symptom profiles on pediatric obsessive compulsive disorder: The effects of comorbid grooming conditions. Journal of Anxiety Disorders, 23, 753–759. doi:10.1016/j.janxdis.2009.02.018
Flessner, C. A., Busch, A. M., Heideman, P. W., & Woods, D. W. (2008). Acceptance-enhanced behavior therapy (AEBT) for trichotillomania and chronic skin picking: Exploring the effects of component sequencing. Behavior Modification, 32, 579–594. doi:10.1177/0145445507313800
Grant, J. E., & Odlaug, B. L. (2009). Update on pathological skin picking. Current Psychiatry Reports, 11, 283–288.
Grant, J. E., Odlaug, B. L., & Chamberlain, S. R. (2011). A cognitive comparison of pathological skin picking and trichotillomania. Journal of Psychiatric Research, 45, 1634–1638. doi:10.1016/j.jpsychires.2011.07.012
Grant, J. E., Odlaug, B. L., Chamberlain, S. R., Keuthen, N. J., Lochner, C., & Stein, D. J. (2012). Skin picking disorder. American Journal of Psychiatry, 169, 1143–1149. doi:10.1176/appi.ajp.2012.12040508
Grant, J. E., & Stein, D. J. (2014). Body-focused repetitive disorders in ICD-11. Revista Brasileira de Psiquiatria, 36, S59–S64. doi:10.1590/1516-4446-2013-1228
Hayes, S. L., Storch, E. A., & Berlanga, L. (2009). Skin picking behaviors: An examination of the prevalence and severity in a community sample. Journal of Anxiety Disorders, 23, 314–319. doi:10.1016/j.janxdis.2009.01.008
Keuthen, N. J., Deckersbach, T., Wilhelm, S., Engelhard, I., Forker, A., O’Sullivan, R. L., . . . & Baer, L. (2001a). The Skin Picking Impact Scale (SPIS): Scale development and psychometric analyses. Psychosomatics, 42, 397–403.
Keuthen, N. J., Sabine, W., Deckersbach, T., Engelhard, I. M., Forker, A. E., Baer, L., & Jenike, M. A. (2001b). The Skin Picking Scale: Scale construction and psychometric analyses. Journal of Psychosomatic Research, 50, 337–341.
Kress, V. E, & Paylo, M. J. (2015). Treating those with mental disorders: A comprehensive approach to case conceptualization and treatment. Upper Saddle River, NJ: Pearson.
LaBrot, L., Dufrene, B. A., Ness, E., & Mitchell, R. (2014). Functional assessment and treatment of trichotillomania and skin-picking: A case study. Journal of Obsessive-Compulsive and Related Disorders, 3, 257–264. doi:10.1016/j.jocrd.2014.06.006
Lang, R., Didden, R., Machalicek, W., Rispoli, M., Sigafoos, J., Lancioni, G., . . . & Kang, S. (2010). Behavioral treatment of chronic skin-picking in individuals with developmental disabilities: A systematic review. Research in Developmental Disabilities, 31, 304–315. doi:10.1016/j.ridd.2009.10.017
Neziroglu, F., Rabinowitz, D., Breytman, A., & Jacofsky, M. (2008). Skin picking phenomenology and severity comparison. Primary Care Companion – Journal of Clinical Psychiatry, 10, 306–312.
Odlaug, B. L., Chamberlain, S. R., & Grant, J. E. (2010). Motor inhibition and cognitive flexibility in pathologic skin picking. Progress in Neuro-Psychopharmacology & Biological Psychiatry, 34, 208–211.
doi:10.1016/j.pnpbp.2009.11.008
Odlaug, B. L., & Grant, J, E. (2010). Pathologic skin picking. The American Journal of Drug and Alcohol Abuse, 36, 296–303. doi:10.3109/00952991003747543
Ravindran, A. V., da Silva, T. L., Ravindran, L. N., Richter, M. A., & Rector, N. A. (2009). Obsessive-compulsive spectrum disorders: A review of the evidence-based treatments. The Canadian Journal of Psychiatry, 54, 331–343.
Schuck, K., Keijsers, G. P., & Rinck, M. (2011). The effects of brief cognitive-behaviour therapy for pathological skin picking: A randomized comparison to wait-list control. Behaviour Research and Therapy, 49, 11–17. doi:10.1016/j.brat.2010.09.005
Shapiro, S. (2008). Addressing self-injury in the school setting. The Journal of School Nursing, 24(3), 124–130. doi:10.1177/1059840512344321
Simeon, D., Stein, D. J., Gross, S., Islam, N., Schmeidler, J., & Hollander, E. (1997). A double-blind trial of fluoxetine in pathological skin picking. Journal of Clinical Psychiatry, 58, 341–347.
Snorrason, Í., Belleau, E. L., & Woods, D. W. (2012). How related are hair pulling disorder (trichotillomania) and skin picking disorder? A review of evidence for comorbidity, similarities and shared etiology. Clinical Psychology Review, 32, 618–629. doi:10.1016/j.cpr.2012.05.008
Snorrason, Í., & Bjorgvinsson, T. (2012). Diagnosis and treatment of hair pulling and skin picking disorders. Laeknabladid, 98, 155–162.
Snorrason, Í., Ólafsson, R. P., Flessner, C. A., Keuthen, N. J., Franklin, M. E., & Woods, D. W. (2013). The Skin Picking Impact Scale: Factor structure, validity and development of a short version. Scandinavian Journal of Psychology, 54, 344–348. doi:0.1111/sjop.12057
Snorrason, Í., Smári, J., & Ólafsson, R. P. (2011). Motor inhibition, reflection impulsivity, and trait impulsivity in pathological skin picking. Behavior Therapy, 42, 521–532. doi:10.1016/j.beth.2010.12.002
Teng, E. J., Woods, D. W., & Twohig, M. P. (2006). Habit reversal as a treatment for chronic skin picking: A pilot investigation. Behavior Modification, 30, 411–422.
Tucker, B. T., Woods, D. W., Flessner, C. A., Franklin, S. A., & Franklin, M. E. (2011). The skin picking impact project: Phenomenology, interference, and treatment utilization of pathological skin picking in a population-based sample. Journal of Anxiety Disorders, 25, 88–95. doi:10.1016/j.janxdis.2010.08.007
Twohig, M. P., Hayes, S. C., & Masuda, A. (2006). A preliminary investigation of acceptance and commitment therapy as a treatment for chronic skin picking. Behaviour Research and Therapy, 44, 1513–1522. doi:10.1016/j.brat.2005.10.002
Walther, M. R., Flessner, C. A., Conelea, C. A., & Woods, D. W. (2009). The Milwaukee Inventory for the Dimensions of Adult Skin Picking (MIDAS): Initial development and psychometric properties. Journal of Behavior Therapy and Experimental Psychiatry, 40, 127–135. doi:10.1016/j.jbtep.2008.07.002
Nicole A. Stargell, NCC, is an Assistant Professor at the University of North Carolina at Pembroke. Victoria E. Kress, NCC, is a Professor at Youngstown State University. Matthew J. Paylo is an Associate Professor at Youngstown State University. Alison Zins is a graduate student at Youngstown State University. Correspondence can be addressed to Nicole Stargell, UNC Pembroke, P.O. Box 1510, Department of Educational Leadership and Counseling, 341 Education Building, Pembroke, NC 28372, nicole.stargell@uncp.edu.
Sep 6, 2014 | Article, Volume 1 - Issue 1
James P. Sampson, Jr., Robert C. Reardon
Fundamental changes occurring in the nature of work have led some authors to contest that established approaches to delivering career services may no longer be efficacious. This article challenges such notions and examines the idea of changing occupations and how these changes may influence the delivery of career services. While important changes have occurred, occupations remain a viable unit of analysis for the assessment and information resources used in delivering career services. The article concludes with clinical implications for career counselors and service providers.
Keywords: career services, occupations, assessment, career theory, technological innovations, clinical implications
While the efficacy of various educational and vocational guidance interventions has always been a matter of debate, concerns have been raised about the continued use of interventions developed in the past and based on possibly outdated concepts (e.g., occupation). An example of such a concern was raised most recently by Savickas et al. (2009).
We reviewed the literature on this topic and constructed a generalized assertion compiled from various sources:
The transition from the industrial age to the information age has been accompanied by
unprecedented change. Virtually every aspect of modern life has been impacted by
technology. Occupations have changed in fundamental ways as technology and globalization
have reshaped the workplace. Occupations have become fluid and organizations are evolving
rapidly, adapting their workforce to respond to a rapidly evolving marketplace.
Although the wording of this concern changes from one talk or publication to another, the essential elements are often repeated in the media and mentioned in presentations at professional meetings. This assertion has been repeated so often that it has attained the status of fact. The only problem is that it is not true. An analysis of current labor market information indicates that the extent of change in occupations, while real and important, is not as pervasive as common knowledge would have us believe.
A second assertion follows from the one above:
Much of the current practice in educational and vocational guidance is the product of the
industrial age. Old models of practice, based on ideas about occupations and work that have
changed dramatically, need to change to reflect the demands of the information age.
These assertions, although popular, are flawed for two reasons. First, the extent of change in occupations is not as great as commonly assumed. Second, even if substantive changes have occurred, we have no data showing that well designed and implemented career interventions created in the past are no longer effective (Brown et al., 2003).
This paper examines the idea of changing occupations and how these changes may have affected the delivery of career services. For the purposes of this paper, occupations are defined as “a group of similar positions found in different industries or professions” (Reardon, Lenz, Sampson, & Peterson, 2009, p. 7).
Misperceptions of the Extent of Change in Occupations
The perception that unprecedented change has occurred in occupations is the result of a variety of factors, including: (a) the idea that the magnitude of change between the agricultural and the industrial age was less than the change between the industrial age to the information age, (b) inaccurate information in the public media about change in occupations, and (c) the failure to use career theory in analyzing occupational change.
Change Across the Agricultural, Industrial, and Information Ages
Technology changes over time have profoundly influenced the lives of individuals, organizations and governments. For example, the steam engine and electricity changed the nature of work from the agricultural age to the industrial age, and the computer has led to the current information age. Some consider the extent of change between the agricultural age and the industrial age as less than or equivalent to the extent of change between the industrial age and the information age. In a discussion of the information age, Watts (1999, p.1) noted, “Robert Reich has called it the ‘second great crossing,’ comparable to the move from the land to the factory.”
While this assertion may be true in some respects, the magnitude of these changes is not equivalent in our opinion. First, there was a massive geographic displacement from rural to urban areas in the transition from the agricultural to the industrial age. While relocation of workers still occurs at the present time, it is not on the scale that it was a century ago. Individuals moving from manufacturing to service occupations are often able to obtain employment without physically relocating. Moreover, in many instances it is factories that are moving to locations where labor is cheap. Second, 100 years ago success as a farmer was dependent on having a wide variety of skills. For example, the farmer did the work because it was too expensive to hire someone else to repair machinery. When farmers moved to urban areas and began working in factories, the range of job skills needed declined substantially. Work was simplified and made routine in order to improve efficiency in the factory. While this was not true for all workers, it did create a clear demarcation in the nature of working between the agricultural age and the industrial age.
More recently, Friedman (2005) suggested that technology innovations and the global economy now make it possible for individuals to work more independently in a flattened world. The work is accomplished in real time without regard to distance or worker location. While new forms of business organizations and ways of working will lead to occupational changes in the information age, the most important difference today from the past may be simply the rate of change.
As aforementioned, we do not see the changes in occupations and work occurring between the industrial age and the information age as having been as dramatic as the changes between the agricultural age and the industrial age. While information technology has increased the speed of change and the increasing complexity of work tasks has required more collaboration among workers, we believe the amount of change in occupations in our contemporary world has been oversold.
In all three eras, there were and have been changes in gender roles and relationships, family life, lifestyles, financial income, the kinds of jobs available, ways of working, job training and the diverse characteristics of workers (e.g., ethnicity, disability, and the nature of occupational choices). But, there are still jobs in construction, business and social services, food production, manufacturing, transportation, education, and a host of other industries, and these jobs comprise the occupations that persist in the new age.
Public Media Information about Change in Occupations
The perception that occupations are undergoing substantive change has been exacerbated by inaccurate information about occupations presented in mass media. The fact that the demand for home health aids, accountants, receptionists and food service workers is growing at 5% is not particularly newsworthy. However, the fact that the demand for robotic technicians is increasing at 50% per year is newsworthy, especially when the story is accompanied by video of a robot performing simple household tasks while the homeowner watches from a corner of the room and comments on how nice it is to have a robot. A brief interview with the robotic technician, stating how exciting and rewarding their job is, reinforces the notion that robotics work is a good option for the future. However, reality presents a different picture.
The concept of big growth and fast growth occupations (Reardon et al., 2009) is relevant here. For example, projected employment growth for environmental engineers and accountants/auditors from 2002 to 2012 shows 18,000 for the former and 205,000 for the latter. But, when the percent of employment growth is examined for these two occupations, the rate for environmental engineers is 38% (fast growth) and accountants/auditors are 19% (big growth with 205,000 jobs projected). The distinction between big growth occupations and fast growth occupations is rarely mentioned in the media. The public, as well as educational and vocational guidance practitioners who have limited knowledge of labor market information, easily conclude that substantive changes in occupations are occurring when only percent change is examined. Indeed, occupations with the most openings are not new, different, or unique but familiar and common (Reardon et al., 2009).
Pikulinski (2004), an economist with the U.S. Department of Labor, reported that most new and emerging occupations are in firms with fewer than 100 employees. Even many of the fast growth occupations in the U.S. are in familiar areas of work. For example, 11 of the 20 fastest-growing occupations are in the fields of health services or the provision of social, personal, or mental health services (Reardon et al., 2009).
Using Career Theory to Understand Occupational Change
Occupational change can be examined from the standpoint of Holland’s (1997) career theory and provides a familiar schema for counselors in examining occupational change. U.S. census data from 1960 to 2000 provides evidence about the extent of change in occupations relative to Holland codes. First, occupational titles included in the census have remained quite constant over time, which is an indication of stability in occupational schema. Second, the pattern of employment for men and women by Holland code (realistic, investigative, artistic, social, enterprising and conventional) has been relatively stable (Reardon, Bullock, & Meyer, 2007). Third, realistic jobs have held constant from 1960 to 2000 and employed the most people; however, the percentage of people working in the realistic area has been declining. Very few people work in the Artistic area (about 1–2%) and this has remained constant over five decades, but occupational employment in the enterprising area has been increasing slightly over the same period.
The application of a career theory developed over the past 40-plus years adds to our understanding of occupations and occupational change, and it should be a basic tool for career counselors. However, this is not often noted in much contemporary career literature forecasting the demise of work as we have known and understood it. We believe that Holland’s (1997) matching model is supported by data and experience related to occupational employment and can inform career services.
Actual Changes in Occupations
It is obvious that some change has occurred in occupations. For example, we would suggest that most occupations have been impacted by information technology ranging from bar codes, cell phones, computers, the Internet, social media and more. However, other aspects of work have not changed. Essential work behaviors such as problem-solving, written and oral communication, interpersonal relationship skills, manual dexterity, and creativity have remained constant despite rapid changes in technology. Moreover, job vacancy notices are still posted announcing the availability of work, and job titles are used as a quick way to communicate information about the nature of the work. Internet job boards such as CareerBuilder and Monster list millions of positions daily, and these positions have job titles for specific employing organizations that can be generalized to occupational titles across fields of work.
Consider the following examples: In dentistry, technology has led to improved instruments, electronic databases are used to store patient records, and X-rays are now viewed and stored digitally. However, other aspects of work have not changed. Essential work behaviors such as assessment, diagnosis, treatment planning, communication with patients, manual dexterity, and selecting and managing staff remain essential to the success of a dentist. The essential work behaviors of a dentist have not changed in 100 years.
Carpenters are another example. Despite advances in materials and methods of home construction, carpenters are still employed in large numbers. Although the use of prefabricated building materials has reduced the need for some specialized craft skills, such as making crown molding, the essential work behaviors of problem-solving, eye-hand coordination, teamwork and planning have remained constant.
The number of individuals employed in various occupations increases and decreases with changes in the economy. This dynamic was as much a feature of the industrial age as it is in the information age. The loss of positions for the coopers who shaped wooden staves and assembled barrels occurred in the industrial age long before computers became commonplace. Web designers are often given as an example of the substantive change currently occurring in occupations. Forklift drivers were unknown in 1870, but were commonplace by 1950 during the industrial age. The pace of change in the information age is undoubtedly faster, but it is a mistake to confuse the rate of change in occupations with the extent of change. A relatively small number of occupations appear and disappear in the labor market each decade, but the characteristics of most occupations change only incrementally and these changes are often peripheral (as is the case with dentists).
Occupational credentialing provides additional evidence of the relative stability of occupations. Despite changes in work tasks, numerous occupations still require a license or certification to work independently. There is no evidence that the number of occupations requiring a credential has decreased. Certainly the knowledge and skills required for credentialed workers evolves over time. These changes are reflected in content modifications in licensure and certification exams, as well as changes in experience requirements required for credentialing. However, the core elements of credentialed occupations are stable enough to warrant continued certification.
The process of identifying and describing an occupation is the work of occupational analysts who use a variety of specialized tools and classification systems in their work. For example, analysts working with census data examine hundreds of thousands of jobs and employment situations reported by citizens in each census period. Researchers then categorize the detailed job information into occupational groups using the census occupational codes and more recently the Standard Occupation Code (SOC; U.S. Dept. of Labor, 2000) to classify occupations. SOC is the system now used with O*NET, the online, comprehensive listing of the most common occupations in the U.S. that employ the most persons.
Implications for Practice in Career Services
We believe the magnitude of change in occupations has been oversold in professional counseling literature and in the popular media. The transition to the information age has not had the substantive impact on occupations that is generally believed. Thomas Gutteridge and Raymond Palmer, a researcher and career counselor, respectively, suggested that it is jobs that are changing, not occupations (as cited in Patterson & Allen, 1996). They noted that it is a mistake to consider the occupational world as unstable or unpredictable because the vast majority of occupations change very little. The findings of Reardon, Bullock, and Meyer (2007) support their assertion. The career assessments and career information used in the provision of educational and vocational guidance services are based on occupations and not jobs, and practitioners should have confidence that this is a useful schema for career services.
While a few occupations will change more, most will change less. Labor market analysts have the expertise to maintain the validity of occupational data. We also have the technology required to maintain and quickly disseminate these data. However, without a public policy to provide adequate and stable funding for analysis and dissemination of occupational information, the opportunity to provide individuals with potentially helpful career information will be negatively impacted.
While important changes in work have occurred, occupations remain a viable unit of analysis for the assessment and information resources used in delivering career services. It is inappropriate to assume that current changes occurring in the nature of work are a sufficient justification for substantive change in the delivery of career services. Career interventions that are old are not out of date unless there is evidence that some other intervention is more effective. Changes in the delivery of career services should be based on evidence that changes are warranted and that other interventions are likely to be more effective. New ideas are not necessarily better and old ones are not necessarily worse. As Savickas et al. (2009, p. 240) stated, “…we must not lose sight of those valuable contributions of 20th century theories and techniques that remain relevant in this new era. As we go forward, we should manage the great inheritance of the last decades of the 20th century, while increasing its richness.”
References
Brown, S. D., Krane, N. E. R., Brecheisen, J. Castelino, P. Budisin, I., Miller, M., & Edens, L. (2003). Critical ingredients of career choice interventions: More analyses and new hypotheses. Journal of Vocational Behavior, 62, 411–428.
Friedman, T. L. (2005). The world is flat. New York, NY: Farrar, Straus, & Giroux.
Holland, J. L. (1997). Making vocational choices. Odessa, FL: Psychological Assessment Resources.
Patterson, V., & Allen, C. (1996). Occupational outlook overview: Where will the jobs be in 2005? Journal of Career Planning & Employment, 56(3), 32–35, 61–64.
Pikulinski, J. (2004). New and emerging occupations. Monthly Labor Review, 127(12), 39–42.
Reardon, R. C., Bullock, E. E., & Myer, K. E. (2007). A Holland perspective on the U.S. workforce from 1960 to 2000. The Career Development Quarterly, 55, 262–274.
Reardon, R., Lenz, J., Sampson, J., & Peterson, G. (2009). Career development and planning: A comprehensive approach (3rd. ed.). Mason, OH: Cengage Learning.
Savickas, M. L., Nota, L., Rossier, J., Dauwalder, J. P., Duarte, M. E., Guichard, J., Soresi, S., Van Esbroek, R., & van Vianin, A. M. E. (2009). Life designing: A paradigm for career construction in the 21st century. The Journal of Vocational Behavior, 75, 239–250.
U.S. Department of Labor. (2000). Standard occupational classification (SOC) system manual. Washington, DC: U.S. Government Printing Office.
Watts, A.G. (1999). Reshaping Career Development for the 21st Century. Inaugural Professorial Lecture. Derby: Centre for Guidance Studies, University of Derby.
James P. Sampson, Jr., NCC, is the Mode L. Stone Distinguished Professor of Counseling and Career Development, Associate Dean of the College of Education, and Co-Director of the Center for the Study of Technology in Counseling and Career Development, while Robert C. Reardon, NCC, is Professor Emeritus and Senior Research Associate in the Center for the Study of Technology in Counseling and Career Development, both at Florida State University. This article was originally presented at a symposium in Padova, Italy, sponsored by the International Association of Educational and Vocational Guidance, the Society for Vocational Psychology, and the National Career Development Association, September 3, 2007. Appreciation is expressed to Ashley Chason, Casey Dozier, and Stephanie Rodriguez for their assistance with the literature review. Correspondence should be directed to James P. Sampson, Jr., Florida State University, 307 Stone Building, Tallahassee, FL 32306-4453, jsampson@fsu.edu.